igormq / ctc_tensorflow_example
时间: 2024-01-14 10:00:43 浏览: 69
igormq / ctc_tensorflow_example 是一个基于TensorFlow的项目,用于演示如何使用TensorFlow来实现连接时间分类(CTC)算法。
连接时间分类算法是一种用于处理序列数据的算法,常用于语音识别和自然语言处理等领域。这个项目提供了一个示例,演示了如何使用TensorFlow来构建一个简单的CTC模型,并使用该模型对序列数据进行分类。
在这个项目中,igormq提供了一些基本的代码结构和示例数据,让用户可以快速上手。通过阅读代码并按照示例进行实验,用户可以学习如何使用TensorFlow来构建和训练CTC模型,以及如何对序列数据进行预测和分类。
这个项目的代码结构清晰,注释详细,适合初学者学习。通过阅读和实践,用户可以加深对CTC算法和TensorFlow框架的理解,为进一步深入学习相关领域打下坚实的基础。
总的来说,igormq / ctc_tensorflow_example 是一个非常有价值的项目,对于想要学习TensorFlow和CTC算法的人来说是一个很好的学习资源。感谢igormq的贡献,希望这个项目能够帮助更多的人更快地掌握这些知识。
相关问题
请将下列shell语句转换为python语句:if [ ${stage} -le 2 ] && [ ${stop_stage} -ge 2 ]; then # Make train dict echo "Make a dictionary" mkdir -p $(dirname $dict) echo "<blank> 0" > ${dict} # 0 will be used for "blank" in CTC echo "<unk> 1" >> ${dict} # <unk> must be 1 if [ "${token_type}" = bpe ]; then # we borrowed these code and scripts which are related bpe from ESPnet. cut -f 2- -d" " data/${train_set}/text | sort > data/lang_char/input.txt tools/spm_train --input=data/lang_char/input.txt --vocab_size=${nbpe} --model_type=${bpemode} --model_prefix=${bpemodel} --input_sentence_size=100000000 tools/spm_encode --model=${bpemodel}.model --output_format=piece < data/lang_char/input.txt | tr ' ' '\n' | sort | uniq | awk '{print $0 " " NR+1}' >> ${dict} elif [ "${token_type}" = char ]; then tools/text2token.py -s 1 -n 1 data/${train_set}/text | cut -f 2- -d" " | tr " " "\n" \ | sort | uniq | grep -a -v -e '^\s*$' | awk '{print $0 " " NR+1}' >> ${dict} fi num_token=$(cat $dict | wc -l) echo "<sos/eos> $num_token" >> $dict # <eos> fi
以下是将上述shell语句转换为Python语句的代码:
```python
if stage <= 2 and stop_stage >= 2:
# Make train dict
print("Make a dictionary")
os.makedirs(os.path.dirname(dict), exist_ok=True)
with open(dict, "w") as f:
f.write("<blank> 0\n") # 0 will be used for "blank" in CTC
f.write("<unk> 1\n") # <unk> must be 1
if token_type == "bpe":
# we borrowed these code and scripts which are related bpe from ESPnet.
with open(f"data/{train_set}/text") as text_file:
texts = [line.strip().split()[1:] for line in text_file]
with open("data/lang_char/input.txt", "w") as input_file:
for text in texts:
input_file.write(" ".join(text) + "\n")
subprocess.run(f"tools/spm_train --input=data/lang_char/input.txt --vocab_size={nbpe} --model_type={bpemode} --model_prefix={bpemodel} --input_sentence_size=100000000", shell=True)
with open(f"{bpemodel}.vocab") as vocab_file:
vocab = [line.strip().split()[0] for line in vocab_file]
with open(dict, "a") as dict_file:
for i, vocab_item in enumerate(vocab):
dict_file.write(f"{vocab_item} {i+2}\n")
elif token_type == "char":
with open(f"data/{train_set}/text") as text_file:
texts = [line.strip().split()[1] for line in text_file]
texts = " ".join(texts)
tokens = subprocess.check_output(f"echo '{texts}' | tools/spm_encode --model={bpemodel}.model --output_format=piece", shell=True)
tokens = tokens.decode().split("\n")
tokens = [token.strip() for token in tokens if token.strip() != ""]
tokens = sorted(set(tokens))
with open(dict, "a") as dict_file:
for i, token in enumerate(tokens):
dict_file.write(f"{token} {i+2}\n")
num_token = sum(1 for line in open(dict))
with open(dict, "a") as dict_file:
dict_file.write(f"<sos/eos> {num_token}\n") # <eos>
```
需要注意的是,在Python中也需要使用相应的模块和工具来实现shell语句中的一些操作,例如在此代码中使用了`os`、`subprocess`模块和`tools/spm_train`、`tools/spm_encode`脚本来进行文件操作和调用外部命令。另外,由于Python中没有直接对应的`$`符号,需要使用`f-string`或者`str.format()`方法来进行字符串格式化。
描述 资源 路径 位置 类型 ctc E299: dereferencing a non-pointer LQ_CCU6.c /LQ_TC264DA_LIB/src/Driver 第 649 行 C/C++ 问题
根据您提供的信息,您在LQ_CCU6.c文件的/LQ_TC264DA_LIB/src/Driver路径的第649行遇到了一个C/C++问题,即E299: dereferencing a non-pointer。这个错误通常发生在您尝试对非指针类型的变量进行解引用操作时。解引用操作只能在指针类型的变量上使用。
要解决此问题,您需要检查LQ_CCU6.c文件中第649行的代码,并确保您正在对一个指针类型的变量进行解引用操作。如果您确定该变量是指针类型的,那么可能是在声明或使用该变量时出现了其他错误。您可以仔细检查变量的声明和使用情况,以确保正确使用指针。
如果您需要进一步的帮助,请提供相关代码的片段,以便我们能够更好地理解问题并为您提供更准确的解决方案。
阅读全文
相关推荐
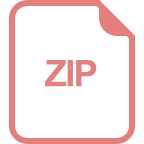
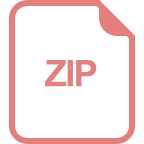
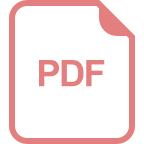
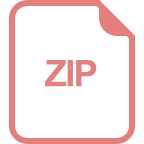
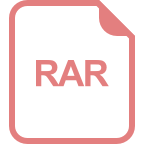
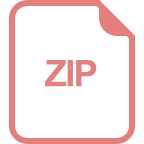
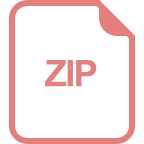
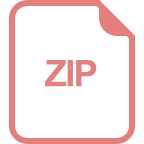
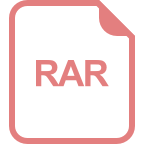
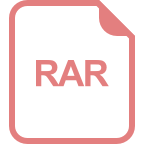
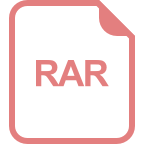
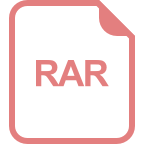


