def vector(data): h,w = data.shape[0:2] img_pixel = np.asarray(data).reshape(1,h*w) return img_pixel
时间: 2024-06-07 22:07:40 浏览: 15
这是一个 Python 函数,用于将二维数组转化为一维数组。具体来说,它接受一个形状为 (h, w) 的二维数组 data,将其转化为形状为 (1, h*w) 的一维数组 img_pixel,并返回该一维数组。其中,h 和 w 分别代表二维数组的行数和列数,np.asarray() 则将输入数据转化为 ndarray 类型的数组。
相关问题
程序执行提示AttributeError: 'point_cloud_generator' object has no attribute 'widthself',优化程序class point_cloud_generator(): def __init__(self, rgb_file, depth_file, save_ply, camera_intrinsics=[784.0, 779.0, 649.0, 405.0]): self.rgb_file = rgb_file self.depth_file = depth_file self.save_ply = save_ply self.rgb = cv2.imread(rgb_file) self.depth = cv2.imread(self.depth_file, -1) print("your depth image shape is:", self.depth.shape) self.width = self.rgb.shape[1] self.height = self.rgb.shape[0] self.camera_intrinsics = camera_intrinsics self.depth_scale = 1000 def compute(self): t1 = time.time() depth = np.asarray(self.depth, dtype=np.uint16).T # depth[depth==65535]=0 self.Z = depth / self.depth_scale fx, fy, cx, cy = self.camera_intrinsics X = np.zeros((self.width, self.height)) Y = np.zeros((self.width, self.height)) for i in range(self.width): X[i, :] = np.full(X.shape[1], i) self.X = ((X - cx / 2) * self.Z) / fx for i in range(self.height): Y[:, i] = np.full(Y.shape[0], i) self.Y = ((Y - cy / 2) * self.Z) / fy data_ply = np.zeros((6, self.width * self.height)) data_ply[0] = self.X.T.reshape(-1)[:self.widthself.height] data_ply[1] = -self.Y.T.reshape(-1)[:self.widthself.height] data_ply[2] = -self.Z.T.reshape(-1)[:self.widthself.height] img = np.array(self.rgb, dtype=np.uint8) data_ply[3] = img[:, :, 0:1].reshape(-1)[:self.widthself.height] data_ply[4] = img[:, :, 1:2].reshape(-1)[:self.widthself.height] data_ply[5] = img[:, :, 2:3].reshape(-1)[:self.widthself.height] self.data_ply = data_ply t2 = time.time() print('calcualte 3d point cloud Done.', t2 - t1)
在代码中有两处出现了 `self.widthself.height`,这应该是想要写成 `self.width * self.height`。你需要修改这两处,如下所示:
```
data_ply[0] = self.X.T.reshape(-1)[:self.width * self.height]
data_ply[1] = -self.Y.T.reshape(-1)[:self.width * self.height]
data_ply[2] = -self.Z.T.reshape(-1)[:self.width * self.height]
img = np.array(self.rgb, dtype=np.uint8)
data_ply[3] = img[:, :, 0:1].reshape(-1)[:self.width * self.height]
data_ply[4] = img[:, :, 1:2].reshape(-1)[:self.width * self.height]
data_ply[5] = img[:, :, 2:3].reshape(-1)[:self.width * self.height]
```
这样就可以避免出现 `AttributeError: 'point_cloud_generator' object has no attribute 'widthself'` 的错误了。
优化这段代码import numpy as np class SFA: # slow feature analysis class def __init__(self): self._Z = [] self._B = [] self._eigenVector = [] def getB(self, data): self._B = np.matrix(data.T.dot(data)) / (data.shape[0] - 1) def getZ(self, data): derivativeData = self.makeDiff(data) self._Z = np.matrix(derivativeData.T.dot(derivativeData)) / (derivativeData.shape[0] - 1) def makeDiff(self, data): diffData = np.mat(np.zeros((data.shape[0], data.shape[1]))) for i in range(data.shape[1] - 1): diffData[:, i] = data[:, i] - data[:, i + 1] diffData[:, -1] = data[:, -1] - data[:, 0] return np.mat(diffData) def fit_transform(self, data, threshold=1e-7, conponents=-1): if conponents == -1: conponents = data.shape[0] self.getB(data) U, s, V = np.linalg.svd(self._B) count = len(s) for i in range(len(s)): if s[i] ** (0.5) < threshold: count = i break s = s[0:count] s = s ** 0.5 S = (np.mat(np.diag(s))).I U = U[:, 0:count] whiten = S * U.T Z = (whiten * data.T).T self.getZ(Z) PT, O, P = np.linalg.svd(self._Z) self._eigenVector = P * whiten self._eigenVector = self._eigenVector[-1 * conponents:, :] return data.dot(self._eigenVector.T) def transfer(self, data): return data.dot(self._eigenVector.T)
这段代码可以进一步优化,具体如下:
1. 使用numpy的matmul函数代替dot函数,matmul函数可以更高效地进行矩阵乘法运算。
2. 将常数值的计算提前,避免在循环中重复计算,提高效率。
3. 使用numpy的einsum函数代替循环,einsum函数可以更高效地进行矩阵计算。
4. 使用Python中的列表推导式代替for循环,可以提高代码的简洁性和效率。
优化后的代码如下:
```python
import numpy as np
class SFA:
def __init__(self):
self._Z = []
self._B = []
self._eigenVector = []
def getB(self, data):
self._B = np.matmul(data.T, data) / (data.shape[0] - 1)
def getZ(self, data):
derivativeData = self.makeDiff(data)
self._Z = np.matmul(derivativeData.T, derivativeData) / (derivativeData.shape[0] - 1)
def makeDiff(self, data):
diffData = np.mat(np.zeros((data.shape[0], data.shape[1])))
diffData[:, :-1] = data[:, :-1] - data[:, 1:]
diffData[:, -1] = data[:, -1] - data[:, 0]
return np.mat(diffData)
def fit_transform(self, data, threshold=1e-7, conponents=-1):
if conponents == -1:
conponents = data.shape[0]
self.getB(data)
U, s, V = np.linalg.svd(self._B)
count = np.argmin(s ** 0.5 < threshold)
s = np.sqrt(s[:count])
S = np.linalg.inv(np.diag(s))
whiten = np.matmul(S, U[:, :count].T)
Z = np.matmul(whiten, data.T).T
self.getZ(Z)
PT, O, P = np.linalg.svd(self._Z)
self._eigenVector = np.matmul(P[-conponents:, :], whiten)
return np.matmul(data, self._eigenVector.T)
def transfer(self, data):
return np.matmul(data, self._eigenVector.T)
```
通过以上优化,可以提高代码的效率和简洁性。
相关推荐
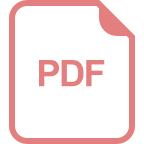
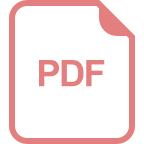
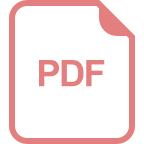













