(confMat(1,1)/sum(confMat(1,:))+confMat(2,2)/sum(confMat(2,:))+... confMat(3,3)/sum(confMat(3,:))+confMat(4,4)/sum(confMat(4,:))+confMat(5,5)/sum(confMat(5,:))+confMat(6,6)/sum(confMat(6,:))+... confMat(7,7)/sum(confMat(7,:))+confMat(8,8)/sum(confMat(8,:))+confMat(9,9)/sum(confMat(9,:)))/9
时间: 2023-11-23 08:07:22 浏览: 32
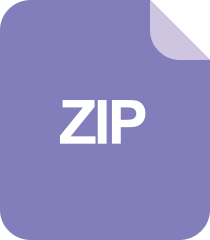
PyTorch学习日志.zip
这段代码是用来计算混淆矩阵的平均准确率的假设混淆矩阵为confMat,它是一个9x9的矩阵,表示9个类别的分类结果。代码中首先计算了每个类别的准确率,通过将对角线上的元素(即分类正确的样本数)除以对应行的总样本数来计算。然后将这些准确率相加,并除以类别数(9)来计算平均准确率。最后返回了平均准确率的值。
阅读全文
相关推荐
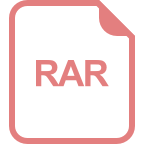
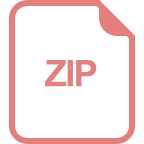


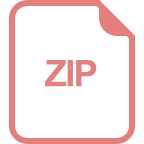
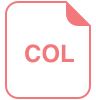
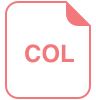
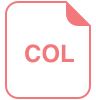
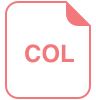
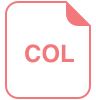
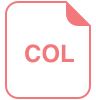






