df=pd.read_excel('C:/Users/86147/OneDrive/文档/66666.xlsx',header=0,usecols=(2,)) df.fillna(method='ffill',inplace=True) df.drop_duplicates(inplace=True) df.to_excel('xinshuju')这个代码怎么修改?
时间: 2024-02-15 19:02:30 浏览: 116
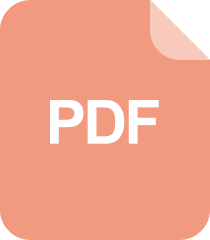
操作模式-详解pandas库pd.read_excel操作读取excel文件参数整理与实例
根据你的代码,我猜测你想将处理后的数据保存到名为 `xinshuju.xlsx` 的 Excel 文件中。但是你的代码中没有指定文件的后缀名,这可能会导致保存失败。另外,你需要指定保存的表单名称(sheet_name)。因此,你可以尝试以下代码:
```python
import pandas as pd
# 读取 Excel 文件
df = pd.read_excel('C:/Users/86147/OneDrive/文档/66666.xlsx', header=0, usecols=(2,))
# 用前一个非缺失值填充缺失值,删除重复行
df.fillna(method='ffill', inplace=True)
df.drop_duplicates(inplace=True)
# 保存数据到 Excel 文件
writer = pd.ExcelWriter('xinshuju.xlsx') # 指定文件名及后缀名
df.to_excel(writer, sheet_name='Sheet1', index=False) # 指定表单名称并去除行索引
writer.save()
```
这个代码将读取 `C:/Users/86147/OneDrive/文档/66666.xlsx` 中的数据,用前一个非缺失值填充缺失值,删除重复行,然后将处理后的数据保存到 `xinshuju.xlsx` 中的 `Sheet1` 表单中,且不包含行索引。
阅读全文
相关推荐
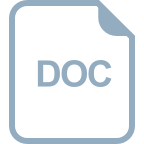
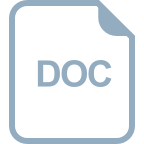














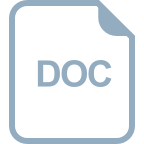
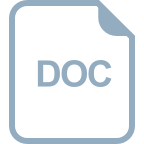
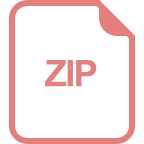