迪哥 diffusion
时间: 2023-10-02 11:09:40 浏览: 101
迪哥是一位在B站上分享关于图像生成模型的知识的UP主。他详细解读了一种名为Diffusion Model的图像生成模型,并提供了公式推导、论文精读以及代码示例等资料。Diffusion Model是一种比GAN更先进的图像生成模型,它通过扩散过程来生成图像。如果你对这个模型感兴趣,你可以观看迪哥在B站上的视频,并阅读他提供的参考资料深入了解。此外,他还提供了一份详细的文字原理解释和代码示例,你可以通过链接下载。
相关问题
逻辑回归python代码实现 迪哥
逻辑回归是一种机器学习算法,常用于二分类问题。下面是一个逻辑回归的Python代码实现的例子:
```python
import numpy as np
import pandas as pd
# Sigmoid函数,用于将预测结果转化为概率值
def sigmoid(z):
return 1 / (1 + np.exp(-z))
# 损失函数,用于评估模型的准确性
def cost(theta, X, y):
theta = np.matrix(theta)
X = np.matrix(X)
y = np.matrix(y)
first = np.multiply(-y, np.log(sigmoid(X * theta.T)))
second = np.multiply((1-y), np.log(1 - sigmoid(X * theta.T)))
return np.sum(first - second) / len(X)
# 梯度下降算法,用于最小化损失函数,得到最优参数
def gradientDescent(X, y, theta, alpha, iters):
temp = np.matrix(np.zeros(theta.shape))
parameters = int(theta.ravel().shape[1])
cost = np.zeros(iters)
for i in range(iters):
error = sigmoid(X * theta.T) - y
for j in range(parameters):
term = np.multiply(error, X[:,j])
temp[0,j] = theta[0,j] - (alpha / len(X)) * np.sum(term)
theta = temp
cost[i] = cost(theta, X, y)
return theta, cost
# 读取数据
data = pd.read_csv('data.csv')
# 添加一列全为1的特征列
data.insert(0, 'Ones', 1)
# 将数据转化为矩阵
cols = data.shape[1]
X = data.iloc[:,0:cols-1]
y = data.iloc[:,cols-1:cols]
X = np.matrix(X.values)
y = np.matrix(y.values)
theta = np.zeros([1,3])
# 设置学习率和迭代次数
alpha = 0.01
iters = 1000
# 执行梯度下降算法,得到最优参数
theta, cost = gradientDescent(X, y, theta, alpha, iters)
# 输出最优参数和损失函数值
print("最优参数:", theta)
print("损失函数值:", cost[-1])
```
pytorch实现神经网络进行气温预测 迪哥 完整代码
import torch
import torch.nn as nn
import torch.optim as optim
# 生成训练数据(示例数据)
x_train = torch.tensor([[1.0], [2.0], [3.0], [4.0], [5.0]])
y_train = torch.tensor([[10.0], [20.0], [30.0], [40.0], [50.0]])
# 定义神经网络模型
class Net(nn.Module):
def __init__(self):
super(Net, self).__init__()
self.fc = nn.Linear(1, 1) # 线性层
def forward(self, x):
output = self.fc(x)
return output
model = Net()
# 定义损失函数和优化器
criterion = nn.MSELoss()
optimizer = optim.SGD(model.parameters(), lr=0.01)
# 训练模型
num_epochs = 1000
for epoch in range(num_epochs):
# 前向传播
output = model(x_train)
loss = criterion(output, y_train)
# 反向传播及优化
optimizer.zero_grad()
loss.backward()
optimizer.step()
if (epoch+1) % 100 == 0:
print('Epoch [{}/{}], Loss: {:.4f}'.format(epoch+1, num_epochs, loss.item()))
# 使用模型进行气温预测
x_test = torch.tensor([[6.0], [7.0]])
predict = model(x_test)
print("预测结果:", predict.data)
阅读全文
相关推荐
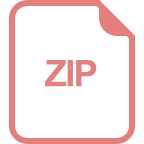
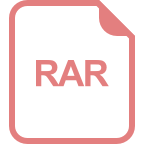
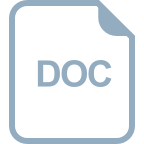
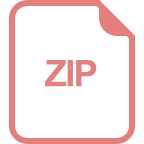
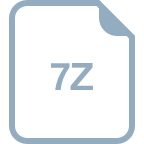
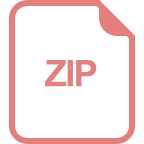
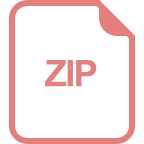
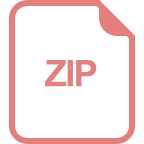