pytorch中1DCNN网络导入数据并划分训练集与测试集代码
时间: 2024-03-26 15:38:54 浏览: 148
以下是一个简单的1D卷积神经网络的PyTorch代码,包括数据导入和训练集/测试集划分:
```
import torch
import torch.nn as nn
import torch.optim as optim
from sklearn.model_selection import train_test_split
import numpy as np
# 假设数据集有10个特征,100个样本,输出有2个类别
input_dim = 10
output_dim = 2
# 生成随机数据
X = np.random.rand(100, input_dim)
y = np.random.randint(output_dim, size=100)
# 划分训练集和测试集
X_train, X_test, y_train, y_test = train_test_split(X, y, test_size=0.2, random_state=42)
# 转换数据为张量
X_train = torch.from_numpy(X_train).float()
X_test = torch.from_numpy(X_test).float()
y_train = torch.from_numpy(y_train).long()
y_test = torch.from_numpy(y_test).long()
# 定义模型
class CNN1D(nn.Module):
def __init__(self, input_dim, hidden_dim, output_dim):
super(CNN1D, self).__init__()
self.conv1 = nn.Conv1d(in_channels=input_dim, out_channels=hidden_dim, kernel_size=3, padding=1)
self.relu1 = nn.ReLU()
self.pool1 = nn.MaxPool1d(kernel_size=2, stride=2)
self.conv2 = nn.Conv1d(in_channels=hidden_dim, out_channels=hidden_dim, kernel_size=3, padding=1)
self.relu2 = nn.ReLU()
self.pool2 = nn.MaxPool1d(kernel_size=2, stride=2)
self.fc1 = nn.Linear(hidden_dim*8, output_dim)
def forward(self, x):
x = self.conv1(x)
x = self.relu1(x)
x = self.pool1(x)
x = self.conv2(x)
x = self.relu2(x)
x = self.pool2(x)
x = x.view(-1, self.num_flat_features(x))
x = self.fc1(x)
return x
def num_flat_features(self, x):
size = x.size()[1:]
num_features = 1
for s in size:
num_features *= s
return num_features
# 实例化模型、损失函数和优化器
model = CNN1D(input_dim, 16, output_dim)
criterion = nn.CrossEntropyLoss()
optimizer = optim.Adam(model.parameters(), lr=0.001)
# 训练模型
for epoch in range(100):
running_loss = 0.0
for i in range(len(X_train)):
optimizer.zero_grad()
outputs = model(X_train[i].unsqueeze(0).transpose(1,2))
loss = criterion(outputs, y_train[i])
loss.backward()
optimizer.step()
running_loss += loss.item()
print('Epoch %d loss: %.3f' % (epoch + 1, running_loss / len(X_train)))
# 测试模型
with torch.no_grad():
correct = 0
total = 0
for i in range(len(X_test)):
outputs = model(X_test[i].unsqueeze(0).transpose(1,2))
_, predicted = torch.max(outputs.data, 1)
total += 1
correct += (predicted == y_test[i]).sum().item()
print('Accuracy of the network on the %d test samples: %d %%' % (len(X_test), 100 * correct / total))
```
这个例子中,数据集有10个特征,100个样本,输出有2个类别。我们使用`train_test_split`函数将数据集划分为训练集和测试集。然后我们将数据转换为PyTorch张量,并定义模型、损失函数和优化器。我们使用随机梯度下降方法训练模型,并在每个epoch计算训练集上的平均损失。最后,我们使用测试集评估模型的准确率。
阅读全文
相关推荐
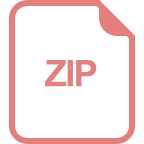
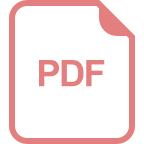
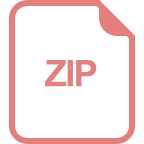















