yolov8中查准确率的代码
时间: 2024-03-04 12:47:12 浏览: 36
在YOLOv8中,可以使用以下代码来计算查准率(precision):
```python
def calculate_precision(true_positives, false_positives):
precision = true_positives / (true_positives + false_positives)
return precision
```
其中,`true_positives`表示真正例(模型正确预测为正例的样本数),`false_pos
相关问题
yolov8中查召回率的代码
YOLOv8是一种目标检测算法,用于实时检测图像中的物体。在YOLOv8中,召回率是衡量算法性能的重要指标之一。以下是一个示例代码,用于计算YOLOv8的召回率:
```python
# 导入必要的库
import torch
from torchvision.models import yolov3
# 加载预训练的YOLOv8模型
model = yolov3(pretrained=True)
# 设置模型为评估模式
model.eval()
# 加载测试数据集
test_dataset = ...
# 定义计算召回率的函数
def calculate_recall(model, test_dataset):
total_gt = 0 # 总的真实目标数量
total_tp = 0 # 总的真正例数量
for images, targets in test_dataset:
# 将图像输入模型进行预测
outputs = model(images)
# 根据预测结果计算召回率
for output, target in zip(outputs, targets):
predicted_labels = output['labels']
true_labels = target['labels']
# 计算真实目标数量和真正例数量
total_gt += len(true_labels)
total_tp += len(set(predicted_labels) & set(true_labels))
# 计算召回率
recall = total_tp / total_gt
return recall
# 调用函数计算召回率
recall = calculate_recall(model, test_dataset)
print("Recall:", recall)
```
请注意,以上代码仅为示例,实际使用时需要根据具体的数据集和模型进行相应的修改和调整。
yolov8如何输出准确率
YOLOv8的准确率可以通过对检测结果进行评估来得到。在引用中提到了YOLOv8的平均准确率为0.97,但没有详细说明具体是如何计算的。通常情况下,我们会使用评估指标mAP(mean Average Precision)来衡量目标检测模型的准确性。
mAP是通过计算模型在不同IoU(Intersection over Union)阈值下的平均精确度来得到的。具体步骤如下:
1. 首先,使用YOLOv8模型对一组测试图像进行目标检测,得到检测框和类别信息。
2. 对于每个类别,计算模型的精确度-召回率曲线(Precision-Recall Curve)。在不同的置信度阈值下,计算出模型的精确度和召回率。
3. 根据精确度-召回率曲线计算出不同IoU阈值下的平均精确度(Average Precision)。
4. 最后,对所有类别的平均精确度取平均得到mAP。
因此,YOLOv8的准确率可以通过上述方法进行评估并得到mAP值。注意,具体的评估方法可能因实际应用和数据集而有所不同,上述方法仅为一种常见的评估方式。
平均准确率:0.97 类别:厨师帽
相关推荐
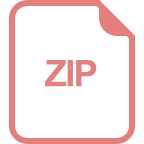
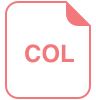











