per-maddpg代码
时间: 2023-12-29 10:02:30 浏览: 29
以下是Per-MADDPG的代码示例,主要涵盖了actor和critic的实现:
```python
import torch
import torch.nn as nn
import torch.optim as optim
import numpy as np
import random
import copy
from collections import deque, namedtuple
device = torch.device("cuda:0" if torch.cuda.is_available() else "cpu")
BUFFER_SIZE = int(1e6) # replay buffer size
BATCH_SIZE = 128 # minibatch size
GAMMA = 0.99 # discount factor
TAU = 1e-3 # for soft update of target parameters
LR_ACTOR = 1e-3 # learning rate of the actor
LR_CRITIC = 1e-3 # learning rate of the critic
WEIGHT_DECAY = 0 # L2 weight decay
UPDATE_EVERY = 2 # how often to update the network
NUM_UPDATE = 1 # how many times to update the network
class ReplayBuffer:
"""
Replay buffer class
"""
def __init__(self, buffer_size, batch_size):
"""
Initialize ReplayBuffer class
:param buffer_size: int, size of the replay buffer
:param batch_size: int, size of the batch
"""
self.memory = deque(maxlen=buffer_size) # internal memory (deque)
self.batch_size = batch_size
self.experience = namedtuple("Experience", field_names=["state", "action", "reward", "next_state", "done"])
def add(self, state, action, reward, next_state, done):
"""
Add an experience to memory.
:param state: current state
:param action: action taken
:param reward: reward received
:param next_state: next state
:param done: whether the episode is done
"""
e = self.experience(state, action, reward, next_state, done)
self.memory.append(e)
def sample(self):
"""
Randomly sample a batch of experiences from memory.
:return: tuple of torch tensors, state, action, reward, next_state, done
"""
experiences = random.sample(self.memory, k=self.batch_size)
states = torch.from_numpy(np.vstack([e.state for e in experiences if e is not None])).float().to(device)
actions = torch.from_numpy(np.vstack([e.action for e in experiences if e is not None])).float().to(device)
rewards = torch.from_numpy(np.vstack([e.reward for e in experiences if e is not None])).float().to(device)
next_states = torch.from_numpy(np.vstack([e.next_state for e in experiences if e is not None])).float().to(device)
dones = torch.from_numpy(np.vstack([e.done for e in experiences if e is not None]).astype(np.uint8)).float().to(device)
return (states, actions, rewards, next_states, dones)
def __len__(self):
"""
Return the current size of internal memory.
"""
return len(self.memory)
class Actor(nn.Module):
"""
Actor neural network
"""
def __init__(self, state_size, action_size, seed, fc1_units=256, fc2_units=128):
"""
Initialize Actor class
:param state_size: int, size of the state space
:param action_size: int, size of the action space
:param seed: int, random seed
:param fc1_units: int, number of neurons in the first hidden layer
:param fc2_units: int, number of neurons in the second hidden layer
"""
super(Actor, self).__init__()
self.seed = torch.manual_seed(seed)
self.fc1 = nn.Linear(state_size, fc1_units)
self.fc2 = nn.Linear(fc1_units, fc2_units)
self.fc3 = nn.Linear(fc2_units, action_size)
self.bn1 = nn.BatchNorm1d(fc1_units)
self.reset_parameters()
def reset_parameters(self):
"""
Initialize the weights of the neural network
"""
self.fc1.weight.data.uniform_(*self.hidden_init(self.fc1))
self.fc2.weight.data.uniform_(*self.hidden_init(self.fc2))
self.fc3.weight.data.uniform_(-3e-3, 3e-3)
def hidden_init(self, layer):
"""
Initialize the weights of the hidden layers
:param layer: layer of the neural network
:return: tuple of floats, initialized weights
"""
fan_in = layer.weight.data.size()[0]
lim = 1. / np.sqrt(fan_in)
return (-lim, lim)
def forward(self, state):
"""
Forward pass of the neural network
:param state: state input
:return: action output
"""
x = self.bn1(self.fc1(state))
x = torch.relu(x)
x = torch.relu(self.fc2(x))
return torch.tanh(self.fc3(x))
class Critic(nn.Module):
"""
Critic neural network
"""
def __init__(self, state_size, action_size, seed, fcs1_units=256, fc2_units=128):
"""
Initialize Critic class
:param state_size: int, size of the state space
:param action_size: int, size of the action space
:param seed: int, random seed
:param fcs1_units: int, number of neurons in the first hidden layer
:param fc2_units: int, number of neurons in the second hidden layer
"""
super(Critic, self).__init__()
self.seed = torch.manual_seed(seed)
self.fcs1 = nn.Linear(state_size, fcs1_units)
self.fc2 = nn.Linear(fcs1_units+action_size, fc2_units)
self.fc3 = nn.Linear(fc2_units, 1)
self.bn1 = nn.BatchNorm1d(fcs1_units)
self.reset_parameters()
def reset_parameters(self):
"""
Initialize the weights of the neural network
"""
self.fcs1.weight.data.uniform_(*self.hidden_init(self.fcs1))
self.fc2.weight.data.uniform_(*self.hidden_init(self.fc2))
self.fc3.weight.data.uniform_(-3e-3, 3e-3)
def hidden_init(self, layer):
"""
Initialize the weights of the hidden layers
:param layer: layer of the neural network
:return: tuple of floats, initialized weights
"""
fan_in = layer.weight.data.size()[0]
lim = 1. / np.sqrt(fan_in)
return (-lim, lim)
def forward(self, state, action):
"""
Forward pass of the neural network
:param state: state input
:param action: action input
:return: Q-value output
"""
xs = self.bn1(self.fcs1(state))
xs = torch.relu(xs)
x = torch.cat((xs, action), dim=1)
x = torch.relu(self.fc2(x))
return self.fc3(x)
class Agent():
"""
Agent class
"""
def __init__(self, state_size, action_size, num_agents, random_seed):
"""
Initialize Agent class
:param state_size: int, size of the state space
:param action_size: int, size of the action space
:param num_agents: int, number of agents
:param random_seed: int, random seed
"""
self.state_size = state_size
self.action_size = action_size
self.num_agents = num_agents
self.seed = random.seed(random_seed)
# Actor networks
self.actor_local = Actor(state_size, action_size, random_seed).to(device)
self.actor_target = Actor(state_size, action_size, random_seed).to(device)
self.actor_optimizer = optim.Adam(self.actor_local.parameters(), lr=LR_ACTOR)
# Critic networks
self.critic_local = Critic(state_size*num_agents, action_size*num_agents, random_seed).to(device)
self.critic_target = Critic(state_size*num_agents, action_size*num_agents, random_seed).to(device)
self.critic_optimizer = optim.Adam(self.critic_local.parameters(), lr=LR_CRITIC, weight_decay=WEIGHT_DECAY)
# Noise process
self.noise = OUNoise((num_agents, action_size), random_seed)
# Replay memory
self.memory = ReplayBuffer(BUFFER_SIZE, BATCH_SIZE)
def act(self, state, add_noise=True):
"""
Returns actions for given state as per current policy.
:param state: current state
:param add_noise: bool, whether to add noise
:return: array of actions
"""
state = torch.from_numpy(state).float().to(device)
self.actor_local.eval()
with torch.no_grad():
action = self.actor_local(state).cpu().data.numpy()
self.actor_local.train()
if add_noise:
action += self.noise.sample()
return np.clip(action, -1, 1)
def reset(self):
"""
Reset the noise process
"""
self.noise.reset()
def learn(self, experiences, gamma):
"""
Update policy and value parameters using given batch of experience tuples.
:param experiences: tuple of torch tensors, state, action, reward, next_state, done
:param gamma: float, discount factor
"""
states, actions, rewards, next_states, dones = experiences
# ---------------------------- update critic ---------------------------- #
# Get predicted next-state actions and Q values from target models
actions_next = self.actor_target(next_states)
q_targets_next = self.critic_target(next_states, actions_next)
# Compute Q targets for current states (y_i)
q_targets = rewards + (gamma * q_targets_next * (1 - dones))
# Compute critic loss
q_expected = self.critic_local(states, actions)
critic_loss = nn.MSELoss()(q_expected, q_targets)
# Minimize the loss
self.critic_optimizer.zero_grad()
critic_loss.backward()
torch.nn.utils.clip_grad_norm_(self.critic_local.parameters(), 1)
self.critic_optimizer.step()
# ---------------------------- update actor ---------------------------- #
# Compute actor loss
actions_pred = self.actor_local(states)
actor_loss = -self.critic_local(states, actions_pred).mean()
# Minimize the loss
self.actor_optimizer.zero_grad()
actor_loss.backward()
self.actor_optimizer.step()
# ----------------------- update target networks ----------------------- #
self.soft_update(self.critic_local, self.critic_target, TAU)
self.soft_update(self.actor_local, self.actor_target, TAU)
def soft_update(self, local_model, target_model, tau):
"""
Soft update model parameters.
θ_target = τ*θ_local + (1 - τ)*θ_target
:param local_model: PyTorch model (weights will be copied from)
:param target_model: PyTorch model (weights will be copied to)
:param tau: float, interpolation parameter
"""
for target_param, local_param in zip(target_model.parameters(), local_model.parameters()):
target_param.data.copy_(tau*local_param.data + (1.0-tau)*target_param.data)
class OUNoise:
"""
Ornstein-Uhlenbeck process
"""
def __init__(self, size, seed, mu=0., theta=0.15, sigma=0.2):
"""
Initialize OUNoise class
:param size: int, size of the noise
:param seed: int, random seed
:param mu: float, mean of the noise
:param theta: float, theta parameter
:param sigma: float, sigma parameter
"""
self.mu = mu * np.ones(size)
self.theta = theta
self.sigma = sigma
self.seed = random.seed(seed)
self.reset()
def reset(self):
"""
Reset the noise
"""
self.state = copy.copy(self.mu)
def sample(self):
"""
Update the noise state and return the current noise value
"""
x = self.state
dx = self.theta * (self.mu - x) + self.sigma * np.random.standard_normal(self.mu.shape)
self.state = x + dx
return self.state
```
在使用Per-MADDPG算法时,需要创建多个Agents对象,每个对象都有自己的actor和critic神经网络,并且每个对象都有自己的replay buffer和noise process。在每个时间步,每个智能体都会执行一次`act()`方法,得到它的动作,然后将其作为一个元组添加到replay buffer中。
然后,每个智能体都会从replay buffer中获取一个批次的经验元组,并使用这些元组来更新它们的actor和critic神经网络。在更新critic神经网络时,需要计算目标Q值,并使用MSE损失计算critic损失。在更新actor神经网络时,需要使用critic神经网络的输出来计算actor损失。最后,使用soft update方法更新目标网络。
相关推荐
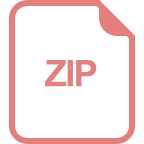
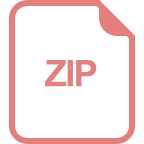
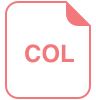













