feature_pyramid: true loss_args: type: AutoBCE batch_size: 8 epochs: 100 learning_rate: 0.001
时间: 2024-02-05 18:02:36 浏览: 77
根据您提供的配置参数,这是关于训练过程中的一些设置:
- `feature_pyramid: true`:这表示在训练过程中使用特征金字塔(Feature Pyramid)。特征金字塔是一种用于处理不同尺度的特征的技术,可以提高模型对不同大小物体的检测和分割能力。
- `loss_args`:这是关于损失函数的设置。在这里,`type: AutoBCE`表示使用自动二元交叉熵(Auto Binary Cross Entropy)作为损失函数。自动二元交叉熵是一种常用的用于二分类问题的损失函数。
- `batch_size: 8`:这表示每个训练批次中包含的样本数量为8。批量大小决定了在每次模型更新时用于计算梯度的样本数量。较大的批量大小可以加快训练速度,但可能会导致内存消耗增加。
- `epochs: 100`:这表示训练过程中的迭代次数为100。迭代次数指的是模型在整个训练集上进行参数更新的次数。较大的迭代次数可以提高模型性能,但也可能导致过拟合。
- `learning_rate: 0.001`:这是指训练过程中的学习率为0.001。学习率是控制模型参数更新步长的超参数,较小的学习率可以使模型收敛得更稳定,但可能需要更长的训练时间。
这些设置将影响训练过程中模型的行为和性能。如果还有其他问题,请随时提问!
相关问题
class TemporalBlock(nn.Module): """ Temporal block with the following layers: - 2x3x3, 1x3x3, spatio-temporal pyramid pooling - dropout - skip connection. """ def __init__(self, in_channels, out_channels=None, use_pyramid_pooling=False, pool_sizes=None): super().__init__() self.in_channels = in_channels self.half_channels = in_channels // 2 self.out_channels = out_channels or self.in_channels self.kernels = [(2, 3, 3), (1, 3, 3)] # Flag for spatio-temporal pyramid pooling self.use_pyramid_pooling = use_pyramid_pooling # 3 convolution paths: 2x3x3, 1x3x3, 1x1x1 self.convolution_paths = [] for kernel_size in self.kernels: self.convolution_paths.append( nn.Sequential( conv_1x1x1_norm_activated(self.in_channels, self.half_channels), CausalConv3d(self.half_channels, self.half_channels, kernel_size=kernel_size), ) ) self.convolution_paths.append(conv_1x1x1_norm_activated(self.in_channels, self.half_channels)) self.convolution_paths = nn.ModuleList(self.convolution_paths) agg_in_channels = len(self.convolution_paths) * self.half_channels if self.use_pyramid_pooling: assert pool_sizes is not None, "setting must contain the list of kernel_size, but is None." reduction_channels = self.in_channels // 3 self.pyramid_pooling = PyramidSpatioTemporalPooling(self.in_channels, reduction_channels, pool_sizes) agg_in_channels += len(pool_sizes) * reduction_channels # Feature aggregation self.aggregation = nn.Sequential( conv_1x1x1_norm_activated(agg_in_channels, self.out_channels),) if self.out_channels != self.in_channels: self.projection = nn.Sequential( nn.Conv3d(self.in_channels, self.out_channels, kernel_size=1, bias=False), nn.BatchNorm3d(self.out_channels), ) else: self.projection = None网络结构是什么?
这段代码实现了一个名为 TemporalBlock 的神经网络模块,该模块包括以下层:
- 3 个卷积路径: 2x3x3 卷积、1x3x3 卷积和 1x1x1 卷积
- dropout 层
- skip 连接
- 可选的 spatio-temporal pyramid pooling 层
- 最后是特征聚合和投影层(如果输入和输出通道数不同)
其中,卷积路径通过 nn.Sequential 和 nn.ModuleList 封装,方便调用和管理。
TemporalBlock 的输入通道数为 in_channels,输出通道数为 out_channels(如果未指定,则默认与输入通道数相同),通过 kernels 参数定义了 3 个卷积核的大小,其中前两个卷积路径使用 2x3x3 和 1x3x3 卷积核,第三个卷积路径使用 1x1x1 卷积核。
如果 use_pyramid_pooling 参数为 True,则会添加一个 spatio-temporal pyramid pooling 层,对输入进行空间和时间维度的金字塔池化,提取多尺度特征,其中 pool_sizes 参数指定了池化核的大小。
最后,特征聚合层将多个路径的特征拼接起来,通过 1x1x1 卷积将通道数调整为 out_channels,如果输入和输出通道数不同,则添加一个投影层进行通道数的调整。
def forward(self, *inputs): (x,) = inputs x_paths = [] for conv in self.convolution_paths: x_paths.append(conv(x)) x_residual = torch.cat(x_paths, dim=1) if self.use_pyramid_pooling: x_pool = self.pyramid_pooling(x) x_residual = torch.cat([x_residual, x_pool], dim=1) x_residual = self.aggregation(x_residual) if self.out_channels != self.in_channels: x = self.projection(x) x = x + x_residual return x网络计算过程
该模块的 forward 方法接收一个输入 x,并通过 3 个卷积路径分别对输入进行卷积,将卷积的结果拼接一个张量 x_residual。如果 use_pyramid_pool 为 True,则对输入进行 spatio-temporal pyramid pooling 操作,并将池化的结果与 x_residual 拼接起来。最后,将拼接后的张量 x_residual 通过一个 1x1x1 卷积层进行特征聚合,并将聚合的结果与输入张量 x 相加,得到最终输出。
如果输入和输出通道数不同,则通过投影层将输入 x 的通道数调整为 out_channels。
阅读全文
相关推荐
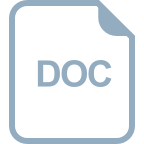
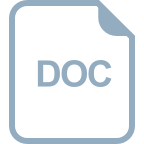
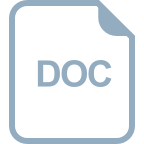
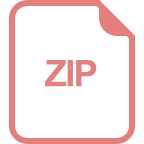
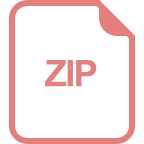
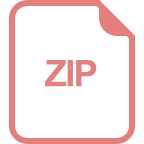
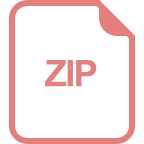
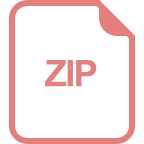
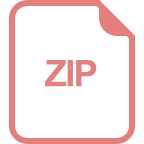
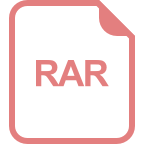
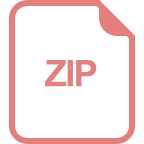
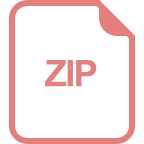
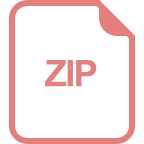
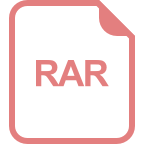
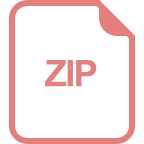


