请用python代码举例一个简单的使用过随机森林算法的例子
时间: 2024-05-10 07:19:22 浏览: 12
好的,以下是一个简单的使用随机森林算法的Python代码示例:
```python
from sklearn.ensemble import RandomForestClassifier
from sklearn.datasets import load_iris
from sklearn.model_selection import train_test_split
# 加载数据集
iris = load_iris()
X, y = iris.data, iris.target
# 将数据集拆分为训练集和测试集
X_train, X_test, y_train, y_test = train_test_split(X, y, test_size=0.3)
# 创建随机森林分类器
rfc = RandomForestClassifier(n_estimators=100)
# 训练模型
rfc.fit(X_train, y_train)
# 预测测试集
y_pred = rfc.predict(X_test)
# 输出准确率
print("Accuracy:", rfc.score(X_test, y_test))
```
上述代码演示了如何使用随机森林算法对鸢尾花数据集进行分类。我们首先加载数据集,然后将其拆分为训练集和测试集。接下来,我们创建了一个具有100个决策树的随机森林分类器,并使用训练数据拟合模型。最后,我们使用测试数据进行预测,并输出模型的准确率。
相关问题
请用Python代码举例随机森林算法
好的,下面是一个简单的随机森林分类器的Python代码示例:
```
from sklearn.ensemble import RandomForestClassifier
from sklearn.datasets import make_classification
# 创建一个随机森林分类器
clf = RandomForestClassifier(n_estimators=100)
# 使用make_classification生成一个虚拟的分类数据集
X, y = make_classification(n_samples=1000, n_features=4, n_informative=2, n_redundant=0, random_state=0, shuffle=False)
# 拟合模型
clf.fit(X, y)
# 预测新数据
print(clf.predict([[0, 0, 0, 0]]))
```
在这个例子中,我们使用了Scikit-learn库中的随机森林分类器。我们首先创建了一个分类器对象并指定了100个决策树。然后,我们使用`make_classification`函数创建了一个虚拟的分类数据集,该数据集包含4个特征和2个信息特征。最后,我们拟合模型并使用`predict`方法对新数据进行预测。
如何使用随机森林算法让一组数据可以预测另一组数据,请用python代码举例
随机森林算法可以用来进行监督学习,其中的决策树可以用来进行分类或回归。以下是使用随机森林算法进行回归预测的Python代码示例:
```python
# 导入需要的库
import pandas as pd
from sklearn.ensemble import RandomForestRegressor
from sklearn.model_selection import train_test_split
# 读取数据集
df = pd.read_csv('data.csv')
# 将数据集分为训练集和测试集
X_train, X_test, y_train, y_test = train_test_split(df.drop('target', axis=1), df['target'], test_size=0.2, random_state=42)
# 定义随机森林回归模型
rf_model = RandomForestRegressor(n_estimators=100, random_state=42)
# 训练模型
rf_model.fit(X_train, y_train)
# 用模型对测试集进行预测
y_pred = rf_model.predict(X_test)
# 输出预测结果
print(y_pred)
```
其中,`data.csv`是存储原始数据的文件,`target`是需要预测的目标变量。代码中,用`train_test_split`将数据集分为训练集和测试集,然后定义随机森林回归模型并用`fit`方法训练模型。最后用`predict`方法对测试集进行预测并输出预测结果。
相关推荐
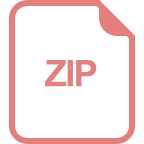








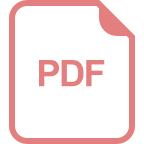
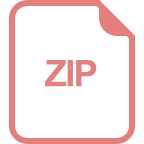
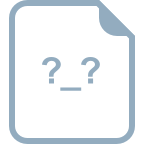