Python中用plt.imshow()显示图片时,如何设置图片保持原图的色彩
时间: 2024-12-25 18:35:27 浏览: 10
在Python的matplotlib库中,如果你想要`plt.imshow()`显示图片时保持原图的色彩,你需要确保在读取图片时设置了正确的颜色模式。通常,如果你的图片是以RGB(红绿蓝)或RGBA(红绿蓝及透明度)格式保存的,matplotlib会自动识别并保持原始色彩。
下面是一个简单的示例:
```python
import matplotlib.pyplot as plt
from PIL import Image
# 读取图片,保持原色彩
img = Image.open('your_image.jpg')
image_array = np.array(img)
# 使用imshow函数显示图片,注意数组形状应该是 (height, width, channels)
plt.imshow(image_array)
# 显示图像
plt.show()
```
在这个例子中,我们首先导入了PIL库来处理图片,然后使用`Image.open()`打开图片,将其转换为NumPy数组。由于NumPy默认读取图片时会保持原有色彩信息,所以无需额外设置。
如果图片是以其他颜色空间(如灰度、CMYK等)存储,可能需要先转换回RGB。在这种情况下,可以使用`image_array = img.convert('RGB')`将图片转换为RGB后再进行显示。
相关问题
for i in range(points1.shape[0]): # 第一幅图 im1 = cv.circle(im1, (points1[i][0].astype(int),points1[i][1].astype(int)), 10,get_color(points4D[i,2]), -1) # 第二幅图 tmp_point = np.dot(R,points4D[i,:].reshape(3,1)) + t tmp_point = tmp_point.reshape(-1) im2 = cv.circle(im2, (points2[i][0].astype(int),points2[i][1].astype(int)), 10,get_color(tmp_point[2]), -1) plt.subplot(121) plt.imshow(im1[:,:,::-1]) plt.subplot(122) plt.imshow(im2[:,:,::-1]) plt.show()这段代码有什么问题,请帮我修改一下
这段代码中,缺少对变量 `R` 和 `t` 的定义和初始化,而这两个变量是计算 `tmp_point` 的必要参数。因此,需要先定义和初始化这两个变量。
假设已经定义了 `R` 和 `t`,那么可以修改代码如下:
```python
# 定义和初始化 R 和 t
R = ...
t = ...
for i in range(points1.shape[0]):
# 第一幅图
im1 = cv.circle(im1, (points1[i][0].astype(int),points1[i][1].astype(int)), 10,get_color(points4D[i,2]), -1)
# 第二幅图
tmp_point = np.dot(R,points4D[i,:].reshape(3,1)) + t
tmp_point = tmp_point.reshape(-1)
im2 = cv.circle(im2, (points2[i][0].astype(int),points2[i][1].astype(int)), 10,get_color(tmp_point[2]), -1)
# 显示图像
plt.subplot(121)
plt.imshow(im1[:,:,::-1])
plt.subplot(122)
plt.imshow(im2[:,:,::-1])
plt.show()
```
另外,因为这段代码中用到了 `cv` 和 `plt` 两个模块,需要先导入这两个模块,例如:
```python
import cv2 as cv
import numpy as np
import matplotlib.pyplot as plt
```
import numpy as np import pandas as pd import matplotlib.pyplot as plt import PIL import torch from torchvision import transforms import torchvision #调用已经训练好的FCN语义分割网络 model = torchvision.models.segmentation.fcn_resnet101(pretrained=True) model.eval() #读取照片 image=PIL.Image.open('1234.jpg') #照片进行预处理 image_transf=transforms.Compose([ transforms.ToTensor(), transforms.Normalize(mean=[0.485,0.456,0.406], std=[0.229,0.224,0.225]) ] ) image_tensor=image_transf(image).unsqueeze(0) output=model(image_tensor)['out'] output.shape #读取图片,进行分割,总共21个通道,因为在21个数据集上训练 #转化为2维图像 outputarg=torch.argmax(output.squeeze(),dim=0).numpy() outputarg def decode_seqmaps(image,label_colors,nc=21): r=np.zeros_like(image).astype(np.uint8) g=np.zeros_like(image).astype(np.uint8) b=np.zeros_like(image).astype(np.uint8) for cla in range(0,nc): idx = image == cla r[idx] = label_colors[cla,0] g[idx] = label_colors[cla,1] b[idx] = label_colors[cla,2] rgbimage= np.stack([r,g,b],axis=2) return rgbimage import os os.environ["KMP_DUPLICATE_LIB_OK"]="TRUE" label_colors =np.array([(0,0,0), #0=background (128,0,0),(0,128,0),(128,128,0),(0,0,128), #1=airplane,2=bicycle,3=bird,4=boat (128,0,128),(0,128,128),(128,128,128),(64,0,0), #6=bus,7=car,8=cat,9=chair (192,0,0),(64,128,0),(192,128,0),(64,0,128), #10=cow,11=dining table,12=dog,13=horse (192,0,128),(64,128,128),(192,128,128),(0,64,0), #14=motorbike,15=person,16=potted plant,17=sheep (128,64,0),(0,192,0),(128,192,0),(0,64,128) #18=sofa,19=train,20=tv/monitor ]) outputrgb=decode_seqmaps(outputarg,label_colors) plt.figure(figsize=(20,8)) plt.subplot(1,2,1) plt.imshow(image) plt.axis('off') plt.subplot(1,2,2) plt.imshow(outputrgb) plt.axis('off') plt.subplots_adjust(wspace=0.05) plt.show()使用了哪些深度学习的模型和方法
这段代码是导入了一些常用的Python库和工具,包括:
- numpy:用于数组和矩阵运算的库;
- pandas:用于数据分析和处理的库;
- matplotlib:用于数据可视化的库;
- PIL:Python图像库,用于图像处理和操作;
- torch:PyTorch深度学习框架的库;
- transforms:PyTorch中用于数据预处理的工具;
- torchvision:PyTorch中用于构建计算机视觉模型的框架。
这些库和工具都是在开发计算机视觉模型时会经常用到的,可以方便地处理图像数据、构建模型并进行训练和验证。
阅读全文
相关推荐
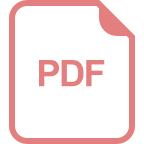
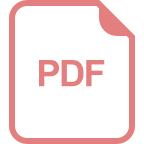
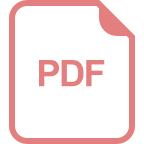
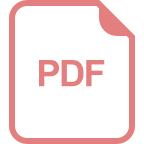
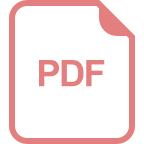
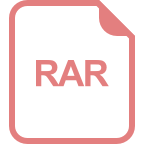
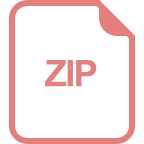
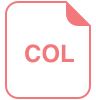
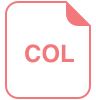
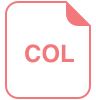






