Python积分与微分计算
时间: 2023-11-05 14:12:58 浏览: 66
Python中可以使用SciPy科学计算库进行积分和微分的计算。下面是一些简单的示例:
积分:
```python
from scipy.integrate import quad
def integrand(x):
return x**2
result, error = quad(integrand, 0, 1)
print(result) # 输出积分结果
```
上述代码中,使用`quad()`函数对函数`integrand()`进行积分,积分下界为0,积分上界为1。`quad()`函数返回积分结果和误差。
微分:
```python
import numpy as np
x = np.linspace(0, 1, 101) # 定义自变量
y = np.sin(x) # 定义因变量
dydx = np.gradient(y, x) # 计算y关于x的导数
print(dydx) # 输出导数结果
```
上述代码中,使用NumPy库中的`gradient()`函数计算函数`y`关于自变量`x`的导数。`gradient()`函数返回一个数组,表示函数在每个点上的导数值。
需要注意的是,这里的微分是数值微分,结果可能存在误差。如果需要精确计算微分,可以考虑使用符号计算库,如SymPy。
相关问题
python的微分与积分计算
Python中有很多库可以用来进行微积分计算,其中比较常用的有 SymPy 和 SciPy。
对于微分,SymPy库可以很方便地计算导数。例如,对于函数 y=x^3 + 2x^2 + x,可以使用以下代码计算它的导数:
```python
import sympy as sp
x = sp.Symbol('x')
y = x**3 + 2*x**2 + x
dy_dx = y.diff(x)
print(dy_dx)
```
输出结果为:
```
3*x**2 + 4*x + 1
```
对于积分,SymPy库同样可以很方便地计算不定积分和定积分。例如,对于函数 y=x^3 + 2x^2 + x,可以使用以下代码计算它的不定积分和定积分:
```python
import sympy as sp
x = sp.Symbol('x')
y = x**3 + 2*x**2 + x
int_y = sp.integrate(y, x)
int_y_limits = sp.integrate(y, (x, 0, 1))
print(int_y)
print(int_y_limits)
```
输出结果分别为:
```
x**4/4 + 2*x**3/3 + x**2/2
7/12
```
另外,SciPy库中也有很多用于数值计算的函数,包括微分和积分函数。例如,可以使用 `scipy.misc.derivative` 函数计算函数在某一点的导数,使用 `scipy.integrate.quad` 函数计算定积分。具体用法可以参考SciPy库的官方文档。
偏微分 蒙特卡洛 python
偏微分是微积分中的一种运算方法,用于求解多元函数的偏导数。偏微分可以将多元函数沿着某个变量的变化率表示出来,而将其他变量视为常数。蒙特卡洛方法是一种随机模拟方法,用于估计数学问题的数值解。在Python中,可以使用数值计算库如NumPy和SciPy来进行偏微分和蒙特卡洛模拟。您有具体的问题或者需要了解哪方面的内容吗?
相关推荐
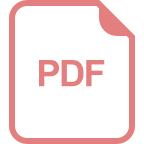












