Confounding-robust policy improvement的主要方法
时间: 2024-04-01 11:35:08 浏览: 15
Confounding-robust policy improvement是一种针对深度强化学习中混淆因素(confounding factors)对策略学习的影响的解决方案。该方法主要分为两个步骤:
1. 识别混淆因素:首先,通过建立一个混淆因素对策略性能的影响模型,来识别混淆因素。具体来说,该模型将原始状态和动作作为输入,预测策略性能的变化。如果预测的变化与真实的变化不一致,则表明该因素是混淆因素。
2. 改进策略:一旦混淆因素被识别,就可以尝试通过改进策略来降低其影响。具体来说,该方法采用了一种策略改进的算法,称为Confounding-robust Actor-Critic (CRAC),来使策略更加鲁棒。该算法的关键是在策略优化的过程中,将混淆因素的影响因素加入到了奖励函数中,从而使策略更加关注与目标任务相关的因素,减少混淆因素对策略学习的影响。
总的来说,Confounding-robust policy improvement的主要方法是通过混淆因素的识别和策略改进来提高深度强化学习的鲁棒性。
相关问题
post-hoc analysis
Post-hoc analysis refers to the analysis of data after an experiment, study, or survey has been conducted. It involves examining the data to identify patterns, trends, and relationships that were not initially anticipated or observed during the initial analysis. Post-hoc analysis is often used to explore the underlying factors that may have influenced the results of a study or experiment, and to identify potential confounding variables that may have affected the outcomes. It is important to note that post-hoc analysis is exploratory in nature and should be interpreted with caution, as it may produce false positive results or lead to overinterpretation of the data.
Please revise the paper:Accurate determination of bathymetric data in the shallow water zone over time and space is of increasing significance for navigation safety, monitoring of sea-level uplift, coastal areas management, and marine transportation. Satellite-derived bathymetry (SDB) is widely accepted as an effective alternative to conventional acoustics measurements over coastal areas with high spatial and temporal resolution combined with extensive repetitive coverage. Numerous empirical SDB approaches in previous works are unsuitable for precision bathymetry mapping in various scenarios, owing to the assumption of homogeneous bottom over the whole region, as well as the limitations of constructing global mapping relationships between water depth and blue-green reflectance takes no account of various confounding factors of radiance attenuation such as turbidity. To address the assumption failure of uniform bottom conditions and imperfect consideration of influence factors on the performance of the SDB model, this work proposes a bottom-type adaptive-based SDB approach (BA-SDB) to obtain accurate depth estimation over different sediments. The bottom type can be adaptively segmented by clustering based on bottom reflectance. For each sediment category, a PSO-LightGBM algorithm for depth derivation considering multiple influencing factors is driven to adaptively select the optimal influence factors and model parameters simultaneously. Water turbidity features beyond the traditional impact factors are incorporated in these regression models. Compared with log-ratio, multi-band and classical machine learning methods, the new approach produced the most accurate results with RMSE value is 0.85 m, in terms of different sediments and water depths combined with in-situ observations of airborne laser bathymetry and multi-beam echo sounder.
回答:本文提出了一种基于底部类型自适应的卫星深度测量(BA-SDB)方法,以解决传统卫星深度测量模型中假设底部均匀的局限性以及不能充分考虑影响反射率的干扰因素,如浊度等。通过基于聚类的底部反射率自适应分割,并采用PSO-LightGBM算法考虑多种因素,实现了对不同沉积物和水深的准确深度估算,与空中激光测深和多波束回声深度声纳联合实测结果相比,均方根误差值达到0.85m,是传统比值法、多波段法和机器学习方法中最准确的方法。
相关推荐
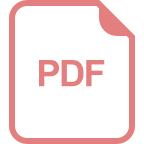
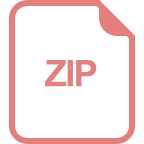
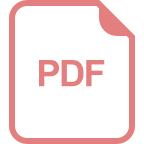
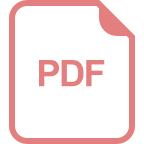
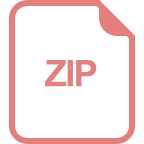
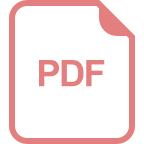
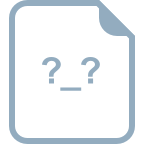
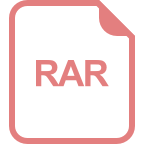
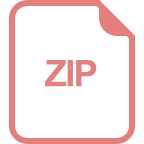
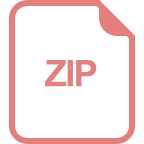
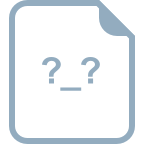
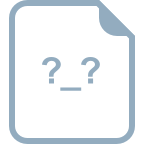
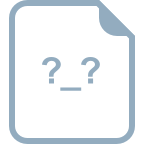
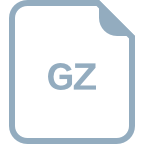
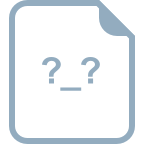