写一段基于pytorch的条件生成对抗神经网络用于生成新数据的代码
时间: 2023-03-04 16:33:27 浏览: 78
好的,下面是一段基于 PyTorch 的条件生成对抗神经网络代码,用于生成新数据:
首先导入所需的 PyTorch 模块和其他库:
```
import torch
import torch.nn as nn
import numpy as np
from torchvision import datasets, transforms
```
接下来定义生成器和判别器的网络结构:
```
class Generator(nn.Module):
def __init__(self, latent_dim, num_classes, img_shape):
super(Generator, self).__init__()
self.label_emb = nn.Embedding(num_classes, num_classes)
self.fc = nn.Linear(latent_dim + num_classes, 128)
self.bn1 = nn.BatchNorm1d(128, 0.8)
self.relu = nn.ReLU(inplace=True)
self.fc2 = nn.Linear(128, int(np.prod(img_shape)))
self.bn2 = nn.BatchNorm1d(int(np.prod(img_shape)), 0.8)
self.sigmoid = nn.Sigmoid()
self.img_shape = img_shape
def forward(self, noise, labels):
gen_input = torch.cat((self.label_emb(labels), noise), -1)
x = self.fc(gen_input)
x = self.bn1(x)
x = self.relu(x)
x = self.fc2(x)
x = self.bn2(x)
x = self.sigmoid(x)
img = x.view(x.size(0), *self.img_shape)
return img
class Discriminator(nn.Module):
def __init__(self, num_classes, img_shape):
super(Discriminator, self).__init__()
self.label_emb = nn.Embedding(num_classes, num_classes)
self.fc = nn.Linear(int(np.prod(img_shape)) + num_classes, 128)
self.bn1 = nn.BatchNorm1d(128, 0.8)
self.relu = nn.LeakyReLU(0.2, inplace=True)
self.fc2 = nn.Linear(128, 1)
self.sigmoid = nn.Sigmoid()
self.img_shape = img_shape
def forward(self, img, labels):
img_flat = img.view(img.size(0), -1)
dis_input = torch.cat((img_flat, self.label_emb(labels)), -1)
x = self.fc(dis_input)
x = self.bn1(x)
x = self.relu(x)
x = self.fc2(x)
x = self.sigmoid(x)
return x
```
然后定义其他所需的参数,包括图像大小、数据集、优化器和损失函数:
```
img_shape = (1, 28, 28)
num_classes = 10
latent_dim = 100
lr = 0.0002
b1 = 0.5
b2 = 0.999
batch_size = 64
n_epochs = 200
dataloader = torch.utils.data.DataLoader(
datasets.MNIST(
"../data",
train=True,
download=True,
transform=transforms.Compose(
[transforms.Resize(img_shape[1:]), transforms.ToTensor(), transforms.Normalize([0.5], [0.5])]
),
),
batch_size=batch_size,
shuffle=True,
)
device = torch.device("cuda" if torch.cuda.is_available() else "cpu")
generator = Generator(latent_dim, num_classes, img_shape).to(device)
discriminator = Discriminator(num_classes, img_shape).to(device)
adversarial_loss = nn.BCELoss()
optimizer_G = torch.optim.Adam(generator.parameters(), lr=lr, betas=(
相关推荐
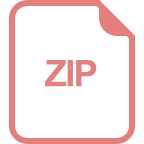
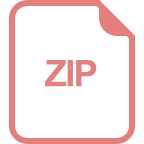
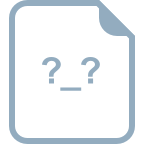











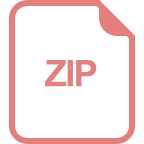
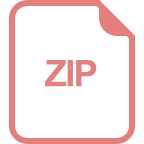
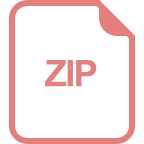