jupyter notebook波士顿房价预测
时间: 2023-10-16 12:09:49 浏览: 130
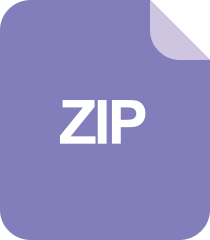
预测波士顿房价

好的,针对波士顿房价预测,您可以使用Jupyter Notebook进行建模和预测。以下是一些基本步骤:
1. 导入数据集:使用pandas库读取csv文件,将数据集导入到Jupyter Notebook中。
2. 数据清洗:检查数据集中是否有缺失值或异常值,并进行处理。
3. 特征工程:选择合适的特征,并进行特征缩放和转换。
4. 模型选择:选择适合问题的模型,如线性回归、决策树、随机森林等。
5. 模型训练:将数据集分为训练集和测试集,使用训练集对模型进行训练。
6. 模型评估:使用测试集对模型进行评估,计算出模型的准确率、均方误差等指标。
7. 模型优化:根据评估结果对模型进行优化,如调整超参数、增加特征等。
8. 模型预测:使用训练好的模型对新数据进行预测。
阅读全文
相关推荐
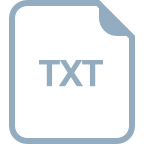


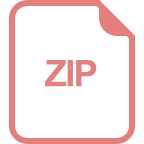
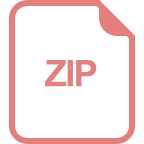
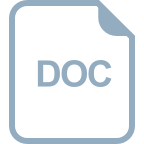




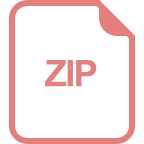
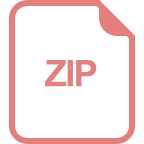
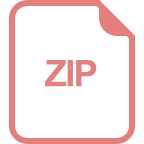
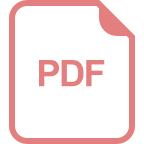
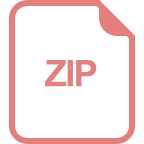
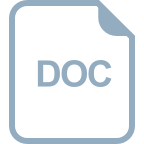