基于pytorch的函数拟合,用relu函数拟合正弦曲线的代码
时间: 2023-09-26 07:09:36 浏览: 101
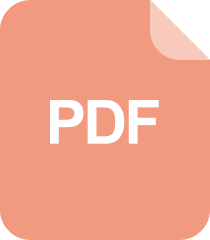
使用Pytorch来拟合函数方式
可以参考如下代码实现:
```
import torch
import torch.nn as nn
import numpy as np
import matplotlib.pyplot as plt
# 生成数据
x = np.arange(0, 10, 0.1)
y = np.sin(x)
# 定义模型
class Net(nn.Module):
def __init__(self):
super(Net, self).__init__()
self.fc1 = nn.Linear(1, 10)
self.fc2 = nn.Linear(10, 1)
self.relu = nn.ReLU()
def forward(self, x):
x = self.fc1(x)
x = self.relu(x)
x = self.fc2(x)
return x
# 初始化模型和优化器
net = Net()
optimizer = torch.optim.Adam(net.parameters(), lr=0.01)
loss_func = nn.MSELoss()
# 训练模型
for epoch in range(1000):
x_data = torch.from_numpy(x.reshape(-1, 1).astype(np.float32))
y_data = torch.from_numpy(y.reshape(-1, 1).astype(np.float32))
output = net(x_data)
loss = loss_func(output, y_data)
optimizer.zero_grad()
loss.backward()
optimizer.step()
# 绘制拟合曲线
x_test = np.arange(0, 10, 0.01)
x_test_data = torch.from_numpy(x_test.reshape(-1, 1).astype(np.float32))
net.eval()
y_test_data = net(x_test_data)
y_test = y_test_data.detach().numpy()
plt.plot(x, y, 'r-', label='sin')
plt.plot(x_test, y_test, 'b-', label='fitting curve')
plt.legend()
plt.show()
```
该代码中,首先生成了一组正弦曲线的数据,然后定义了一个包含两个全连接层和一个ReLU激活函数的神经网络模型。接着使用MSE损失函数和Adam优化器训练模型,并在训练结束后用测试数据绘制拟合曲线。
阅读全文
相关推荐
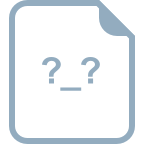
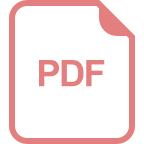













