遗传算法NSGA-Ⅱ求解带时间窗的多目标函数路径优化Python代码
时间: 2024-03-28 09:05:17 浏览: 133
以下是一个简单的示例代码,使用NSGA-II算法求解带时间窗的多目标函数路径优化问题。请注意,这只是一个基本的框架,你需要根据具体的问题进行适当的修改和扩展。
```python
import random
from deap import base, creator, tools, algorithms
# 定义问题参数
num_tasks = 10 # 任务数量
time_windows = [(0, 10), (5, 15), (10, 20), (8, 18), (12, 22), (15, 25), (20, 30), (18, 28), (22, 32), (25, 35)]
# 每个任务的时间窗限制,格式为(开始时间,结束时间)
# 定义遗传算法参数
population_size = 100
num_generations = 50
# 定义适应度函数
def evaluate(individual):
# 计算每个任务的开始时间和结束时间
start_times = [0] + individual
end_times = [start_times[i] + time_windows[i][1] for i in range(num_tasks)]
# 计算目标函数1:最小化总体完成时间
total_completion_time = max(end_times)
# 计算目标函数2:最小化违约惩罚
penalty = sum(max(0, end_times[i] - time_windows[i][1]) for i in range(num_tasks))
return total_completion_time, penalty
# 创建遗传算法的适应度函数
creator.create("FitnessMin", base.Fitness, weights=(-1.0, -1.0))
creator.create("Individual", list, fitness=creator.FitnessMin)
toolbox = base.Toolbox()
# 定义变量的取值范围
toolbox.register("attr_int", random.randint, 0, max(time_windows[i][1] for i in range(num_tasks)))
toolbox.register("individual", tools.initRepeat, creator.Individual, toolbox.attr_int, n=num_tasks)
toolbox.register("population", tools.initRepeat, list, toolbox.individual)
# 定义遗传算法操作
toolbox.register("evaluate", evaluate)
toolbox.register("mate", tools.cxTwoPoint)
toolbox.register("mutate", tools.mutUniformInt, low=0, up=max(time_windows[i][1] for i in range(num_tasks)), indpb=0.05)
toolbox.register("select", tools.selNSGA2)
def main():
# 创建初始种群
population = toolbox.population(n=population_size)
# 运行遗传算法
for generation in range(num_generations):
offspring = algorithms.varAnd(population, toolbox, cxpb=0.5, mutpb=0.1)
fits = toolbox.map(toolbox.evaluate, offspring)
for fit, ind in zip(fits, offspring):
ind.fitness.values = fit
population = toolbox.select(offspring + population, k=population_size)
# 输出最优解
best_individuals = tools.selBest(population, k=1)
best_fitness = evaluate(best_individuals[0])
print("Best individual:", best_individuals[0])
print("Best fitness:", best_fitness)
if __name__ == "__main__":
main()
```
这段代码使用DEAP库来实现NSGA-II算法。首先,我们定义了问题参数,包括任务数量和每个任务的时间窗限制。然后,我们定义了适应度函数,其中计算了两个目标函数:最小化总体完成时间和最小化违约惩罚。接下来,我们使用DEAP的工具函数来创建遗传算法的操作,并定义了变量的取值范围。最后,我们运行遗传算法并输出最优解。
请注意,这只是一个简单的示例代码,你需要根据具体的问题进行适当的修改和扩展,例如添加约束条件、调整遗传算法的参数等。希望对你有所帮助!
阅读全文
相关推荐
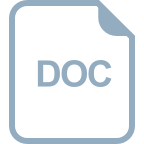
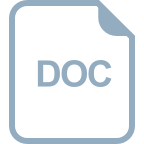
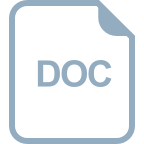
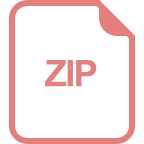
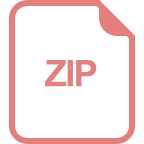
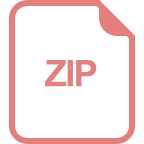
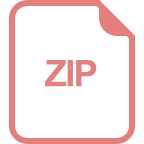
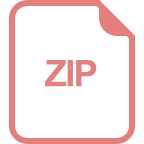
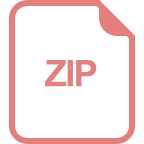
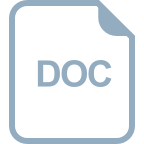
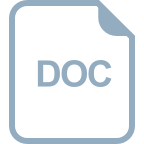
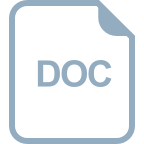






