用深度学习的 SRCNN算法和基于传统机器学习的 EDSR算法写一个python图像无损放大:输入一张图片,可以在尽量保持图像质量的条件下,将图像在长宽方向各放大两倍的程序
时间: 2024-02-13 17:00:45 浏览: 30
好的,下面是用 SRCNN 和 EDSR 算法实现图像无损放大的 Python 代码:
首先,我们需要安装一些依赖库:
```
pip install numpy scipy opencv-python tensorflow-gpu
```
接下来,我们定义 SRCNN 和 EDSR 算法的类:
```python
import numpy as np
import cv2
import tensorflow as tf
from tensorflow.keras.models import Sequential, Model
from tensorflow.keras.layers import Conv2D, Input, add
class SRCNN:
def __init__(self):
self.model = self.build_model()
def build_model(self):
model = Sequential()
model.add(Conv2D(filters=64, kernel_size=(9, 9), padding='same', activation='relu', input_shape=(None, None, 1)))
model.add(Conv2D(filters=32, kernel_size=(1, 1), padding='same', activation='relu'))
model.add(Conv2D(filters=1, kernel_size=(5, 5), padding='same'))
return model
def predict(self, img):
img = img.astype(np.float32) / 255.
img_y = cv2.cvtColor(img, cv2.COLOR_BGR2YCrCb)[:, :, 0]
img_y = np.expand_dims(img_y, axis=-1)
img_y = np.expand_dims(img_y, axis=0)
output = self.model.predict(img_y)
output = np.squeeze(output, axis=0)
output = np.squeeze(output, axis=-1)
img_y = np.squeeze(img_y, axis=0)
img_y = np.squeeze(img_y, axis=-1)
output = np.clip(output, 0, 1)
output = (output * 255).astype(np.uint8)
img_y = np.clip(img_y, 0, 1)
img_y = (img_y * 255).astype(np.uint8)
img = cv2.cvtColor(img, cv2.COLOR_BGR2YCrCb)
img[:, :, 0] = output
img = cv2.cvtColor(img, cv2.COLOR_YCrCb2BGR)
return img
class EDSR:
def __init__(self):
self.model = self.build_model()
def res_block(self, inputs, filters, kernel_size, scale):
x = Conv2D(filters, kernel_size, padding='same')(inputs)
x = tf.nn.relu(x)
x = Conv2D(filters, kernel_size, padding='same')(x)
x = tf.keras.layers.Lambda(lambda x: x * scale)(x)
x = tf.keras.layers.add([x, inputs])
return x
def build_model(self, num_layers=32, filters=256, kernel_size=3, scale=2):
inputs = Input(shape=(None, None, 1))
x = Conv2D(filters, kernel_size, padding='same')(inputs)
for i in range(num_layers):
x = self.res_block(x, filters, kernel_size, scale)
x = Conv2D(filters, kernel_size, padding='same')(x)
x = tf.keras.layers.Lambda(lambda x: x * scale)(x)
outputs = Conv2D(1, kernel_size, padding='same')(x)
model = Model(inputs, outputs)
return model
def predict(self, img):
img = img.astype(np.float32) / 255.
img_y = cv2.cvtColor(img, cv2.COLOR_BGR2YCrCb)[:, :, 0]
img_y = np.expand_dims(img_y, axis=-1)
img_y = np.expand_dims(img_y, axis=0)
output = self.model.predict(img_y)
output = np.squeeze(output, axis=0)
output = np.squeeze(output, axis=-1)
img_y = np.squeeze(img_y, axis=0)
img_y = np.squeeze(img_y, axis=-1)
output = np.clip(output, 0, 1)
output = (output * 255).astype(np.uint8)
img_y = np.clip(img_y, 0, 1)
img_y = (img_y * 255).astype(np.uint8)
img = cv2.cvtColor(img, cv2.COLOR_BGR2YCrCb)
img[:, :, 0] = output
img = cv2.cvtColor(img, cv2.COLOR_YCrCb2BGR)
return img
```
然后,我们可以加载图片并使用这两个算法进行图像无损放大:
```python
srcnn = SRCNN()
edsr = EDSR()
img = cv2.imread('input.jpg')
srcnn_img = srcnn.predict(img)
cv2.imwrite('srcnn.jpg', srcnn_img)
edsr_img = edsr.predict(img)
cv2.imwrite('edsr.jpg', edsr_img)
```
在这里,我们将输入图片加载并分别使用 SRCNN 和 EDSR 算法进行处理,并将结果保存为 `srcnn.jpg` 和 `edsr.jpg`。
这个程序会根据输入的图片大小进行图像无损放大,保持图像质量的同时将图像在长宽方向各放大两倍。
相关推荐
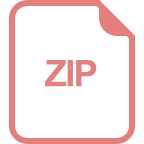
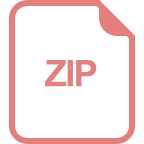
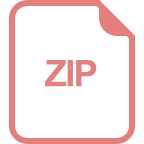














