Python+SA函数
时间: 2024-02-16 20:58:26 浏览: 81
根据提供的引用内容,我了解到Python中的函数是一种可重复使用的代码块,可以接受输入参数并返回输出结果。下面是一个示例:
```python
# 导入模块
from greet import greet_user
# 调用函数
names = ['Alice', 'Bob', 'Charlie']
greet_user(names)
```
这个示例中,我们从greet模块中导入了greet_user函数,并传入一个名字列表作为参数。函数会遍历列表中的每个名字,并打印出"Hello! "加上名字。同时,函数还会修改列表中的每个名字,将其加上一个数字后重新赋值。
相关问题
python的sa函数
模拟退火算法(Simulated Annealing)是一种优化算法,用于在一个大的搜索空间中找到一个近似最优解。它模拟了固体退火的过程,将固体从高温逐渐降温到低温,通过随机走动来达到更稳定的状态。
在Python中,常用的模拟退火算法函数是`scipy.optimize.anneal()`函数。这个函数可以用来求解一些优化问题,通过调整参数和目标函数,找到最优解。
pso+sa算法(粒子群和模拟退火算法的结合算法)_源码
### 回答1:
PSO SA算法是将粒子群算法和模拟退火算法结合在一起的一种优化算法,它综合了两种算法的优点,能够更高效地搜索最优解。
以下是PSO SA算法的源码示例:
```python
import numpy as np
def cost_function(x):
return x[0]**2 + x[1]**2 # 自定义目标函数,此处以二维函数为例
def pso_sa(num_particles, max_iterations, dimensions, max_temperature):
# 初始化粒子群的位置和速度
particles_position = np.random.uniform(low=-10, high=10, size=(num_particles, dimensions))
particles_velocity = np.zeros((num_particles, dimensions))
# 初始化全局最优解和个体最优解
global_best_position = np.zeros(dimensions)
global_best_cost = float('inf')
particles_best_position = np.zeros((num_particles, dimensions))
particles_best_cost = np.ones(num_particles) * float('inf')
# 初始化模拟退火参数
temperature = max_temperature
for iteration in range(max_iterations):
for i in range(num_particles):
# 更新粒子速度和位置
particles_velocity[i] = 0.5 * particles_velocity[i] + 0.2 * np.random.uniform() * (particles_best_position[i] - particles_position[i]) + 0.2 * np.random.uniform() * (global_best_position - particles_position[i])
particles_position[i] += particles_velocity[i]
# 判断是否超出范围
for j in range(dimensions):
particles_position[i][j] = np.clip(particles_position[i][j], -10, 10)
# 计算适应度值
cost = cost_function(particles_position[i])
# 更新个体最优解
if cost < particles_best_cost[i]:
particles_best_cost[i] = cost
particles_best_position[i] = particles_position[i]
# 更新全局最优解
if cost < global_best_cost:
global_best_cost = cost
global_best_position = particles_position[i]
# 模拟退火过程
for i in range(dimensions):
random_offset = np.random.uniform(low=-1, high=1)
new_position = global_best_position + random_offset
new_cost = cost_function(new_position)
if new_cost < global_best_cost:
global_best_cost = new_cost
global_best_position = new_position
elif np.exp((global_best_cost - new_cost) / temperature) > np.random.uniform():
global_best_cost = new_cost
global_best_position = new_position
# 降低温度
temperature *= 0.95
return global_best_position, global_best_cost
# 测试算法
best_position, best_cost = pso_sa(num_particles=50, max_iterations=100, dimensions=2, max_temperature=10)
print("最优解: ", best_position)
print("最优值: ", best_cost)
```
以上是一个简化的PSO SA算法的源码示例。在该代码中,我们实现了粒子群算法和模拟退火算法的结合。可以根据需要自定义目标函数、粒子数量、迭代次数和维度等参数,并获取最优解和最优值。
希望可以帮助到您!
### 回答2:
PSO-SA算法是粒子群优化算法(Particle Swarm Optimization)和模拟退火算法(Simulated Annealing)的结合算法。它综合了两种算法的优点,在求解复杂问题时具有较高的效率和准确性。
下面是一个简单的PSO-SA算法的源码示例:
```
import random
import math
class Particle:
def __init__(self, x0):
self.position_i = [] # 粒子位置
self.velocity_i = [] # 粒子速度
self.pos_best_i = [] # 粒子历史最佳位置
self.err_best_i = -1 # 粒子历史最佳误差
self.err_i = -1 # 粒子当前误差
for i in range(0, num_dimensions):
self.velocity_i.append(random.uniform(-1, 1))
self.position_i.append(x0[i])
# 更新粒子位置和速度
def update_velocity(self, pos_best_g):
w = 0.5 # 惯性权重
c1 = 1 # 学习因子
c2 = 2 # 学习因子
for i in range(0, num_dimensions):
r1, r2 = random.random(), random.random()
# 更新速度
vel_cognitive = c1 * r1 * (self.pos_best_i[i] - self.position_i[i])
vel_social = c2 * r2 * (pos_best_g[i] - self.position_i[i])
self.velocity_i[i] = w * self.velocity_i[i] + vel_cognitive + vel_social
# 限制速度范围
if self.velocity_i[i] > max_velocity:
self.velocity_i[i] = max_velocity
elif self.velocity_i[i] < -max_velocity:
self.velocity_i[i] = -max_velocity
# 更新粒子位置
def update_position(self, bounds):
for i in range(0, num_dimensions):
self.position_i[i] = self.position_i[i] + self.velocity_i[i]
# 限制位置范围
if self.position_i[i] > bounds[i][1]:
self.position_i[i] = bounds[i][1]
elif self.position_i[i] < bounds[i][0]:
self.position_i[i] = bounds[i][0]
# 计算粒子适应度
def evaluate(self, cost_func):
self.err_i = cost_func(self.position_i)
if self.err_i < self.err_best_i or self.err_best_i == -1:
self.pos_best_i = self.position_i
self.err_best_i = self.err_i
class PSO_SA:
def __init__(self, cost_func, x0, bounds, num_particles, max_iter, max_velocity):
global num_dimensions
num_dimensions = len(x0)
err_best_g = -1 # 全局最佳误差
pos_best_g = [] # 全局最佳位置
swarm = []
for i in range(0, num_particles):
swarm.append(Particle(x0))
# 开始优化过程
i = 0
while i < max_iter:
for j in range(0, num_particles):
swarm[j].evaluate(cost_func) # 计算粒子适应度
if swarm[j].err_i < err_best_g or err_best_g == -1:
pos_best_g = list(swarm[j].position_i)
err_best_g = float(swarm[j].err_i)
for j in range(0, num_particles):
swarm[j].update_velocity(pos_best_g) # 更新粒子速度
swarm[j].update_position(bounds) # 更新粒子位置
# 模拟退火过程
t = 1.0 - float(i) / max_iter
for j in range(0, num_particles):
delta = random.uniform(-1, 1)
for k in range(0, num_dimensions):
swarm[j].position_i[k] += delta * t
i += 1
# 输出优化结果
print("最优解: ", pos_best_g)
print("最小误差: ", err_best_g)
```
这是一个包含PSO和SA算法的简单的粒子群优化算法实现。该算法通过随机初始化粒子群的位置和速度,并在每一代中更新粒子的速度和位置,通过计算适应度函数来评估粒子的优劣。同时,在优化过程中还加入了模拟退火过程,以增加算法的全局搜索能力。最终输出结果为找到的最优解以及对应的最小误差。
通过这个源码示例,我们可以看到PSO-SA算法的实现过程。当然,这只是一个简单的实现,实际应用中还需要根据具体问题进行调整和优化。
### 回答3:
PSO-SA算法是一种将粒子群算法(Particle Swarm Optimization,PSO)和模拟退火算法(Simulated Annealing,SA)进行结合的优化算法。其源码如下:
```python
import numpy as np
def pso_sa(cost_function, bounds, num_particles, max_iter):
# 初始化粒子群
particles = np.random.uniform(bounds[0], bounds[1], (num_particles, len(bounds)))
best_pos = np.copy(particles)
best_cost = np.full(num_particles, float('inf'))
# 初始化模拟退火参数
temperature = 100
cooling_rate = 0.95
global_best_pos = None
global_best_cost = float('inf')
for i in range(max_iter):
for j in range(num_particles):
# 更新粒子位置
particles[j] += np.random.uniform(-1, 1) * (best_pos[j] - particles[j]) + np.random.uniform(-1, 1) * (global_best_pos - particles[j])
# 限定粒子位置在搜索空间范围内
particles[j] = np.clip(particles[j], bounds[0], bounds[1])
# 计算粒子的目标函数值
cost = cost_function(particles[j])
# 更新局部最优解和全局最优解
if cost < best_cost[j]:
best_cost[j] = cost
best_pos[j] = np.copy(particles[j])
if cost < global_best_cost:
global_best_cost = cost
global_best_pos = np.copy(particles[j])
# 模拟退火过程
delta_cost = cost - best_cost[j]
if delta_cost > 0 and np.exp(-delta_cost / temperature) < np.random.uniform(0, 1):
particles[j] = np.copy(best_pos[j])
# 降低温度
temperature *= cooling_rate
return global_best_pos
# 示例应用:求解一元函数 f(x) = x^2 的最小值
def cost_function(x):
return x**2
bounds = [-10, 10] # 搜索空间范围为 [-10, 10]
num_particles = 100 # 粒子数量为 100
max_iter = 100 # 迭代次数为 100
result = pso_sa(cost_function, bounds, num_particles, max_iter)
print("最小值点的坐标为:", result)
print("最小值为:", cost_function(result))
```
该源码实现了PSO-SA算法的主要逻辑。首先,根据给定的搜索空间范围、粒子数量和迭代次数,初始化粒子群和模拟退火参数。然后,通过迭代更新粒子位置,并计算粒子的目标函数值。在更新过程中,根据目标函数值的变化和模拟退火策略,选择是否接受新的位置。最后,返回全局最优解的坐标。
该源码中给出了一个示例应用,求解一元函数f(x) = x^2的最小值。根据定义的目标函数和搜索空间范围,运行PSO-SA算法,得到最小值点的坐标和最小值。
阅读全文
相关推荐
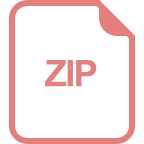
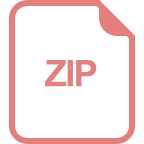
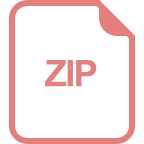
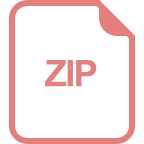
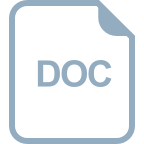
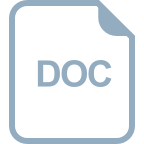
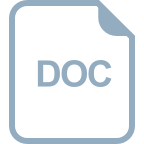
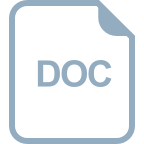
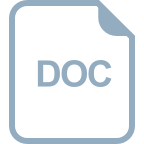
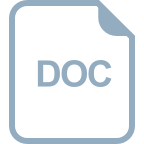
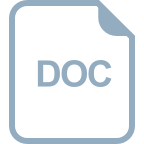
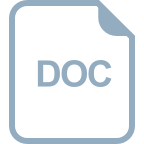

