import pandas as pd import numpy as np # 导入数据 df = pd.read_csv("E:\data2.csv") # 根据label列分组并计数 df_label = df.groupby("LBDSCHSI").size() # 转化为作图所需要的array格式,如:([[ 0, 37536],[ 1, 12322]]) datas = list(zip(df_label.index.to_list(), df_label.to_list())) datas= np.array([[0,2.83],[2.83,5.20],[5.20,6.45],[6.45,8.0]]) # 绘制饼图 import matplotlib.pyplot as plt # 设置字体和编码格式 plt.rcParams['font.sans-serif'] = 'simhei' plt.rcParams['axes.unicode_minus'] = False # 添加数据,标签,比例 plt.pie(datas[:, 1],labels=datas[:, 0],autopct="%.2f%%") # 在右上方添加图例 plt.legend(datas[:, 0], loc="upper right") # 添加标题 plt.title("LBDSCHSI(胆固醇)含量分析") # 展示饼图 plt.show()
时间: 2024-01-16 19:01:56 浏览: 111
这段代码使用 Pandas 和 Matplotlib 库,读取了一个 CSV 文件,对其中的数据进行处理,然后绘制了一个饼图。具体来说:
- `pd.read_csv("E:\data2.csv")` 读取了一个名为 data2.csv 的 CSV 文件,并将其存储在一个 Pandas 数据帧中。
- `df.groupby("LBDSCHSI").size()` 根据 LBDSCHSI 列中的数值进行分组,并计算每组中的数量。
- `list(zip(df_label.index.to_list(), df_label.to_list()))` 将分组计数结果转化为一个列表,其中每个元素都是一个元组,包含了 LBDSCHSI 值和对应的计数值。
- `np.array([[0, 2.83], [2.83, 5.20], [5.20, 6.45], [6.45, 8.0]])` 提供了一个硬编码的数据 array,用于绘制饼图。其中每个元素都是一个列表,包含了一个区间的起点和终点,以及对应的计数值。
- `plt.pie(datas[:, 1],labels=datas[:, 0],autopct="%.2f%%")` 使用 Matplotlib 的 `pie()` 函数绘制饼图,其中 `datas[:, 1]` 表示用于绘制饼图的数据,`datas[:, 0]` 表示每个数据对应的标签,`autopct="%.2f%%"` 表示在饼图上显示每个区域的百分比。
- `plt.legend(datas[:, 0], loc="upper right")` 在饼图的右上方添加图例,其中 `loc="upper right"` 表示图例的位置在右上方。
- `plt.title("LBDSCHSI(胆固醇)含量分析")` 添加饼图的标题。
- `plt.show()` 展示饼图。
阅读全文
相关推荐
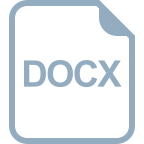
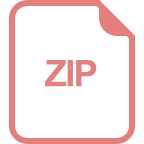
















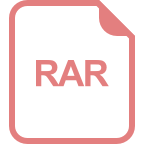