调用gcn函数的main使用python
时间: 2023-12-03 17:02:34 浏览: 81
以下是一个使用Python调用GCN函数的示例代码:
```python
import numpy as np
import tensorflow as tf
from gcn import gcn_layer
# 构造输入数据
X = np.random.randn(50, 100) # 50个节点,每个节点有100个特征
A = np.random.randn(50, 50) # 50个节点的邻接矩阵
# 构造模型
input_x = tf.placeholder(tf.float32, shape=[None, 100])
input_a = tf.placeholder(tf.float32, shape=[None, None])
hidden_size = 64
output_size = 10
hidden1 = gcn_layer(input_x, input_a, hidden_size, name='hidden1')
hidden2 = gcn_layer(hidden1, input_a, hidden_size, name='hidden2')
output = gcn_layer(hidden2, input_a, output_size, activation=tf.nn.softmax, name='output')
# 运行模型
with tf.Session() as sess:
sess.run(tf.global_variables_initializer())
y_pred = sess.run(output, feed_dict={input_x: X, input_a: A})
print(y_pred)
```
在这个示例中,我们首先构造了一个50个节点、每个节点有100个特征的输入数据X,以及一个50个节点的邻接矩阵A。然后,我们使用TF构造了一个3层的GCN模型,其中每层都使用GCN函数实现。在运行模型时,我们将输入数据X和邻接矩阵A作为feed_dict传递给模型,得到输出结果y_pred。
阅读全文
相关推荐















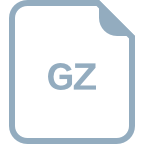

