matlab代码下载关于蚁群算法动态避障
时间: 2023-06-06 16:02:18 浏览: 74
蚁群算法动态避障是一种基于蚂蚁觅食行为的智能算法,在机器人路径规划、自动驾驶、无人机控制等领域有广泛应用。在Matlab中实现这种算法,需要以下步骤:
首先,要定义机器人的状态空间,包括位置、速度、方向等参数。然后,构建环境模型,包括地形、障碍物、目标等。利用传感器获得环境信息,如距离、角度、速度等,并将其转化为数字信号。
进一步,根据蚂蚁觅食行为的规则,建立蚁群模型。这包括设定信息素的初始值、更新规则、挥发速度等参数。通过模拟蚂蚁的寻食路径,不断更新信息素值,最终找到最优的路径。
在避障方面,需要解决机器人与障碍物的距离关系,采用奖惩机制来引导机器人避开障碍物。具体来说,如果机器人在一段时间内没有成功避开障碍物,就会受到负面奖励,反之则得到正面奖励。
最后,运用Matlab的图形界面工具,将机器人在环境中的移动过程可视化,可以更加直观地观察机器人的运行情况。同时,将代码封装为函数或对象,方便重复使用和扩展。
总之,蚁群算法动态避障的Matlab代码实现需要考虑多方面的因素,如环境建模、蚁群模型构建、避障奖惩机制等。只有细致周到地处理这些问题,才能实现一个高效、鲁棒的程序。
相关问题
机械臂避障路径规划蚁群算法matlab完整代码
以下是机械臂避障路径规划蚁群算法的 MATLAB 完整代码:
```matlab
clear all;
close all;
clc;
% 参数设置
start_point = [0, 0]; % 起点
end_point = [10, 10]; % 终点
obstacle = [4, 4]; % 障碍物
alpha = 1; % 信息素重要程度因子
beta = 5; % 启发函数重要程度因子
rho = 0.5; % 信息素挥发因子
Q = 1; % 常系数
ant_count = 50; % 蚂蚁数量
iter_max = 500; % 最大迭代次数
d = 2; % 坐标维数
t0 = 1; % 初始信息素浓度
eta = 1 / (norm(end_point - start_point)); % 启发函数
% 初始化信息素矩阵
tau = t0 * ones(d + 1, d + 1);
tau(end, :) = 0;
tau(:, end) = 0;
% 绘制地图
figure(1);
hold on;
grid on;
axis([0 12 0 12]);
plot(start_point(1), start_point(2), 'ro', 'MarkerSize', 10);
plot(end_point(1), end_point(2), 'ro', 'MarkerSize', 10);
plot(obstacle(1), obstacle(2), 's', 'MarkerSize', 10, 'MarkerFaceColor', 'r');
text(start_point(1) + 0.5, start_point(2) + 0.5, 'Start');
text(end_point(1) + 0.5, end_point(2) + 0.5, 'End');
text(obstacle(1) + 0.5, obstacle(2) + 0.5, 'Obstacle');
xlabel('X');
ylabel('Y');
title('Map');
% 开始迭代
for iter = 1:iter_max
% 初始化蚂蚁位置
ant_pos = repmat(start_point, ant_count, 1);
ant_path = zeros(ant_count, d);
ant_dist = zeros(ant_count, 1);
% 每只蚂蚁搜索路径
for k = 1:ant_count
for i = 1:d
% 计算可行方向的概率
p = tau(ant_pos(k, i) + 1, :) .^ alpha .* eta .^ beta;
p(ant_pos(k, i)) = 0;
p(end) = 0;
p = p / sum(p);
% 选择下一个位置
next_pos = find(rand < cumsum(p), 1) - 1;
if next_pos == d
break;
end
% 更新路径和距离
ant_path(k, i) = next_pos;
ant_dist(k) = ant_dist(k) + norm(ant_pos(k, :) - ant_path(k, :));
ant_pos(k, i + 1) = next_pos;
end
end
% 计算每只蚂蚁的路径信息素贡献
delta_tau = zeros(d + 1, d + 1);
for k = 1:ant_count
if any(ant_path(k, :) == obstacle)
continue;
end
for i = 1:d
delta_tau(ant_pos(k, i) + 1, ant_path(k, i) + 1) = delta_tau(ant_pos(k, i) + 1, ant_path(k, i) + 1) + Q / ant_dist(k);
end
delta_tau(end, ant_pos(k, end) + 1) = delta_tau(end, ant_pos(k, end) + 1) + Q / ant_dist(k);
end
% 更新信息素
tau = (1 - rho) * tau + delta_tau;
% 更新启发函数
eta = 1 / (norm(end_point - start_point) + iter * ant_dist(1));
% 绘制路径
if mod(iter, 10) == 0
figure(1);
plot(start_point(1), start_point(2), 'ro', 'MarkerSize', 10);
plot(end_point(1), end_point(2), 'ro', 'MarkerSize', 10);
plot(obstacle(1), obstacle(2), 's', 'MarkerSize', 10, 'MarkerFaceColor', 'r');
text(start_point(1) + 0.5, start_point(2) + 0.5, 'Start');
text(end_point(1) + 0.5, end_point(2) + 0.5, 'End');
text(obstacle(1) + 0.5, obstacle(2) + 0.5, 'Obstacle');
xlabel('X');
ylabel('Y');
title(['Iteration ' num2str(iter)]);
for k = 1:ant_count
if any(ant_path(k, :) == obstacle)
continue;
end
line([start_point(1), ant_pos(k, 1)], [start_point(2), ant_pos(k, 2)], 'Color', [0.5, 0.5, 0.5], 'LineWidth', 1);
for i = 1:d-1
line([ant_pos(k, i), ant_path(k, i)], [ant_pos(k, i+1), ant_path(k, i+1)], 'Color', [0.5, 0.5, 0.5], 'LineWidth', 1);
end
line([ant_path(k, d), end_point(1)], [ant_path(k, d+1), end_point(2)], 'Color', [0.5, 0.5, 0.5], 'LineWidth', 1);
end
end
% 判断是否到达终点
if any(ant_path(:, end) == end_point)
disp(['Iteration ' num2str(iter) ': Reach the end point!']);
break;
end
end
```
希望能对你有所帮助!
蚁群算法避障python
蚁群算法是一种基于模拟自然界蚂蚁觅食行为的优化算法,常用于解决路径规划和避障等问题。在Python中,可以使用numpy库进行矩阵运算、matplotlib库进行可视化等。
以下是一个简单的蚁群算法避障的Python实现示例:
```python
import numpy as np
import matplotlib.pyplot as plt
# 定义地图和障碍物
map_size = (100, 100)
obstacles = [(40, 50), (60, 70), (80, 30)]
# 定义蚂蚁的数量和最大迭代次数
ant_count = 50
max_iter = 100
# 初始化蚂蚁位置和速度
ants_pos = np.random.rand(ant_count, 2) * np.array(map_size)
ants_vel = np.random.rand(ant_count, 2) - 0.5
# 迭代更新蚂蚁位置和速度
for iter in range(max_iter):
for i in range(ant_count):
# 计算蚂蚁的下一步位置
next_pos = ants_pos[i] + ants_vel[i]
# 判断下一步位置是否与障碍物相交
if any(np.linalg.norm(next_pos - obs) < 2 for obs in obstacles):
# 避障处理,随机调整速度方向
ants_vel[i] = np.random.rand(2) - 0.5
else:
# 更新蚂蚁位置
ants_pos[i] = next_pos
# 绘制蚂蚁移动路径和障碍物
plt.figure()
plt.scatter(ants_pos[:, 0], ants_pos[:, 1], color='red', s=10)
plt.scatter([obs[0] for obs in obstacles], [obs[1] for obs in obstacles], color='black', s=100)
plt.xlim(0, map_size[0])
plt.ylim(0, map_size[1])
plt.show()
```
在这个示例中,首先定义了地图的大小和障碍物的位置。然后使用numpy库生成了随机的蚂蚁位置和速度。接着进行迭代更新,对于每只蚂蚁,计算它的下一步位置并判断是否与障碍物相交,如果相交则随机调整速度方向,否则更新蚂蚁位置。最后使用matplotlib库进行可视化,将蚂蚁的移动路径和障碍物绘制在地图上。
这只是一个简单的蚁群算法避障示例,实际应用中可能需要更复杂的处理和优化。希望对你有所帮助!
相关推荐
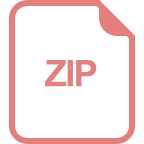
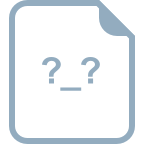
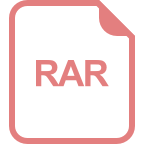












