利用随机库make_classification写原生Python编写感知器算法
时间: 2023-08-01 21:06:06 浏览: 114
好的,我来解答您的问题。
感知器算法是一种二分类算法,它的目的是找到一个线性超平面来将两类数据分开。在这里,我将使用 Python 的随机库 make_classification 生成一些随机数据,然后使用原生 Python 编写感知器算法。
首先,我们需要导入必要的库:
```python
import numpy as np
from sklearn.datasets import make_classification
```
然后,我们可以使用 make_classification 生成一些随机数据:
```python
X, y = make_classification(n_samples=100, n_features=2, n_informative=2, n_redundant=0, n_clusters_per_class=1, random_state=42)
```
这会生成 100 个样本,每个样本有 2 个特征,其中 2 个特征是有用的,没有冗余特征,每个类别有 1 个簇,并且使用随机种子 42。
接下来,我们可以定义感知器算法:
```python
class Perceptron:
def __init__(self, learning_rate=0.1, n_iters=1000):
self.lr = learning_rate
self.n_iters = n_iters
self.activation_func = self._unit_step_func
self.weights = None
self.bias = None
def fit(self, X, y):
n_samples, n_features = X.shape
# init parameters
self.weights = np.zeros(n_features)
self.bias = 0
y_ = np.array([1 if i > 0 else 0 for i in y])
# gradient descent
for _ in range(self.n_iters):
for idx, x_i in enumerate(X):
linear_output = np.dot(x_i, self.weights) + self.bias
y_predicted = self.activation_func(linear_output)
update = self.lr * (y_[idx] - y_predicted)
self.weights += update * x_i
self.bias += update
def predict(self, X):
linear_output = np.dot(X, self.weights) + self.bias
y_predicted = self.activation_func(linear_output)
return y_predicted
def _unit_step_func(self, x):
return np.where(x>=0, 1, 0)
```
该算法使用梯度下降法来更新权重和偏置。在初始化时,我们定义了学习率和迭代次数,激活函数使用了阶跃函数,权重和偏置初始化为零。在拟合过程中,我们对每个样本进行迭代,根据预测值和真实值之间的误差更新权重和偏置。在预测过程中,我们计算线性输出,然后使用激活函数将其转换为二进制输出。
最后,我们可以使用以下代码来训练和测试我们的模型:
```python
perceptron = Perceptron(learning_rate=0.1, n_iters=1000)
perceptron.fit(X, y)
y_pred = perceptron.predict(X)
print(y_pred)
```
这将打印出模型的预测结果。
希望这个例子对您有所帮助!
阅读全文
相关推荐











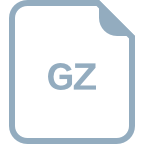


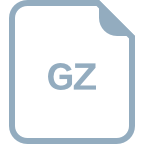

