python实现使用ransac算法对surf算法进行优化
时间: 2023-07-08 12:23:33 浏览: 166
RANSAC(Random Sample Consensus)是一种用于估计数学模型参数的迭代方法,它可以从一组包含异常值的观测数据中估计出最佳的数学模型参数。SURF(Speeded Up Robust Features)是一种计算机视觉算法,可以用于图像特征提取和匹配。
要使用RANSAC算法对SURF算法进行优化,可以按照以下步骤进行:
1. 提取图像的SURF特征点。
2. 使用RANSAC算法对提取的SURF特征点进行筛选,去除掉可能是异常值的点。
3. 根据剩余的特征点来计算图像之间的变换矩阵,例如仿射变换矩阵或透视变换矩阵。
4. 使用变换矩阵将两幅图像进行配准,以实现图像对齐和拼接。
在Python中,可以使用OpenCV库来实现SURF算法和RANSAC算法。具体实现步骤如下:
1. 导入必要的库:
```python
import cv2
import numpy as np
from sklearn.linear_model import RANSACRegressor
```
2. 读取两幅图像并提取SURF特征点:
```python
img1 = cv2.imread('image1.jpg')
img2 = cv2.imread('image2.jpg')
surf = cv2.xfeatures2d.SURF_create()
kp1, des1 = surf.detectAndCompute(img1, None)
kp2, des2 = surf.detectAndCompute(img2, None)
```
3. 使用RANSAC算法进行特征点筛选:
```python
model, inliers = None, None
ransac = RANSACRegressor()
for i in range(100):
# 随机选取四个特征点
sample = np.random.choice(len(kp1), 4, replace=False)
# 计算仿射变换矩阵
M = cv2.getAffineTransform(
np.float32([kp1[s].pt for s in sample]),
np.float32([kp2[s].pt for s in sample]))
# 计算变换后的特征点坐标
dst = cv2.transform(
np.float32([kp1[s].pt for s in range(len(kp1))]).reshape(-1, 1, 2), M)
# 计算特征点坐标之间的残差
diff = np.linalg.norm(dst.reshape(-1, 2) - np.float32([kp2[s].pt for s in range(len(kp2))]), axis=1)
# 计算符合阈值的内点
inliers_cur = np.where(diff < 5)[0]
# 更新最优模型和内点集合
if model is None or len(inliers_cur) > len(inliers):
model, inliers = M, inliers_cur
```
4. 根据内点集合计算变换矩阵并进行图像配准:
```python
M, _ = cv2.findHomography(
np.float32([kp1[s].pt for s in inliers]).reshape(-1, 1, 2),
np.float32([kp2[s].pt for s in inliers]).reshape(-1, 1, 2),
cv2.RANSAC, 5.0)
result = cv2.warpPerspective(img1, M, (img1.shape[1] + img2.shape[1], img1.shape[0]))
result[0:img2.shape[0], 0:img2.shape[1]] = img2
cv2.imwrite('result.jpg', result)
```
注意,以上代码仅供参考,具体实现可能需要根据实际情况进行调整。
阅读全文
相关推荐
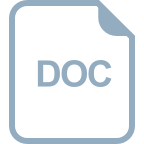
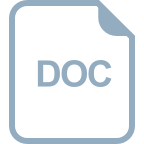
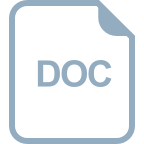



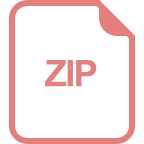


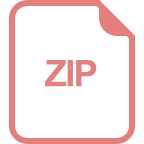







