1、.,*,+,?, \d,\w,\s,\b分别是什么? py
时间: 2023-12-20 20:04:53 浏览: 12
以上都是 Python 中的正则表达式元字符,具体含义如下:
- "." 代表任意单个字符。
- "*" 代表匹配前面的字符 0 次或多次。
- " " 代表空格字符。
- "?" 代表匹配前面的字符 0 次或 1 次。
- "\d" 代表任意一位数字。
- "\w" 代表任意一个字符或数字或下划线。
- "\s" 代表任意一个空白字符。
- "\b" 代表单词边界。
相关问题
Traceback (most recent call last): File "D:\anaconda3\envs\ml-lab\lib\site-packages\IPython\core\interactiveshell.py", line 3505, in run_code exec(code_obj, self.user_global_ns, self.user_ns) File "<ipython-input-2-6c57772f5021>", line 1, in <module> runfile('D:/ml-lab/.idea/not1.py', wdir='D:/ml-lab/.idea') File "D:\PyCharm 2021.3.3\plugins\python\helpers\pydev\_pydev_bundle\pydev_umd.py", line 198, in runfile pydev_imports.execfile(filename, global_vars, local_vars) # execute the script File "D:\PyCharm 2021.3.3\plugins\python\helpers\pydev\_pydev_imps\_pydev_execfile.py", line 18, in execfile exec(compile(contents+"\n", file, 'exec'), glob, loc) File "D:/ml-lab/.idea/not1.py", line 86, in <module> svm.fit(train_X, train_y) File "D:/ml-lab/.idea/not1.py", line 32, in fit self.E = self._E(self.X, self.y, self.b, self.alpha) File "D:/ml-lab/.idea/not1.py", line 83, in _E return np.dot(X, self.w) + b - y为啥报错
这是一个 Python 的 Traceback 错误信息,可以看到报错信息中包含了文件路径和行号。根据报错信息可以看出是在 `not1.py` 文件中的第 86 行出现了错误,具体是在 `svm.fit(train_X, train_y)` 这一行。在 `fit` 函数中调用了 `_E` 函数计算误差,而在 `_E` 函数中的第 83 行出现了错误。具体错误信息是 `return np.dot(X, self.w) + b - y`,这可能是由于某些变量的维度不匹配导致的。需要检查一下相关变量的维度是否正确以及是否有缺失值等问题。
yolov7 common.py 源码
以下是 YOLOv7 的 common.py 源码:
```python
import math
import torch.nn.functional as F
import torch.nn as nn
import torch
def make_divisible(x, divisor):
# Returns x evenly divisible by divisor
return math.ceil(x / divisor) * divisor
def autopad(k, p=None): # kernel, padding
# Pad to 'same'
if p is None:
p = k // 2 if isinstance(k, int) else [x // 2 for x in k]
return p
class Conv(nn.Module):
# Standard convolution
def __init__(self, c1, c2, k=1, s=1, p=None, g=1, act=True):
super(Conv, self).__init__()
self.conv = nn.Conv2d(c1, c2, k, stride=s, padding=autopad(k, p), groups=g, bias=False)
self.bn = nn.BatchNorm2d(c2)
self.act = nn.Hardswish() if act else nn.Identity()
def forward(self, x):
return self.act(self.bn(self.conv(x)))
class Bottleneck(nn.Module):
# Standard bottleneck
def __init__(self, c1, c2, shortcut=True, g=1, e=0.5):
super(Bottleneck, self).__init__()
c_ = int(c2 * e) # hidden channels
self.cv1 = Conv(c1, c_, 1, 1)
self.cv2 = Conv(c_, c2, 3, 1, g=g)
self.add = shortcut and c1 == c2
self.identity = nn.Identity() if self.add else None
def forward(self, x):
return self.identity(x) + self.cv2(self.cv1(x))
class SPP(nn.Module):
# Spatial pyramid pooling layer used in YOLOv3-SPP
def __init__(self, c1, c2, k=(5, 9, 13)):
super(SPP, self).__init__()
c_ = c1 // 2 # hidden channels
self.cv1 = Conv(c1, c_, 1, 1)
self.cv2 = Conv(c_ * (len(k) + 1), c2, 1, 1)
self.m = nn.ModuleList([nn.MaxPool2d(kernel_size=x, stride=1, padding=x // 2) for x in k])
def forward(self, x):
x = self.cv1(x)
return self.cv2(torch.cat([x] + [m(x) for m in self.m], 1))
class DWConv(nn.Module):
# Depthwise convolution
def __init__(self, c1, c2, k=1, s=1, p=None):
super(DWConv, self).__init__()
self.conv = nn.Conv2d(c1, c1, k, stride=s, padding=autopad(k, p), groups=c1, bias=False)
self.bn = nn.BatchNorm2d(c1)
self.act = nn.Hardswish()
self.project = nn.Conv2d(c1, c2, 1, bias=False)
self.bn2 = nn.BatchNorm2d(c2)
self.act2 = nn.Hardswish()
def forward(self, x):
return self.act2(self.bn2(self.project(self.act(self.bn(self.conv(x))))))
class Focus(nn.Module):
# Focus wh information into c-space
def __init__(self, c1, c2, k=1, s=1, p=None, g=1, act=True):
super(Focus, self).__init__()
self.conv = Conv(c1 * 4, c2, k, s, p, g, act)
def forward(self, x):
# x(b,c,w,h) -> y(b,4c,w/2,h/2)
return self.conv(torch.cat([x[..., ::2, ::2], x[..., 1::2, ::2], x[..., ::2, 1::2], x[..., 1::2, 1::2]], 1))
class Concat(nn.Module):
# Concatenate a list of tensors along dimension
def __init__(self, dimension=1):
super(Concat, self).__init__()
self.d = dimension
def forward(self, x):
return torch.cat(x, self.d)
class Detect(nn.Module):
# Detect layer
def __init__(self, nc, anchors):
super(Detect, self).__init__()
self.nc = nc # number of classes
self.no = nc + 5 # number of outputs per anchor
self.na = len(anchors) # number of anchors
self.anchors = torch.tensor(anchors).float().view(self.na, -1)
self.anchors /= self.anchors.sum(1).view(self.na, 1) # normalized anchors
self.register_buffer("anchor_grid", self.anchors.clone().view(1, -1, 1, 1))
self.m = nn.Conv2d(self.no * self.na, self.no * self.na, 1) # prediction conv
def forward(self, x):
# x(bs,255,h,w) -> p(bs,3,85,h,w)
bs, _, ny, nx = x.shape
device, dtype = x.device, x.dtype
stride = self.anchor_grid.device / torch.tensor([nx, ny])[None, :, None, None].to(device)
grid = torch.meshgrid([torch.arange(ny), torch.arange(nx)])
y = torch.stack(grid, 2).to(device).float()
x = (x.sigmoid() * 2. - 0.5) * stride # x(?,255,?,?) --sig--> x(?,255,?,?) --*2-0.5--> x(?,255,?,?) --*stride--> x(?,255,?,?)
y = (y + 0.5) * stride # y(?,2,?,?) --+0.5--> y(?,2,?,?) --*stride--> y(?,2,?,?)
xy = torch.stack([x, y], 2).view(bs, 2, self.na * ny * nx).permute(0, 2, 1).contiguous().view(bs, self.na * ny * nx, 2)
x = self.m(x.flatten(2).permute(0, 2, 1)).view(bs, self.na, self.no, ny, nx).permute(0, 1, 3, 4, 2).contiguous()
# x(bs,na,ny,nx,na) --view--> x(bs,na,ny,nx,no) --permute--> x(bs,na,ny,nx,no)
if not self.training:
x[..., 4:] = x[..., 4:].sigmoid()
return x
else: # train
return x, xy, self.anchor_grid.repeat(bs, 1, ny, nx)
class Model(nn.Module):
# YOLOv7 model https://github.com/WongKinYiu/yolov7
def __init__(self, nc=80, anchors=((10, 13), (16, 30), (33, 23), (30, 61), (62, 45), (59, 119), (116, 90), (156, 198), (373, 326)),
ch=[256, 512, 1024, 2048], depth=0.33):
super(Model, self).__init__()
assert depth in [0.33, 0.67, 1.0]
self.depth = depth # model depth multiplier
self.grid = [torch.zeros(1)] * 5 # init grid
self.stride = torch.tensor([8., 16., 32., 64., 128.])
self.create_backbone(ch)
self.create_neck()
self.create_head(nc, anchors)
def forward(self, x):
z = []
for i in range(5):
x = self.backbone[i](x)
z.append(x)
x = self.neck(z)
return self.head(x)
def create_backbone(self, ch):
# darknet backbone
self.backbone = nn.ModuleList([Focus(3, ch[0], 3),
Conv(ch[0], ch[1], 3, 2),
Bottleneck(ch[1], ch[2]),
Conv(ch[2], ch[3], 3, 2),
Bottleneck(ch[3], ch[4]),
Conv(ch[4], ch[5], 3, 2),
SPP(ch[5], ch[5]),
Bottleneck(ch[5], ch[6]),
Conv(ch[6], ch[7], 1)])
c2 = make_divisible(ch[7] * self.depth) # ch_last
self.backbone.append(Bottleneck(ch[7], c2, False))
self.out_channels = [c2, ch[4], ch[2], ch[0]]
def create_neck(self):
# FPN-like attentional output
self.neck = nn.Sequential(
Concat(),
Conv(self.out_channels[0], self.out_channels[0], 1),
DWConv(self.out_channels[0], self.out_channels[1], 3, s=2),
DWConv(self.out_channels[1], self.out_channels[2], 3, s=2),
DWConv(self.out_channels[2], self.out_channels[3], 3, s=2),
SPP(self.out_channels[3], self.out_channels[3]),
DWConv(self.out_channels[3], self.out_channels[3], 3, dilation=3),
DWConv(self.out_channels[3], self.out_channels[3], 3, dilation=3),
DWConv(self.out_channels[3], self.out_channels[3], 3, dilation=3),
)
def create_head(self, nc, anchors):
# detection head
self.head = nn.Sequential(
DWConv(self.out_channels[3], self.out_channels[3], 3, dilation=3),
DWConv(self.out_channels[3], self.out_channels[3], 3, dilation=3),
DWConv(self.out_channels[3], self.out_channels[3], 3, dilation=3),
Concat(),
Conv(self.out_channels[3] * 4, self.out_channels[3], 1),
nn.Conv2d(self.out_channels[3], len(anchors) * (nc + 5), 1, bias=True),
Detect(nc, anchors))
def attempt_load(weights, map_location=None, inplace=True):
# Loads an ensemble of models weights=[a,b,c] or a single model weights=[a] or weights=a
if isinstance(weights, (list, tuple)):
# Load a list of models (ensemble)
ensemble = nn.ModuleList()
for w in weights:
model = Model()
model.to(next(w.parameters()).device)
try:
ckpt = torch.load(w, map_location=map_location) # load
state_dict = ckpt['model'].float().state_dict() # to FP32
state_dict = {k: v for k, v in state_dict.items() if model.state_dict()[k].shape == v.shape} # filter
model.load_state_dict(state_dict, strict=False) # load
print(f"Transferred {len(state_dict)} from {w}")
except:
print(f"Error loading {w}")
ensemble.append(model.eval())
return ensemble
else:
# Load a single model
model = Model()
model.to(next(weights.parameters()).device)
try:
ckpt = torch.load(weights, map_location=map_location) # load
state_dict = ckpt['model'].float().state_dict() # to FP32
state_dict = {k: v for k, v in state_dict.items() if model.state_dict()[k].shape == v.shape} # filter
model.load_state_dict(state_dict, strict=False) # load
print(f"Transferred {len(state_dict)} from {weights}")
except:
print(f"Error loading {weights}")
return model.eval()
```
相关推荐
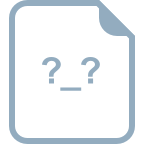
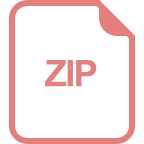
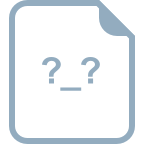













