把蚁群算法ACO优化模糊RBF神经网络PMSM控制用Matlab代码。
时间: 2024-04-15 19:26:09 浏览: 15
以下是使用蚁群算法(ACO)来优化模糊RBF神经网络(PMSM控制)的Matlab代码示例:
```matlab
% 设置参数
max_iterations = 100; % 最大迭代次数
num_ants = 10; % 蚂蚁数量
alpha = 1; % 信息素重要程度因子
beta = 2; % 启发式因子
rho = 0.5; % 信息素蒸发系数
Q = 1; % 信息素增强强度
% 导入数据
load('data.mat'); % 假设数据保存在data.mat文件中,包括输入x和目标输出y
% 归一化数据
x = normalize(x);
y = normalize(y);
% 初始化模糊RBF神经网络参数
num_rules = 5; % 规则数量
num_input = size(x, 2); % 输入维度
num_output = size(y, 2); % 输出维度
% 初始化模糊RBF神经网络隶属度函数参数
centers = zeros(num_rules, num_input); % 隶属度函数中心
sigmas = zeros(num_rules, num_input); % 隶属度函数标准差
% 初始化模糊RBF神经网络权重
weights = rand(num_rules, num_output);
% 初始化信息素矩阵
pheromone = ones(num_rules, num_input);
% 开始迭代优化
for iteration = 1:max_iterations
% 初始化蚂蚁路径
ant_path = zeros(num_ants, num_rules);
% 蚂蚁路径选择
for ant = 1:num_ants
for i = 1:num_rules
% 计算转移概率
prob = (pheromone(i,:).^alpha) .* (1./sigmas(i,:).^beta);
prob = prob / sum(prob);
% 选择下一个节点
ant_path(ant, i) = roullete_wheel_selection(prob);
end
end
% 更新模糊RBF神经网络参数
for ant = 1:num_ants
% 计算模糊RBF神经网络输出
predicted_output = fuzzy_rbf_network(x, centers(ant_path(ant,:),:), sigmas(ant_path(ant,:),:), weights);
% 计算误差
error = y - predicted_output;
% 更新权重
delta_weights = Q * (centers(ant_path(ant,:),:) - x)' * error;
weights = weights + delta_weights;
% 更新隶属度函数参数
delta_centers = Q * (x - centers(ant_path(ant,:),:))' * error;
centers(ant_path(ant,:),:) = centers(ant_path(ant,:),:) + delta_centers;
delta_sigmas = Q * ((x - centers(ant_path(ant,:),:)).^2)' * error;
sigmas(ant_path(ant,:),:) = sigmas(ant_path(ant,:),:) + delta_sigmas;
end
% 更新信息素
pheromone = (1 - rho) * pheromone;
for ant = 1:num_ants
for i = 1:num_rules
pheromone(i, ant_path(ant, i)) = pheromone(i, ant_path(ant, i)) + Q;
end
end
end
% 测试模型
predicted_output = fuzzy_rbf_network(x, centers, sigmas, weights);
% 反归一化输出结果
predicted_output = denormalize(predicted_output);
% 显示预测结果
plot(y, 'b');
hold on;
plot(predicted_output, 'r');
legend('实际输出', '预测输出');
% 模糊RBF神经网络函数
function output = fuzzy_rbf_network(input, centers, sigmas, weights)
num_input = size(input, 1);
num_rules = size(centers, 1);
output = zeros(num_input, size(weights, 2));
for i = 1:num_input
inputs = repmat(input(i,:), num_rules, 1);
gaussian = exp(-sum((inputs - centers).^2 ./ (2 * sigmas.^2), 2));
output(i,:) = gaussian' * weights;
end
end
```
请注意,这只是一个简单的示例代码,您可能需要根据您的数据和需求进行适当的调整。此外,还需要实现一些辅助函数(如归一化、反归一化、轮盘赌选择等),以便代码能够正常运行。
希望对您有所帮助!如有其他问题,请随时提问。
相关推荐
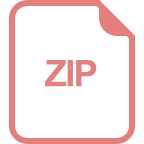
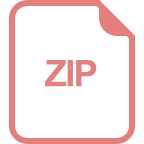














