人工神经网络算法代码
时间: 2024-10-08 21:08:15 浏览: 11
人工神经网络(Artificial Neural Networks, 简称ANN)是一种计算模型,它受生物神经系统启发,由大量相互连接的节点(称为神经元)组成,用于解决各种复杂的机器学习任务,如分类、回归和聚类等。编写ANN的代码通常涉及到深度学习库,比如Python中的TensorFlow或PyTorch。
以下是一个简单的使用Python和Keras库搭建一个多层感知机(Multilayer Perceptron, MLP)的示例:
```python
import tensorflow as tf
from tensorflow.keras.models import Sequential
from tensorflow.keras.layers import Dense
# 创建一个Sequential模型
model = Sequential()
# 添加输入层,隐藏层和输出层
model.add(Dense(units=64, activation='relu', input_dim=input_shape)) # 隐藏层
model.add(Dense(units=32, activation='relu')) # 可能还有更多的隐藏层
model.add(Dense(units=num_classes, activation='softmax')) # 输出层(对于分类问题)
# 编译模型,指定损失函数、优化器和评估指标
model.compile(optimizer='adam', loss='categorical_crossentropy', metrics=['accuracy'])
# 使用训练数据拟合模型
model.fit(X_train, y_train, epochs=epochs, batch_size=batch_size, validation_data=(X_test, y_test))
# 保存模型
model.save('my_model.h5')
```
在这个例子中,`input_shape`是输入数据的维度,`num_classes`是类别数,`epochs`是训练轮次,`batch_size`是批次大小。每个`Dense`层代表神经网络中的一个权重矩阵,激活函数决定了神经元如何处理输入。
相关推荐
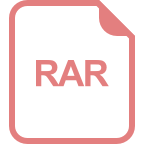
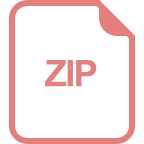
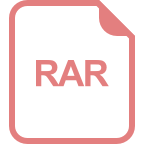
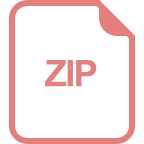
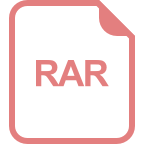
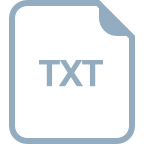
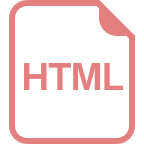
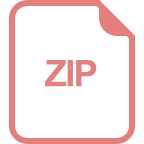
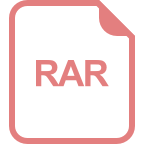
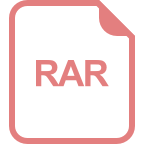
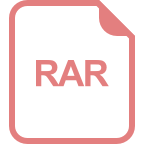
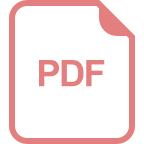
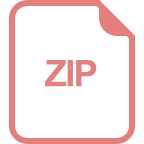
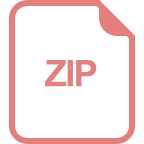
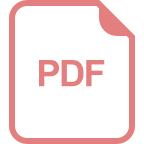
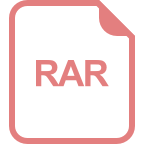
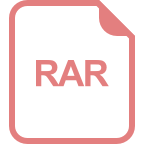
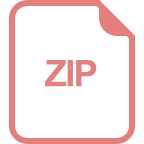