分布式模型预测控制 代码
时间: 2024-03-10 07:41:37 浏览: 37
分布式模型预测控制是指将机器学习或深度学习模型的预测任务分布到多个计算节点上进行并行处理,以提高预测性能和吞吐量的技术。下面是一个简单的分布式模型预测控制的代码示例:
```python
# 导入必要的库
import tensorflow as tf
from tensorflow.keras.models import load_model
from tensorflow.keras.preprocessing import image
import numpy as np
# 加载模型
model = load_model('path_to_model.h5')
# 定义预测函数
def predict(image_path):
img = image.load_img(image_path, target_size=(224, 224))
img = image.img_to_array(img)
img = np.expand_dims(img, axis=0)
img = img / 255.0 # 数据预处理,归一化到0-1之间
# 使用分布式模型预测控制进行预测
with tf.device('/job:worker/task:0'): # 指定计算节点
preds = model.predict(img)
return preds
# 调用预测函数进行预测
image_path = 'path_to_image.jpg'
predictions = predict(image_path)
# 打印预测结果
print(predictions)
```
以上代码示例使用TensorFlow库加载已训练好的模型,并定义了一个预测函数`predict`,该函数接受一个图像路径作为输入,并返回模型对该图像的预测结果。在预测函数中,使用`tf.device`指定了计算节点,可以根据实际情况进行调整。
相关推荐
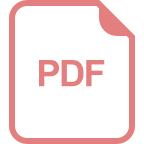
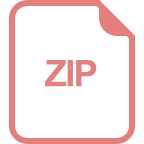














