calibration plot
时间: 2023-08-27 19:02:25 浏览: 334
校准绘图(calibration plot)是一种用于评估预测模型准确性的图表。它是一个散点图,其中横轴表示预测的概率或得分,纵轴表示实际事件发生的概率。校准绘图通过比较预测概率与实际概率之间的关系来评估模型的准确性和校准性。
在理想情况下,如果模型是完全校准的,预测概率应该与实际概率完全吻合。这意味着在校准绘图中,所有的数据点应该落在理想的对角线上。然而,实际应用中,很少有模型能够完美校准。
校准绘图的作用是帮助我们识别模型的偏差和过度自信问题。如果模型在某个特定预测概率范围内过于自信,那么校准绘图中的数据点会偏离对角线。例如,如果模型总是高估事件发生的概率,那么数据点将会位于对角线的上方。相反,如果模型总是低估事件发生的概率,数据点将会位于对角线的下方。
校准绘图还可以用来选择最佳的预测模型。当比较多个模型时,我们可以通过观察校准绘图并选择那些离对角线最近的模型作为最佳的预测模型。这通常意味着该模型能够更好地预测实际事件的发生。
总之,校准绘图是一种评估预测模型准确性和校准性的强大工具。通过观察校准绘图,我们可以识别模型的偏差和过度自信问题,并选择最佳的预测模型。
相关问题
Calibration plot代码
以下是一个简单的 Python 代码示例,用于绘制校准曲线:
```python
import matplotlib.pyplot as plt
from sklearn.calibration import calibration_curve
from sklearn.datasets import make_classification
from sklearn.ensemble import RandomForestClassifier
from sklearn.model_selection import train_test_split
# 生成一个二分类数据集
X, y = make_classification(n_samples=1000, n_features=10, n_classes=2, random_state=42)
# 将数据集分为训练集和测试集
X_train, X_test, y_train, y_test = train_test_split(X, y, test_size=0.2, random_state=42)
# 训练一个随机森林分类器
clf = RandomForestClassifier(n_estimators=100, random_state=42)
clf.fit(X_train, y_train)
# 绘制校准曲线
prob_pos = clf.predict_proba(X_test)[:, 1]
fraction_of_positives, mean_predicted_value = calibration_curve(y_test, prob_pos, n_bins=10)
plt.plot(mean_predicted_value, fraction_of_positives, 's-', label='Random Forest')
plt.plot([0, 1], [0, 1], 'k--', label='Perfectly calibrated')
plt.xlabel('Mean predicted value')
plt.ylabel('Fraction of positives')
plt.legend()
plt.show()
```
这个代码使用 `sklearn.calibration.calibration_curve` 函数计算校准曲线的数据点,然后使用 `matplotlib` 库绘制曲线。
R语言决策树calibration plot代码
以下是R语言决策树calibration plot的代码:
```R
library(rpart.plot)
library(ggplot2)
# 构建决策树模型
model <- rpart(Species ~ ., data = iris)
# 预测结果
pred <- predict(model, type = "prob")
# 将预测结果与实际结果合并
result <- data.frame(pred, iris$Species)
# 计算每个预测概率区间的实际结果比例
calib <- result %>%
group_by(Species) %>%
mutate(bin = cut(pred[, Species], breaks = seq(0, 1, 0.1), include.lowest = TRUE)) %>%
group_by(bin, .add = TRUE) %>%
summarise(n = n()) %>%
mutate(prop = n / sum(n))
# 绘制calibration plot
ggplot(calib, aes(x = as.numeric(bin), y = prop, fill = Species)) +
geom_bar(stat = "identity", position = "dodge") +
scale_fill_manual(values = c("#00AFBB", "#E7B800", "#FC4E07")) +
labs(x = "Predicted probability", y = "Actual proportion", fill = "Species") +
theme_minimal()
```
希望对你有帮助!
阅读全文
相关推荐
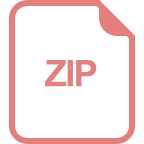
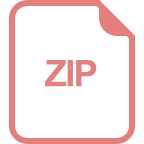
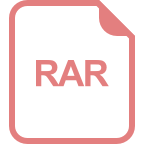
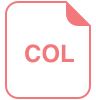




