overlaps在深度学习中是什么意思
时间: 2023-11-13 14:05:12 浏览: 72
在深度学习中,"overlaps" 可以指代两个不同的概念。
1. 感受野重叠(Receptive Field Overlaps):这是指在卷积神经网络 (CNN) 中,每个神经元对输入图像的感受野(局部输入区域)的重叠程度。感受野是指一个神经元对应输入图像上的区域,它决定了神经元所能感知到的信息范围。当感受野之间存在重叠时,神经元可以共享相同的信息,从而提取更丰富和全局性的特征。
2. 重叠层(Overlapping Layers):在一些神经网络架构中,特征图(Feature Map)之间可以通过重叠的方式进行连接。这种重叠的层可以在空间上共享信息,并提供更多的上下文相关性。这种结构常见于一些分割或定位任务中,例如目标检测或语义分割。
需要根据上下文来确定在特定情况下 "overlaps" 所指的具体含义。
相关问题
oracle中的overlaps为什么不推荐使用?
Oracle中的OVERLAPS是一个日期函数,它用于比较两个时间段是否有重叠。虽然这个函数可以很方便地进行时间段重叠的判断,但是由于它的执行效率较低,因此Oracle官方并不推荐使用它。
主要原因有以下几点:
1. OVERLAPS函数在执行时需要进行大量的时间段比较计算,这会导致执行效率较低,尤其是在处理大量数据时。
2. OVERLAPS函数只能比较两个时间段是否重叠,不能对多个时间段进行比较,这在实际应用中会受到限制。
3. 在Oracle中,使用其他函数如INTERVAL、TIMESTAMP等也可以进行时间段的比较,而且执行效率更高,因此推荐使用这些函数代替OVERLAPS。
综上所述,虽然OVERLAPS函数可以方便地进行时间段重叠的判断,但由于其执行效率较低,Oracle官方并不推荐使用它。
mysql OVERLAPS
MySQL的OVERLAPS函数用于判断两个时间段是否有重叠。它接受四个参数,分别是开始时间和结束时间的两个时间段。如果两个时间段有重叠,则返回1,否则返回0。
以下是一个示例:
```mysql
SELECT * FROM table_name
WHERE (start_time1, end_time1) OVERLAPS (start_time2, end_time2);
```
在上面的示例中,`table_name`是你要查询的表名,`start_time1`和`end_time1`是第一个时间段的开始时间和结束时间,`start_time2`和`end_time2`是第二个时间段的开始时间和结束时间。
请注意,OVERLAPS函数只能用于比较时间段,而不能用于比较日期或时间。
相关推荐
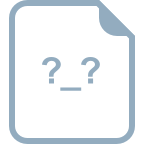












