我想把new_loss = np.mean((single_image_objective_vectors - centroid) ** 2)这个代码np.mean函数如何修改成tensor的,并且将这些tensor放在cuda上运行
时间: 2024-04-30 10:18:05 浏览: 4
要将`np.mean()`函数修改为tensor函数,可以使用`torch.mean()`函数。同时,如果要将tensor放在cuda上运行,可以使用`.to()`方法。具体代码如下:
```python
import torch
# 将numpy数组转换为tensor
single_image_objective_vectors = torch.tensor(single_image_objective_vectors)
centroid = torch.tensor(centroid)
# 将tensor放在cuda上运行
single_image_objective_vectors = single_image_objective_vectors.to('cuda')
centroid = centroid.to('cuda')
# 计算新的损失
new_loss = torch.mean((single_image_objective_vectors - centroid) ** 2)
```
需要注意的是,以上代码需要先确保有可用的cuda设备。可以使用以下代码检查:
```python
import torch
if torch.cuda.is_available():
print('cuda is available!')
else:
print('cuda is not available!')
```
如果输出`cuda is not available!`,则说明当前系统没有可用的cuda设备。
相关问题
null_space = np.linalg.solve(A, np.zeros(A.shape[0]))、
对不起,我之前的回答有误,`np.linalg.solve()`函数是用于求解形如Ax=b的线性方程组的解,而不是求解Ax=0的零空间。对于求解Ax=0的零空间,可以使用`np.linalg.eig()`函数来进行特征值分解。
以下是使用NumPy库中的函数求解矩阵A的零空间的示例代码:
```python
import numpy as np
A = np.array([[1, -2, 2, -1],
[2, -4, 8, 0],
[-2, 4, -2, 3],
[3, -6, -6, 0]])
# 求解A的特征值和特征向量
eigenvalues, eigenvectors = np.linalg.eig(A)
# 找到特征值为0的索引
null_space_index = np.where(np.isclose(eigenvalues, 0))
# 提取特征向量对应的索引
null_space_vectors = eigenvectors[:, null_space_index]
print(null_space_vectors)
```
这样,变量`null_space_vectors`将包含矩阵A的零空间的特征向量。输出结果将是一个二维数组,每一列表示一个特征向量。
s_dsb=mt.*cos(2*pi*fc*t);
根据提供的引用内容,s_dsb=mt.*cos(2*pi*fc*t)是一个调幅信号的表式,其中mt是调制信号,fc是载波频率,t是时间。这个达式表示了调制信号mt通过乘以一个载波信号cos(2*pi*fc*t)来调幅。调幅是一种将调制信号的幅变化嵌入到载波信号中的调制方式。
范例:<<引用:T2F.m function [f,sf]= T2F(t,st) %利用FFT计算信号的频谱并与信号的真实频谱的抽样比较。 %脚本文件T2F.m定义了函数T2F,计算信号的傅立叶变换。 %This is a function using the FFT function to calculate a signal Fourier %Translation %Input is the time and the signal vectors,the length of time must greater %than 2 %Output is the frequency and the signal spectrum dt = t(2)-t(1); T=t(end); df = 1/T; N = length(st); f=-N/2*df : df : N/2*df-df; sf = fft(st); sf = T/N*fftshift(sf); 。引用:lpf.m function [t,st]=lpf(f,sf,B) %This function filter an input data using a lowpass filter %Inputs: f: frequency samples % sf: input data spectrum samples % B: lowpass bandwidth with a rectangle lowpass %Outputs: t: time samples % st: output data time samples df = f(2)-f(1); T = 1/df; hf = zeros(1,length(f));%全零矩阵 bf = [-floor( B/df ): floor( B/df )] + floor( length(f)/2 ); hf(bf)=1; yf=hf.*sf; [t,st]=F2T(f,yf); st = real(st);。
请回答我或者给我介绍或演示一下: s_dsb=mt.*cos(2*pi*fc*t)的含义是什么?
s_dsb=mt.*cos(2*pi*fc*t)表示调幅信号的表达式,其中mt是调制信号,fc是载波频率,t是时间。这个表达式表示了调制信号mt通过乘以一个载波信号cos(2*pi*fc*t)来进行调幅。调幅是一种将调制信号的幅度变化嵌入到载波信号中的调制方式。
相关推荐
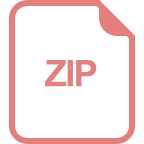













