用python实现以下功能,用imblearn包进行数据均衡化,包括多列的输入和一列输出参数,最后均衡化后的数据与最多的数据量保持一致
时间: 2024-02-13 18:00:50 浏览: 78
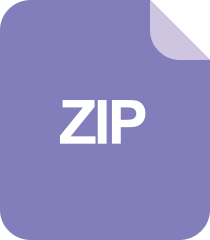
利用python进行数据分析
好的,根据你的要求,我们可以使用 `imblearn` 中的 `RandomOverSampler` 或者 `SMOTE` 进行数据均衡化,然后将样本数量调整为最多的样本数量。
以下是示例代码:
```python
from imblearn.over_sampling import RandomOverSampler
from sklearn.datasets import make_classification
from sklearn.model_selection import train_test_split
import pandas as pd
# 生成样本数据,X为多列输入,y为一列输出
X, y = make_classification(n_classes=2, class_sep=2,
weights=[0.1, 0.9], n_informative=3,
n_redundant=1, flip_y=0, n_features=20,
n_clusters_per_class=1, n_samples=1000,
random_state=10)
# 划分训练集和测试集
X_train, X_test, y_train, y_test = train_test_split(X, y, test_size=0.3, random_state=42)
# 进行样本均衡化
ros = RandomOverSampler(random_state=0)
X_train_resampled, y_train_resampled = ros.fit_resample(X_train, y_train)
# 调整样本数量
max_count = max(pd.Series(y_train_resampled).value_counts())
X_train_resampled = pd.DataFrame(X_train_resampled)
y_train_resampled = pd.Series(y_train_resampled)
X_train_resampled = pd.concat([X_train_resampled[y_train_resampled==0].sample(n=max_count, replace=True, random_state=0),
X_train_resampled[y_train_resampled==1].sample(n=max_count, replace=True, random_state=0)])
y_train_resampled = pd.concat([pd.Series([0]*max_count),
pd.Series([1]*max_count)])
# 输出样本均衡化前后的样本数量
print("原始数据集中1和0的数量:")
print(y_train.value_counts())
print("均衡化后数据集中1和0的数量:")
print(y_train_resampled.value_counts())
```
其中 `make_classification` 函数可以生成随机的分类数据,用于模拟真实数据。`train_test_split` 函数可以划分训练集和测试集。`RandomOverSampler` 可以进行随机过采样,使得样本数量均衡化。最后,我们可以将样本数量调整为最多的样本数量,即先将不足最多样本数量的样本进行有放回的抽样,使得样本数量达到最多的样本数量,然后将两个类别的样本合并。最后,我们可以输出样本均衡化前后的样本数量,以验证均衡化效果。
希望我的回答能够对你有所帮助。
阅读全文
相关推荐
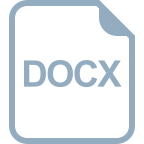


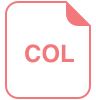
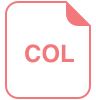
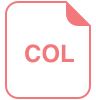
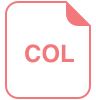
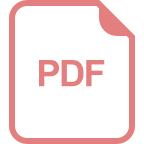
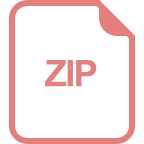
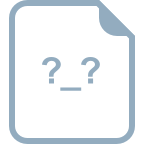
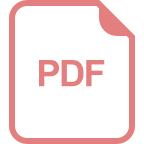
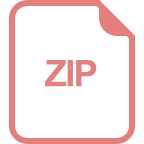
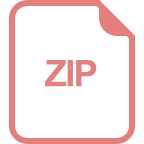
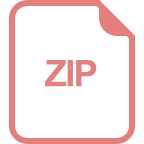