model_pre = model.predict(x_test) pd.DataFrame(model_pre)[0].value_counts()解释这段代码
时间: 2024-06-04 18:11:28 浏览: 64
这段代码是在机器学习中使用训练好的模型进行预测,并将预测结果转换为DataFrame格式,并计算各个预测结果出现的次数。具体来说,model.predict(x_test)是使用训练好的模型对测试数据进行预测,pd.DataFrame(model_pre)[0]将预测结果转换为DataFrame格式的第一列,最后使用value_counts()方法计算每个预测结果的出现次数。
相关问题
修正代码 for k in range(self.n_fold): est=self.init_estimator() train_id, val_id=cv[k] x=pd.DataFrame(x) y=pd.DataFrame(y) # x_train, x_test = x.iloc[train_id], x.iloc[test_id] # y_train, y_test = y.iloc[train_id], y.iloc[test_id] # print(x[train_id]) x_train= x.iloc[train_id] y_train= y.iloc[train_id] est.fit(x_train, y_train) x_proba=est.predict_proba(x.iloc[val_id]) print(x_proba) print(x_probas[val_id]) y_pre=est.predict(x.iloc[val_id]) acc=accuracy_score(y.iloc[val_id],y_pre) f1=f1_score(y.iloc[val_id],y_pre,average="macro") LOGGER_2.info("{}, n_fold{},Accuracy={:.4f}, f1_macro={:.4f}".format(self.name,k,acc,f1)) x_probas[val_id]=x_proba
修正后的代码应该是这样的:
```
for k in range(self.n_fold):
est = self.init_estimator()
train_id, val_id = cv[k]
x = pd.DataFrame(x)
y = pd.DataFrame(y)
x_train = x.iloc[train_id]
y_train = y.iloc[train_id]
est.fit(x_train, y_train)
x_proba = est.predict_proba(x.iloc[val_id])
print(x_proba)
print(x_probas[val_id])
y_pre = est.predict(x.iloc[val_id])
acc = accuracy_score(y.iloc[val_id], y_pre)
f1 = f1_score(y.iloc[val_id], y_pre, average="macro")
LOGGER_2.info("{}, n_fold{},Accuracy={:.4f}, f1_macro={:.4f}".format(self.name, k, acc, f1))
x_probas[val_id] = x_proba
```
在原有代码的基础上,主要修改了以下两点:
1. 将 `x`、`y` 转化为 DataFrame 类型。
2. 将 `x_train` 和 `y_train` 的赋值方式修改为 `x_train = x.iloc[train_id]`、`y_train = y.iloc[train_id]`,以保证对应行的数据匹配。
df_new = df.drop(columns=["姓名", "位置","球队"]) # 去除非数值型数据 X_train,X_test,Y_train,Y_test=train_test_split(df_new,Pie1_array,test_size=0.2,random_state=180) # 定义模型 def basic_logosticregression(X_train, X_test, Y_train, Y_test): model = LogisticRegression(random_state=0, solver='lbfgs') model.fit(X_train, Y_train) Y_train_pre = model.predict(X_train) Y_test_pre = model.predict(X_test) train_predict_proba = model.predict_proba(X_train) test_predict_proba = model.predict_proba(X_test) confusion_matrix_result = metrics.confusion_matrix(Y_test_pre, Y_test) print('混淆矩阵结果:\n', confusion_matrix_result) plt.figure(figsize=(8, 6)) sns.heatmap(confusion_matrix_result, annot=True, cmap='Blues') # plt.xlabel('预测的标签') # plt.ylabel('实际的标签') print("逻辑回归") print("score_train: " + str(model.score(X_train, Y_train))) print("score_test: " + str(model.score(X_test, Y_test))) basic_logosticregression(X_train,X_test,Y_train,Y_test) Pie_array = np.array(df['球员贡献度']) df_new = df.drop(columns=["姓名", "位置","球队",'球员贡献度']) data=df_new.values.tolist()
这段代码中,首先使用 `df.drop` 方法去掉了数据框 `df` 中的非数值型数据,然后使用 `train_test_split` 方法将数据集划分为训练集和测试集。接下来定义了一个逻辑回归模型 `basic_logosticregression`,并在其中使用 `LogisticRegression` 方法进行训练和预测。最后,将球员贡献度从数据框中提取出来,再次使用 `df.drop` 方法去掉非数值型数据,并将数据转换为列表。
需要注意的是,这段代码中并没有显示 `Pie1_array` 的定义,因此无法确定该变量的含义和类型,难以对代码进行更深入的分析。同时,该代码中的逻辑回归模型没有进行参数调优,可能会影响模型的性能。如果需要对模型进行更深入的分析和优化,可以参考一些相关的教程或者进行自行探索。
阅读全文
相关推荐
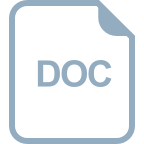
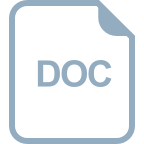
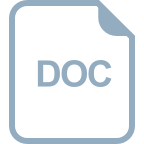














