Read the stock price data of Alphabet Inc. (GOOG) for the time period between April 01, 2020 and October 01, 2020 in the file 'alphabet.csv' into a Pandas DataFrame. You can download it from Blackboard in session 12. (1) Convert the “Date” column into Datetime format and assign it as the index column. And output the DataFram containing data for month x. (2) Calculate the net increase of stock price for each day and append them as a new column “Net Increase” to the DataFrame using the closing price minus the opening price. And output the DataFram containing data for month x. (3) Find the day that has the highest opening price, and calculate the mean of closing price for all days. NOTE:You should add the following code to your program to ensure that all columns are displayed when printing.Otherwise, the system will consider it as incorrect. pd.set_option('display.max_columns',1000) pd.set_option('display.width', 1000) pd.set_option('display.max_colwidth',1000)
时间: 2024-01-31 14:02:34 浏览: 25
以下是对应的Python代码实现:
```python
import pandas as pd
# 读取csv文件
df = pd.read_csv('alphabet.csv')
# 将“Date”列转换成Datetime格式,并将其设置为索引列
df['Date'] = pd.to_datetime(df['Date'])
df.set_index('Date', inplace=True)
# 输出2020年4月的数据
print("Data for April 2020:")
print(df['2020-04'])
# 计算每日的净涨幅,并将其添加为新列“Net Increase”
df['Net Increase'] = df['Close'] - df['Open']
# 输出2020年5月的数据
print("Data for May 2020:")
print(df['2020-05'])
# 找到开盘价最高的那天,并计算所有交易日的收盘价平均值
max_open = df['Open'].idxmax()
mean_close = df['Close'].mean()
print(f"The day with the highest opening price was {max_open.date()}, with an opening price of {df.loc[max_open]['Open']:.2f}.")
print(f"The mean closing price for all days was {mean_close:.2f}.")
# 设置pandas的显示选项,确保所有列都能显示出来
pd.set_option('display.max_columns', 1000)
pd.set_option('display.width', 1000)
pd.set_option('display.max_colwidth', 1000)
```
在这个示例中,我们使用 Pandas 的 `read_csv` 函数读取了 `alphabet.csv` 文件中的数据,并将其转换成了 DataFrame 对象。接着,我们将“Date”列转换成了 Datetime 格式,并将其设置为索引列。然后,我们按照题目要求,输出了2020年4月和5月的数据,并计算了每日的净涨幅,并将其添加为新列“Net Increase”。最后,我们找到了开盘价最高的那天,并计算了所有交易日的收盘价平均值,并输出了结果。同时,我们也设置了 Pandas 的显示选项,确保所有列都能显示出来。
相关推荐
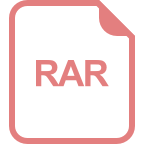
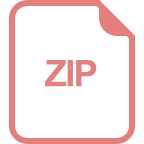
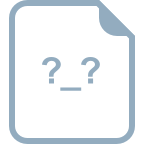
















