代码生成LSTM检测网络异常数据
时间: 2023-05-25 12:06:47 浏览: 119
由于 LSTM 网络对时间序列数据具有很好的建模能力,因此可以将其用于检测异常数据。以下是使用 TensorFlow 2.0 代码生成 LSTM 检测网络异常数据的示例代码:
```python
# 导入必要的库
import tensorflow as tf
from tensorflow.keras.layers import Input, LSTM, Dense, Dropout
from tensorflow.keras.models import Model
# 定义检测网络异常数据的 LSTM 模型
def anomaly_detection_model(input_shape):
inputs = Input(shape=input_shape)
x = LSTM(units=64, return_sequences=True)(inputs)
x = Dropout(0.2)(x)
x = LSTM(units=64, return_sequences=False)(x)
x = Dropout(0.2)(x)
outputs = Dense(units=input_shape[1])(x)
model = Model(inputs=inputs, outputs=outputs)
return model
# 训练和测试 LSTM 模型
def train(model, train_data, validation_data, epochs, batch_size):
model.compile(optimizer='adam', loss='mse')
history = model.fit(train_data, train_data,
validation_data=(validation_data, validation_data),
epochs=epochs, batch_size=batch_size)
return history
# 使用训练好的 LSTM 模型检测数据是否异常
def detect_anomalies(model, data, threshold):
predictions = model.predict(data)
errors = tf.reduce_mean(tf.square(data - predictions), axis=1)
return errors > threshold
```
该代码生成一个包含两个 LSTM 层和一个全连接层的模型,用于检测时间序列数据中的异常。模型使用均方误差作为损失函数,在训练和测试期间都使用相同的数据进行训练。训练完毕后,可以使用 `detect_anomalies` 函数来检查输入数据是否存在异常。该函数将计算预测值与实际值之间的平均误差,并根据指定的阈值确定输入数据是否为异常。
阅读全文
相关推荐
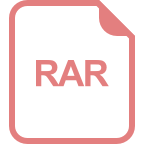
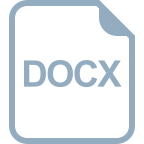
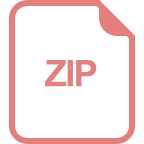
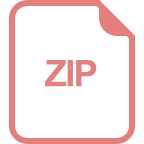
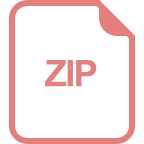
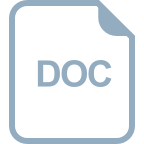
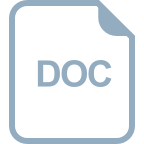
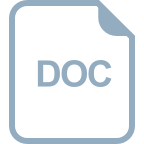
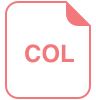
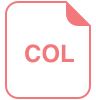
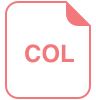
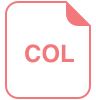
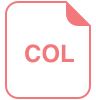
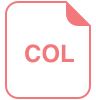
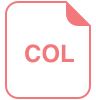
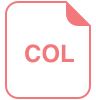
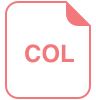