用python写一个随机森林模型
时间: 2024-04-21 21:07:21 浏览: 26
由于随机森林模型需要用到多个决策树,因此我们需要先实现决策树。
以下是一个简单的决策树实现。
```python
class DecisionTree:
def __init__(self, max_depth=None):
self.max_depth = max_depth
def fit(self, X, y):
self.n_features_ = X.shape[1]
self.tree_ = self._grow_tree(X, y)
def predict(self, X):
return [self._predict(inputs) for inputs in X]
def _best_split(self, X, y):
m = y.size
if m <= 1:
return None, None
num_parent = [np.sum(y == c) for c in range(self.n_classes_)]
best_gini = 1.0 - sum((n / m) ** 2 for n in num_parent)
best_idx, best_thr = None, None
for idx in range(self.n_features_):
thresholds, classes = zip(*sorted(zip(X[:, idx], y)))
num_left = [0] * self.n_classes_
num_right = num_parent.copy()
for i in range(1, m):
c = classes[i - 1]
num_left[c] += 1
num_right[c] -= 1
gini_left = 1.0 - sum((num_left[x] / i) ** 2 for x in range(self.n_classes_))
gini_right = 1.0 - sum((num_right[x] / (m - i)) ** 2 for x in range(self.n_classes_))
gini = (i * gini_left + (m - i) * gini_right) / m
if thresholds[i] == thresholds[i - 1]:
continue
if gini < best_gini:
best_gini = gini
best_idx = idx
best_thr = (thresholds[i] + thresholds[i - 1]) / 2
return best_idx, best_thr
def _grow_tree(self, X, y, depth=0):
num_samples_per_class = [np.sum(y == i) for i in range(self.n_classes_)]
predicted_class = np.argmax(num_samples_per_class)
node = Node(predicted_class=predicted_class)
if depth < self.max_depth:
idx, thr = self._best_split(X, y)
if idx is not None:
indices_left = X[:, idx] < thr
X_left, y_left = X[indices_left], y[indices_left]
X_right, y_right = X[~indices_left], y[~indices_left]
node.feature_index = idx
node.threshold = thr
node.left = self._grow_tree(X_left, y_left, depth + 1)
node.right = self._grow_tree(X_right, y_right, depth + 1)
return node
def _predict(self, inputs):
node = self.tree_
while node.left:
if inputs[node.feature_index] < node.threshold:
node = node.left
else:
node = node.right
return node.predicted_class
```
接下来是随机森林模型的实现。
```python
class RandomForest:
def __init__(self, n_trees, max_depth=None, max_samples=None, max_features=None):
self.n_trees = n_trees
self.max_depth = max_depth
self.max_samples = max_samples
self.max_features = max_features
def fit(self, X, y):
self.trees = []
n_samples = X.shape[0]
n_features = X.shape[1]
max_features = self.max_features or n_features
for i in range(self.n_trees):
tree = DecisionTree(max_depth=self.max_depth)
if self.max_samples:
indices = np.random.choice(n_samples, self.max_samples, replace=True)
else:
indices = np.arange(n_samples)
if self.max_features:
features = np.random.choice(n_features, self.max_features, replace=False)
else:
features = np.arange(n_features)
tree.n_classes_ = self.n_classes_
tree.n_features_ = max_features
tree.feature_indices_ = features
tree.fit(X[indices][:, features], y[indices])
self.trees.append(tree)
def predict(self, X):
y_preds = np.array([tree.predict(X[:, tree.feature_indices_]) for tree in self.trees]).T
return [np.bincount(y).argmax() for y in y_preds]
```
这样我们就实现了一个简单的随机森林模型。
相关推荐
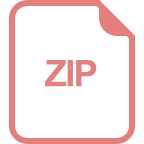
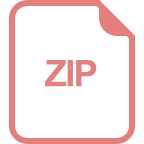
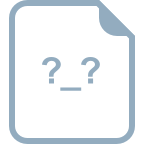
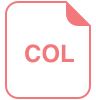
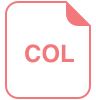
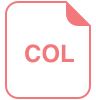
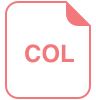
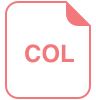









