float char_to_float(char* data_byte),这个函数 如何调用》
时间: 2024-05-09 19:21:53 浏览: 14
这个函数可以通过以下方式调用:
```
char* data = "3.14";
float result = char_to_float(data);
```
注意传入的参数必须是一个指向字符数组的指针,指向的字符数组中必须是表示浮点数的字符串,如"3.14"。函数会将字符串转换为对应的float类型返回。
相关问题
oled怎么显示浮点小数_LCD浮点数显示函数的探讨
关于 OLED 显示浮点数,一般可以通过将浮点数转换为字符数组,然后将字符数组显示在 OLED 屏幕上来实现。具体实现方法可以参考下面的代码:
```C
#include <stdio.h>
#include <stdlib.h>
#include <string.h>
// OLED 显示
void OLED_ShowString(uint8_t x, uint8_t y, uint8_t *chr, uint8_t Char_Size)
{
uint8_t c = 0,i = 0,j = 0;
switch(Char_Size)
{
case 16:
{
while (chr[j]!='\0')
{
c = chr[j]-32;
if(x>112){x=0;y+=16;}
OLED_Set_Pos(x,y);
for(i=0;i<8;i++)
OLED_WR_Byte(F8X16[c*16+i],OLED_DATA);
OLED_Set_Pos(x,y+8);
for(i=0;i<8;i++)
OLED_WR_Byte(F8X16[c*16+i+8],OLED_DATA);
x+=8;
j++;
}
}
break;
case 12:
{
while (chr[j]!='\0')
{
c = chr[j]-32;
if(x>116){x=0;y+=12;}
OLED_Set_Pos(x,y);
for(i=0;i<6;i++)
OLED_WR_Byte(F6x8[c][i],OLED_DATA);
x+=6;
j++;
}
}
break;
}
}
// 浮点数转换为字符数组
void FloatToStr(float f, char *str)
{
int i = 0;
int integer = (int)f; // 取整数部分
float decimal = f - integer; // 取小数部分
if (integer < 0) // 处理负数
{
integer = -integer;
decimal = -decimal;
str[i++] = '-';
}
// 转换整数部分
if (integer == 0)
{
str[i++] = '0';
}
else
{
while (integer > 0)
{
str[i++] = integer % 10 + '0';
integer /= 10;
}
}
// 转换小数部分
str[i++] = '.';
decimal = decimal * 1000000;
integer = (int)decimal;
if (integer == 0)
{
str[i++] = '0';
}
else
{
while (integer > 0)
{
str[i++] = integer % 10 + '0';
integer /= 10;
}
}
str[i] = '\0';
// 反转字符数组
for (int j = 0; j < i / 2; j++)
{
char temp = str[j];
str[j] = str[i - j - 1];
str[i - j - 1] = temp;
}
}
// 测试函数
void test()
{
float f = 3.1415926;
char str[20];
FloatToStr(f, str);
OLED_ShowString(0, 0, (uint8_t *)str, 16);
}
int main()
{
test();
return 0;
}
```
在上面的代码中,`FloatToStr()` 函数将浮点数转换为字符数组,`OLED_ShowString()` 函数将字符数组显示在 OLED 屏幕上。具体的显示方法可以根据自己的 OLED 屏幕型号和驱动芯片进行调整。
怎么把这段c#转换为在c++中的dll函数实现,并在最后把float[] c返回给到c#,注意c++中的session和和c#的inferencesession不同 InferenceSession session = new InferenceSession(modelPath); Mat src_f = copy_from_mat(img); var wl = m_width * m_height; VectorOfMat temp = new VectorOfMat(); CvInvoke.Split(src_f, temp); float[] typedArr = new float[3 * m_width * m_height]; unsafe { fixed (float* target = typedArr) { for (int i = 0; i < temp.Size; i++) { var rawDataPointer = temp[i].DataPointer; Buffer.MemoryCopy((byte*)rawDataPointer, (byte*)target + (i * wl * sizeof(float)), wl * sizeof(float), wl * sizeof(float)); } } } var input = new DenseTensor<float>(typedArr, new[] { 1, 3, m_height, m_width }); var inputs = new List<NamedOnnxValue> { NamedOnnxValue.CreateFromTensor("images", input) }; var results = session.Run(inputs).ToArray(); //var ooo = results[0].AsTensor<float>(); float[] c = results[0].AsTensor<float>().ToArray();
你想要将上述C#代码转换为C++的DLL函数,以便在C#中使用。下面是一个可能的实现:
首先,你需要包含以下头文件:
```cpp
#include <vector>
#include <memory>
#include <string>
#include "onnxruntime_cxx_api.h"
#include "opencv2/opencv.hpp"
```
接下来,你可以编写一个函数,该函数将接收模型路径和OpenCV图像作为输入,并返回结果数组。请注意,为了将结果传递回C#,你需要使用指针并将其包装在一个结构体中。
```cpp
typedef struct {
float* data;
int size;
} FloatArray;
FloatArray run_inference(const char* model_path, cv::Mat img) {
// Load the model
Ort::SessionOptions session_options;
Ort::Env env(ORT_LOGGING_LEVEL_WARNING, "onnx");
Ort::Session session(env, model_path, session_options);
// Convert the image to a float array
int m_width = img.cols;
int m_height = img.rows;
cv::Mat src_f;
img.convertTo(src_f, CV_32FC3);
std::vector<cv::Mat> temp;
cv::split(src_f, temp);
float* typedArr = new float[3 * m_width * m_height];
for (int i = 0; i < temp.size(); i++) {
std::memcpy(typedArr + (i * m_width * m_height), temp[i].data, m_width * m_height * sizeof(float));
}
// Create the input tensor
Ort::MemoryInfo memory_info = Ort::MemoryInfo::CreateCpu(OrtArenaAllocator, OrtMemTypeDefault);
std::vector<int64_t> input_shape = { 1, 3, m_height, m_width };
Ort::Value input_tensor = Ort::Value::CreateTensor<float>(memory_info, typedArr, 3 * m_width * m_height, input_shape.data(), input_shape.size());
// Run the inference
const char* input_names[] = { "images" };
Ort::RunOptions run_options;
std::vector<Ort::Value> inputs = { input_tensor };
std::vector<const char*> output_names = { "output" };
std::vector<Ort::Value> output_tensors = session.Run(run_options, input_names, inputs.data(), inputs.size(), output_names.data(), output_names.size());
// Convert the output tensor to a float array
float* output_data = output_tensors[0].GetTensorMutableData<float>();
int output_size = output_tensors[0].GetTensorTypeAndShapeInfo().GetElementCount();
FloatArray result = { output_data, output_size };
// Clean up
delete[] typedArr;
return result;
}
```
最后,你需要将该函数导出到DLL。这可以通过以下方式完成:
```cpp
extern "C" __declspec(dllexport) FloatArray __cdecl run_inference(const char* model_path, cv::Mat img);
```
现在你可以在C#中调用此函数,并将结果转换为float数组。
相关推荐
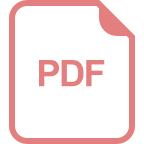












