trainer = torch.optim.SGD([ {"params":net[0].weight,'weight_decay': wd}, {"params":net[0].bias}], lr=lr)
时间: 2024-05-31 21:10:28 浏览: 165
这是一个基于随机梯度下降法(SGD)的优化器,用于更新神经网络中的参数。在这里,使用了一个字典来指定不同的参数及其对应的超参数。具体来说,其中包含两个参数:网络的权重和偏置,分别对应 net[0].weight 和 net[0].bias。同时,还设置了权重衰减参数 weight_decay 和学习率 lr。其中,权重衰减是一种正则化方法,通过惩罚较大的权重值来避免过拟合;学习率则控制了每次更新参数时的步长,过大或过小的学习率都可能导致训练不稳定或效果不佳。
相关问题
def train(net, train_iter, val_iter, num_epochs, lr, wd, devices, lr_period, lr_decay): global val_acc, metric trainer = torch.optim.SGD(net.parameters(), lr=lr, momentum=0.9, weight_decay=wd) scheduler = torch.optim.lr_scheduler.StepLR(trainer, lr_period, lr_decay) num_batches, timer = len(train_iter), d2l.Timer() train_loss, train_accs, val_accs = [], [], [] for epoch in range(num_epochs): net.train() metric = d2l.Accumulator(3) for i, (features, labels) in enumerate(train_iter): trainer.zero_grad() features, labels = features.to(devices[0]), labels.to(devices[0]) l, acc = d2l.train_batch_ch13(net, features, labels, loss, trainer, devices) metric.add(l, acc, labels.shape[0]) train_loss.append(metric[0] / metric[2]) train_accs.append(metric[1] / metric[2]) if val_iter is not None: val_acc = d2l.evaluate_accuracy_gpu(net, val_iter) val_accs.append(val_acc) d2l.plot(range(1, epoch + 2), [train_loss, train_accs, val_accs], xlabel='epoch', legend=['train loss', 'train acc', 'val acc'], figsize=(8, 6)) scheduler.step() RuntimeError: Input type (torch.cuda.FloatTensor) and weight type (torch.FloatTensor) should be the same
这段代码出现了一个 RuntimeError,提示输入类型(torch.cuda.FloatTensor)和权重类型(torch.FloatTensor)应该是相同的。这说明在使用 GPU 训练时,输入数据和权重数据类型不一致。可以在数据加载时将数据类型转换为 cuda 类型,或者将模型参数转换为 CPU 类型。
def train(net, train_iter, val_iter, num_epochs, lr, wd, devices, lr_period, lr_decay): global val_acc, metric trainer = torch.optim.SGD(net.patameters(), lr=lr, momentum=0.9, weight_decay=wd) scheduler = torch.optim.lr_scheduler.StepLR(trainer, lr_period, lr_decay) num_batches, timer = len(train_iter), d2l.Timer() legend = ['train loss', 'train acc'] # 画出来的图的线条标签 if val_iter is not None: legend.append('valid acc') animator = d2l.Animator(xlabel='epoch', xlim=[1, num_epochs], legend=legend) net = nn.DataParallel(net, device_ids=0).to(device) for epoch in range(num_epochs): # 开始训练 net.train() metric = d2l.Accumulator(3) for i, (features, labels) in enumerate(train_iter): timer.start() l, acc = d2l.train_batch_ch13(net, features, labels, loss, trainer, devices) metric.add(l, acc, labels.shape[0]) timer.stop() if (i + 1) % (num_batches // 5) == 0 or i == num_batches - 1: animator.add(epoch + (i + 1) / num_batches, (metric[0] / metric[2], metric[1] / metric[2], None)) if val_iter is not None: val_acc = d2l.evaluate_accuracy_gpu(net, val_iter) animator.add(epoch + 1, (None, None, val_acc)) scheduler.step() measures = (f'train loss {metric[0] / metric[2]:.3f},'f'train acc {metric[1] / metric[2]:.3f}') if val_iter is not None: measures += f', val acc {val_acc :.3f}'检查并优化
在这段代码中,有一个拼写错误,应该是 `net.parameters()` 而不是 `net.patameters()`,所以修改一下即可:
```python
def train(net, train_iter, val_iter, num_epochs, lr, wd, devices, lr_period, lr_decay):
global val_acc, metric
trainer = torch.optim.SGD(net.parameters(), lr=lr, momentum=0.9, weight_decay=wd)
scheduler = torch.optim.lr_scheduler.StepLR(trainer, lr_period, lr_decay)
num_batches, timer = len(train_iter), d2l.Timer()
legend = ['train loss', 'train acc'] # 画出来的图的线条标签
if val_iter is not None:
legend.append('valid acc')
animator = d2l.Animator(xlabel='epoch', xlim=[1, num_epochs], legend=legend)
net = nn.DataParallel(net, device_ids=0).to(device)
for epoch in range(num_epochs):
# 开始训练
net.train()
metric = d2l.Accumulator(3)
for i, (features, labels) in enumerate(train_iter):
timer.start()
l, acc = d2l.train_batch_ch13(net, features, labels, loss, trainer, devices)
metric.add(l, acc, labels.shape[0])
timer.stop()
if (i + 1) % (num_batches // 5) == 0 or i == num_batches - 1:
animator.add(epoch + (i + 1) / num_batches, (metric[0] / metric[2], metric[1] / metric[2], None))
if val_iter is not None:
val_acc = d2l.evaluate_accuracy_gpu(net, val_iter)
animator.add(epoch + 1, (None, None, val_acc))
scheduler.step()
measures = (f'train loss {metric[0] / metric[2]:.3f},'
f'train acc {metric[1] / metric[2]:.3f}')
if val_iter is not None:
measures += f', val acc {val_acc :.3f}'
```
此外,您也需要确认您已经正确引入了相关的库,比如 `torch`、`nn`、`d2l` 等。
阅读全文
相关推荐


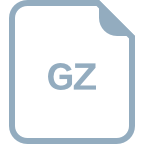













