用堆叠自编码器进行对指定文件夹内的4类彩色图像进行分类并可视化混淆矩阵python代码
时间: 2024-05-13 18:15:25 浏览: 136
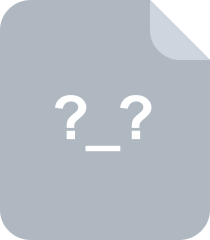
彩色图像分类.py
以下是用堆叠自编码器进行对指定文件夹内的4类彩色图像进行分类并可视化混淆矩阵的Python代码:
```python
import numpy as np
import matplotlib.pyplot as plt
import os
import cv2
from sklearn.model_selection import train_test_split
from sklearn.metrics import confusion_matrix
from keras.layers import Input, Dense, Flatten
from keras.models import Model
from keras.callbacks import EarlyStopping
# 定义输入图像尺寸
IMG_SIZE = 128
# 定义类别标签和对应的数字编码
CATEGORIES = ['cat', 'dog', 'bird', 'fish']
NUM_CATEGORIES = len(CATEGORIES)
LABELS = {i: label for i, label in enumerate(CATEGORIES)}
# 加载图像数据和类别标签
dataset = []
for category in CATEGORIES:
path = os.path.join('data', category)
label = CATEGORIES.index(category)
for img_file in os.listdir(path):
img_path = os.path.join(path, img_file)
img = cv2.imread(img_path)
img = cv2.resize(img, (IMG_SIZE, IMG_SIZE))
dataset.append([img, label])
# 将数据分为训练集和测试集
X = np.array([data[0] for data in dataset]).astype('float32') / 255
y = np.array([data[1] for data in dataset])
X_train, X_test, y_train, y_test = train_test_split(X, y, test_size=0.2, random_state=42)
# 定义自编码器模型
input_layer = Input(shape=(IMG_SIZE, IMG_SIZE, 3))
x = Flatten()(input_layer)
x = Dense(512, activation='relu')(x)
x = Dense(256, activation='relu')(x)
x = Dense(128, activation='relu')(x)
x = Dense(64, activation='relu')(x)
encoded = Dense(32, activation='relu')(x)
x = Dense(64, activation='relu')(encoded)
x = Dense(128, activation='relu')(x)
x = Dense(256, activation='relu')(x)
x = Dense(512, activation='relu')(x)
decoded = Dense(IMG_SIZE * IMG_SIZE * 3, activation='sigmoid')(x)
autoencoder = Model(input_layer, decoded)
# 定义编码器模型
encoder = Model(input_layer, encoded)
# 编译自编码器模型
autoencoder.compile(optimizer='adam', loss='binary_crossentropy')
# 训练自编码器模型
early_stop = EarlyStopping(monitor='val_loss', patience=5)
autoencoder.fit(X_train, X_train, epochs=50, batch_size=32, validation_split=0.2, callbacks=[early_stop])
# 提取特征向量
X_train_encoded = encoder.predict(X_train)
X_test_encoded = encoder.predict(X_test)
# 定义分类器模型
input_layer = Input(shape=(32,))
x = Dense(64, activation='relu')(input_layer)
x = Dense(32, activation='relu')(x)
output_layer = Dense(NUM_CATEGORIES, activation='softmax')(x)
classifier = Model(input_layer, output_layer)
# 编译分类器模型
classifier.compile(optimizer='adam', loss='sparse_categorical_crossentropy', metrics=['accuracy'])
# 训练分类器模型
early_stop = EarlyStopping(monitor='val_loss', patience=5)
classifier.fit(X_train_encoded, y_train, epochs=50, batch_size=32, validation_split=0.2, callbacks=[early_stop])
# 在测试集上进行预测并计算混淆矩阵
y_pred = classifier.predict(X_test_encoded)
y_pred = np.argmax(y_pred, axis=1)
conf_matrix = confusion_matrix(y_test, y_pred)
# 可视化混淆矩阵
plt.imshow(conf_matrix, cmap='Blues')
plt.title('Confusion Matrix')
plt.xticks(np.arange(NUM_CATEGORIES), CATEGORIES)
plt.yticks(np.arange(NUM_CATEGORIES), CATEGORIES)
plt.colorbar()
for i in range(NUM_CATEGORIES):
for j in range(NUM_CATEGORIES):
plt.text(j, i, conf_matrix[i, j], ha='center', va='center')
plt.xlabel('Predicted Label')
plt.ylabel('True Label')
plt.show()
```
该代码首先加载指定文件夹内的彩色图像数据和类别标签,并将其分为训练集和测试集。然后,定义一个堆叠自编码器模型,用于提取图像的特征向量。接着,定义一个分类器模型,用于对特征向量进行分类。最后,在测试集上进行预测,并计算混淆矩阵,最终可视化混淆矩阵。
阅读全文
相关推荐


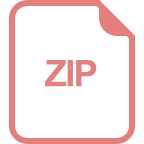
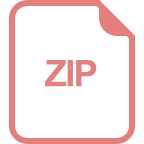
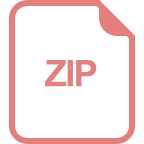
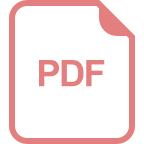
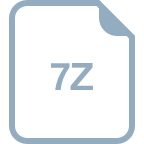
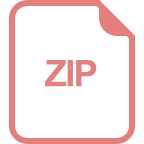
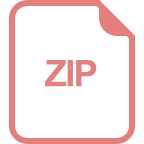
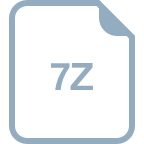
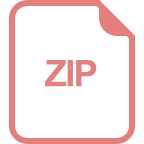
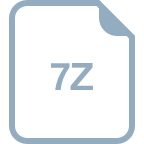
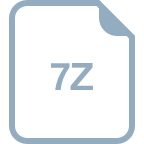
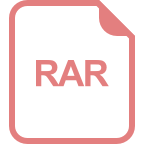
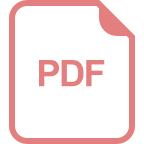
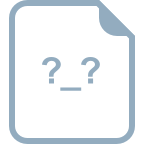
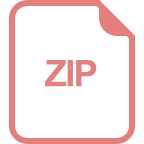