class Travels(object): @staticmethod def insert_db(item, pipeline_obj): sql = ''' INSERT travels(id,name,authorId,viewCount,likeCount, commentCount,publishTime,picUrl,authorName, authorHeadImg,authorIndentity,hasLike) VALUES('{}','{}','{}','{}','{}','{}','{}','{}','{}','{}','{}','{}') '''.format(item["id"], item["name"], item["authorId"], item["viewCount"], item["likeCount"], item["commentCount"], item["publishTime"], item["picUrl"], item["authorName"], item["authorHeadImg"], item["authorIndentity"], item["hasLike"]) pipeline_obj.mysql_conn.query(sql ) pipeline_obj.mysql_conn.commit() @staticmethod def insert_redis(item, pipeline_obj): detail_url = "http://www.tuniu.com/trips/" + str(item["id"]) pipeline_obj.redis_obj.rpush("tuniu:detail_urls", detail_url) class TravelsDetail(object): @staticmethod def insert_db(item, pipeline_obj): sql = ''' INSERT travels_detail(id,taglist,destination,price)VALUES('{}','{}','{}','{}') '''.format(item["id"], item["taglist"], item["destination"], item["price"]) pipeline_obj.mysql_conn.query(sql) pipeline_obj.mysql_conn.commit()将每一行代码都做解释
时间: 2024-04-25 16:26:00 浏览: 218
这段代码定义了两个类:Travels和TravelsDetail,分别用于将旅游信息和旅游详情信息存储到MySQL数据库中。在这两个类中,都定义了一个静态方法insert_db,用于将数据插入到数据库中。在Travels类中,还定义了一个静态方法insert_redis,用于将旅游详情页的URL存储到Redis缓存中。
具体实现中,insert_db方法使用了SQL的INSERT语句,将item中的数据插入到名为travels的表中。pipeline_obj是传入的Pipeline类实例,通过它可以访问数据库连接和执行SQL语句。插入完成后,需要执行commit方法提交更改。
在Travels类的insert_redis方法中,首先根据item中的id构造了旅游详情页的URL,然后通过Redis的rpush方法将URL存储到名为tuniu:detail_urls的列表中。pipeline_obj.redis_obj是传入的Redis连接实例,通过它可以访问Redis缓存。
在TravelsDetail类的insert_db方法中,也是使用SQL的INSERT语句将item中的数据插入到名为travels_detail的表中,然后执行commit方法提交更改。
相关问题
# See: https://doc.scrapy.org/en/latest/topics/item-pipeline.html import pymysql import redis from spiders.items import TravelsItem, TravelsDetailItemclass Travels(object): @staticmethod def insert_db(item, pipeline_obj): sql = ''' INSERT travels(id,name,authorId,viewCount,likeCount, commentCount,publishTime,picUrl,authorName, authorHeadImg,authorIndentity,hasLike) VALUES('{}','{}','{}','{}','{}','{}','{}','{}','{}','{}','{}','{}') '''.format(item["id"], item["name"], item["authorId"], item["viewCount"], item["likeCount"], item["commentCount"], item["publishTime"], item["picUrl"], item["authorName"], item["authorHeadImg"], item["authorIndentity"], item["hasLike"]) pipeline_obj.mysql_conn.query(sql ) pipeline_obj.mysql_conn.commit() @staticmethod def insert_redis(item, pipeline_obj): detail_url = "http://www.tuniu.com/trips/" + str(item["id"]) pipeline_obj.redis_obj.rpush("tuniu:detail_urls", detail_url) class TravelsDetail(object): @staticmethod def insert_db(item, pipeline_obj): sql = ''' INSERT travels_detail(id,taglist,destination,price)VALUES('{}','{}','{}','{}') '''.format(item["id"], item["taglist"], item["destination"], item["price"]) pipeline_obj.mysql_conn.query(sql) pipeline_obj.mysql_conn.commit()class TuniutripsPipeline(object): def open_spider(self, spider): self.mysql_conn = pymysql.connect(host="localhost", port=3306, user="root", passwd="123456", db="test") pool = redis.ConnectionPool(host="127.0.0.1", password='') self.redis_obj = redis.Redis(connection_pool=pool) def process_item(self, item, spider): if isinstance(item, TravelsItem): Travels.insert_db(item, self) Travels.insert_redis(item, self) elif isinstance(item, TravelsDetailItem): TravelsDetail.insert_db(item, self) def close_spider(self, spider): self.mysql_conn.close()将每一行代码都做解释
这段代码是一个Scrapy爬虫的Pipeline,用于将爬取的数据存储到MySQL数据库和Redis缓存中。在这段代码中,定义了两个类Travels和TravelsDetail,分别用于插入旅游信息和旅游详情信息到MySQL数据库中。在TuniutripsPipeline类中,定义了三个方法:open_spider用于建立数据库连接和Redis连接;process_item用于处理爬取的数据,根据数据类型调用Travels或TravelsDetail的插入方法;close_spider用于关闭数据库连接。具体实现中,使用了pymysql和redis-py库进行数据库连接和操作。
from pyspark.sql import SparkSession import matplotlib.pyplot as plt # 指定Mysql的配置 from pyspark.sql.types import StructField, StringType, StructType options = { "url": "jdbc:mysql://localhost:3306/test?useSSL=true", "driver": "com.mysql.jdbc.Driver", "dbtable": "(SELECT taglist from travels_detail where taglist !='None') t1", "user": "root", "password": "root" } spark = SparkSession.builder.getOrCreate() # 加载Mysql数据 data = spark.read.format("jdbc").options(**options).load() # 将每一行的taglist转为列表 def convert_to_list(line): tmp_list = line[0].replace("#", "").split(",") datas = [] for i in tmp_list: if len(i) > 0 and "牛" not in i: datas.append((i, 1)) return datas rdd = data.rdd.flatMap(lambda line: convert_to_list(line)).reduceByKey(lambda x, y: x + y) schemaString = "tag count" fields = [StructField(field_name, StringType(), True) for field_name in schemaString.split()] schema = StructType(fields) schema_data = spark.createDataFrame(rdd, schema).orderBy("count", ascending=False) # 将数据转换为Pandas数据帧 result_pdf = schema_data.limit(5).toPandas() # 设置matplotlib支持中文 plt.rcParams['font.family'] = ['sans-serif'] plt.rcParams['font.sans-serif'] = ['SimHei'] # colors=color, explode=explode, plt.pie(result_pdf["count"], labels=result_pdf["tag"], shadow=True, autopct='%1.1f%%') plt.legend() plt.show()逐行解释
这段代码是使用 PySpark 从 MySQL 数据库中读取数据,将每一行的 taglist 字段转换为列表,统计列表中每个元素出现的次数,然后将结果转换为 Pandas 数据帧,最后使用 matplotlib 绘制饼图。
这段代码的详细解释如下:
1. 导入需要的模块
```
from pyspark.sql import SparkSession
import matplotlib.pyplot as plt
from pyspark.sql.types import StructField, StringType, StructType
```
这里导入了 `SparkSession` 用于创建 Spark 应用程序,`matplotlib.pyplot` 用于绘制饼图,`StructField`、`StringType`、`StructType` 用于定义数据结构。
2. 配置 MySQL 连接参数
```
options = {
"url": "jdbc:mysql://localhost:3306/test?useSSL=true",
"driver": "com.mysql.jdbc.Driver",
"dbtable": "(SELECT taglist from travels_detail where taglist !='None') t1",
"user": "root",
"password": "root"
}
```
这里定义了连接 MySQL 数据库的参数,包括 URL、驱动程序、表名、用户名和密码。
3. 创建 SparkSession
```
spark = SparkSession.builder.getOrCreate()
```
这里创建了一个 SparkSession 对象,用于连接 Spark 集群。
4. 加载 MySQL 数据
```
data = spark.read.format("jdbc").options(**options).load()
```
这里使用 `SparkSession` 对象的 `read` 方法,读取 MySQL 数据库中的数据。
5. 将每一行的 taglist 转为列表
```
def convert_to_list(line):
tmp_list = line[0].replace("#", "").split(",")
datas = []
for i in tmp_list:
if len(i) > 0 and "牛" not in i:
datas.append((i, 1))
return datas
rdd = data.rdd.flatMap(lambda line: convert_to_list(line)).reduceByKey(lambda x, y: x + y)
```
这里定义了一个函数 `convert_to_list`,用于将每一行的 taglist 字段转换为列表,并且去掉其中的 "#" 和 "牛",统计列表中每个元素出现的次数。
然后使用 `flatMap` 将每一行的 taglist 转换为一个元素为 `(tag, 1)` 的列表,再使用 `reduceByKey` 统计每个 tag 出现的次数。
6. 将数据转换为 Pandas 数据帧
```
schemaString = "tag count"
fields = [StructField(field_name, StringType(), True) for field_name in schemaString.split()]
schema = StructType(fields)
schema_data = spark.createDataFrame(rdd, schema).orderBy("count", ascending=False)
result_pdf = schema_data.limit(5).toPandas()
```
这里先定义了一个字符串 `schemaString`,表示数据结构的字段名,然后使用 `StructField` 和 `StructType` 定义了数据结构。
接着使用 `createDataFrame` 方法将 RDD 转换为 DataFrame,并按照 `count` 字段降序排序,最后使用 `limit` 和 `toPandas` 方法将结果转换为 Pandas 数据帧。
7. 绘制饼图
```
plt.rcParams['font.family'] = ['sans-serif']
plt.rcParams['font.sans-serif'] = ['SimHei']
plt.pie(result_pdf["count"], labels=result_pdf["tag"], shadow=True, autopct='%1.1f%%')
plt.legend()
plt.show()
```
这里设置 matplotlib 支持中文,然后使用 `pie` 方法绘制饼图,其中 `result_pdf["count"]` 和 `result_pdf["tag"]` 分别表示数据帧中的 `count` 和 `tag` 列。最后使用 `legend` 和 `show` 方法显示图表。
阅读全文
相关推荐
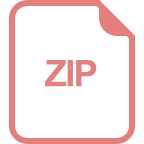
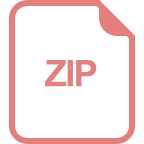
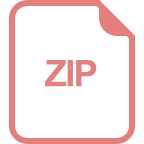
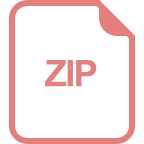
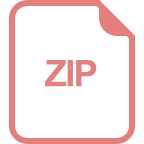
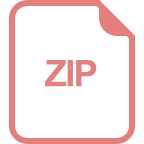
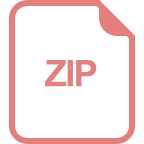
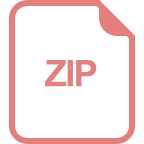
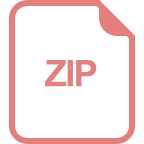
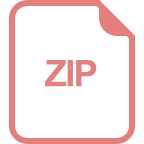
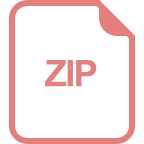
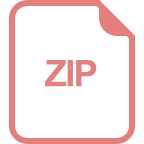
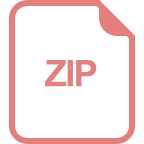
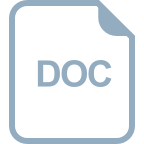
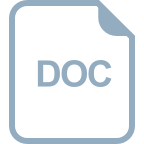

