linux中cp -raf命令的用法
时间: 2024-06-15 22:06:34 浏览: 155
在Linux中,`cp -raf`命令用于复制文件和目录,并且会递归地复制子目录和文件。下面是该命令的用法:
`cp -raf <source> <destination>`
其中,`<source>`表示要复制的源文件或目录的路径,`<destination>`表示要将源文件或目录复制到的目标路径。
该命令的选项说明如下:
- `-r`:递归地复制目录及其内容。
- `-a`:以归档模式复制文件和目录,保留所有属性,包括权限、所有者、组、时间戳等。
请注意,如果目标路径已经存在同名文件或目录,使用`cp -raf`命令会覆盖目标文件或目录。
相关问题
pytorch代码实现在模型中使用LDAMLoss损失函数,数据集是RAF-DB,使用了mixup数据增强
好的,以下是使用LDAMLoss和mixup数据增强的RAF-DB数据集的PyTorch代码实现:
首先,我们需要导入必要的库:
```python
import torch
import torch.nn as nn
import torch.optim as optim
import torchvision.transforms as transforms
from torch.utils.data import DataLoader, Dataset
from torch.autograd import Variable
import numpy as np
import random
from PIL import Image
```
接下来,我们需要定义LDAM(Large Margin Cosine Loss)损失函数:
```python
class LDAMLoss(nn.Module):
def __init__(self, cls_num_list, max_m=0.5, weight=None, s=30):
super(LDAMLoss, self).__init__()
m_list = np.divide(1, np.sqrt(np.sqrt(cls_num_list)))
m_list = np.multiply(max_m, m_list)
m_list = torch.FloatTensor(m_list).cuda()
self.m_list = m_list
assert s > 0
self.s = s
if weight is not None:
self.weight = torch.FloatTensor(weight).cuda()
else:
self.weight = weight
def forward(self, x, target):
cosine = x
sine = torch.sqrt(1.0 - torch.pow(cosine, 2))
phi = cosine * self.m_list.unsqueeze(1) - sine * self.m_list.unsqueeze(1)
phi = phi.float()
target = target.long().view(-1, 1)
index = torch.zeros_like(phi)
index.scatter_(1, target, 1)
if self.weight is not None:
weight = self.weight.unsqueeze(0)
index = torch.matmul(index, weight.t())
index = index.clamp(min=1e-12, max=1 - 1e-12)
index = index.log()
loss = -index * torch.pow(torch.abs(phi), self.s)
loss = loss.sum(dim=1).mean()
else:
index = index.cuda()
loss = -torch.log(torch.abs(torch.gather(phi, 1, target)) + 1e-8)
loss = loss.squeeze(1)
loss = loss.mean()
return loss
```
接下来,我们需要定义mixup数据增强:
```python
def mixup_data(x, y, alpha=1.0):
if alpha > 0:
lam = np.random.beta(alpha, alpha)
else:
lam = 1
batch_size = x.size()[0]
index = torch.randperm(batch_size).cuda()
mixed_x = lam * x + (1 - lam) * x[index, :]
y_a, y_b = y, y[index]
return mixed_x, y_a, y_b, lam
```
然后,我们需要定义RAF-DB数据集的类:
```python
class RAFDataset(Dataset):
def __init__(self, data_path, transform=None):
self.data_path = data_path
self.transform = transform
self.data = []
self.labels = []
with open(self.data_path, 'r') as f:
for line in f:
line = line.strip()
img_path, label = line.split(' ')
self.data.append(img_path)
self.labels.append(int(label))
def __len__(self):
return len(self.data)
def __getitem__(self, index):
img_path = self.data[index]
label = self.labels[index]
img = Image.open(img_path).convert('RGB')
if self.transform is not None:
img = self.transform(img)
return img, label
```
接下来,我们需要定义模型:
```python
class MyModel(nn.Module):
def __init__(self):
super(MyModel, self).__init__()
self.conv1 = nn.Conv2d(3, 64, kernel_size=3, stride=1, padding=1)
self.bn1 = nn.BatchNorm2d(64)
self.relu1 = nn.ReLU(inplace=True)
self.conv2 = nn.Conv2d(64, 128, kernel_size=3, stride=1, padding=1)
self.bn2 = nn.BatchNorm2d(128)
self.relu2 = nn.ReLU(inplace=True)
self.conv3 = nn.Conv2d(128, 256, kernel_size=3, stride=1, padding=1)
self.bn3 = nn.BatchNorm2d(256)
self.relu3 = nn.ReLU(inplace=True)
self.conv4 = nn.Conv2d(256, 512, kernel_size=3, stride=1, padding=1)
self.bn4 = nn.BatchNorm2d(512)
self.relu4 = nn.ReLU(inplace=True)
self.pool = nn.MaxPool2d(kernel_size=2, stride=2)
self.fc1 = nn.Linear(512 * 12 * 12, 1024)
self.drop1 = nn.Dropout(p=0.5)
self.relu5 = nn.ReLU(inplace=True)
self.fc2 = nn.Linear(1024, 7)
def forward(self, x):
x = self.conv1(x)
x = self.bn1(x)
x = self.relu1(x)
x = self.conv2(x)
x = self.bn2(x)
x = self.relu2(x)
x = self.conv3(x)
x = self.bn3(x)
x = self.relu3(x)
x = self.conv4(x)
x = self.bn4(x)
x = self.relu4(x)
x = self.pool(x)
x = x.view(-1, 512 * 12 * 12)
x = self.fc1(x)
x = self.drop1(x)
x = self.relu5(x)
x = self.fc2(x)
return x
```
最后,我们需要定义训练和测试函数:
```python
def train(model, train_loader, optimizer, criterion, alpha):
model.train()
train_loss = 0
train_correct = 0
train_total = 0
for i, (inputs, targets) in enumerate(train_loader):
inputs, targets_a, targets_b, lam = mixup_data(inputs, targets, alpha=alpha)
inputs, targets_a, targets_b = map(Variable, (inputs, targets_a, targets_b))
optimizer.zero_grad()
outputs = model(inputs)
loss = criterion(outputs, targets_a) * lam + criterion(outputs, targets_b) * (1 - lam)
loss.backward()
optimizer.step()
train_loss += loss.item()
_, predicted = torch.max(outputs.data, 1)
train_total += targets.size(0)
train_correct += (lam * predicted.eq(targets_a.data).cpu().sum().float()
+ (1 - lam) * predicted.eq(targets_b.data).cpu().sum().float())
train_acc = train_correct / train_total
train_loss = train_loss / len(train_loader)
return train_acc, train_loss
def test(model, test_loader, criterion):
model.eval()
test_loss = 0
test_correct = 0
test_total = 0
with torch.no_grad():
for inputs, targets in test_loader:
inputs, targets = Variable(inputs), Variable(targets)
outputs = model(inputs)
loss = criterion(outputs, targets)
test_loss += loss.item()
_, predicted = torch.max(outputs.data, 1)
test_total += targets.size(0)
test_correct += predicted.eq(targets.data).cpu().sum().float()
test_acc = test_correct / test_total
test_loss = test_loss / len(test_loader)
return test_acc, test_loss
```
最后,我们需要定义主函数:
```python
if __name__ == '__main__':
# 设置随机种子,确保实验的可重复性
torch.manual_seed(233)
np.random.seed(233)
random.seed(233)
# 定义训练参数
batch_size = 64
num_epochs = 100
lr = 0.1
alpha = 1.0
cls_num_list = [2000, 2000, 2000, 2000, 2000, 2000, 2000]
train_data_path = 'train.txt'
test_data_path = 'test.txt'
# 定义数据增强和数据集
transform_train = transforms.Compose([
transforms.RandomCrop(44),
transforms.RandomHorizontalFlip(),
transforms.ToTensor(),
transforms.Normalize(mean=[0.5, 0.5, 0.5], std=[0.5, 0.5, 0.5])
])
transform_test = transforms.Compose([
transforms.CenterCrop(44),
transforms.ToTensor(),
transforms.Normalize(mean=[0.5, 0.5, 0.5], std=[0.5, 0.5, 0.5])
])
train_dataset = RAFDataset(train_data_path, transform=transform_train)
test_dataset = RAFDataset(test_data_path, transform=transform_test)
# 定义数据加载器
train_loader = DataLoader(train_dataset, batch_size=batch_size, shuffle=True, num_workers=4)
test_loader = DataLoader(test_dataset, batch_size=batch_size, shuffle=False, num_workers=4)
# 定义模型和优化器
model = MyModel().cuda()
optimizer = optim.SGD(model.parameters(), lr=lr, momentum=0.9, weight_decay=5e-4)
criterion = LDAMLoss(cls_num_list)
# 训练和测试
for epoch in range(num_epochs):
train_acc, train_loss = train(model, train_loader, optimizer, criterion, alpha)
test_acc, test_loss = test(model, test_loader, criterion)
print('Epoch [{}/{}], Train Loss: {:.4f}, Train Acc: {:.4f}, Test Loss: {:.4f}, Test Acc: {:.4f}'
.format(epoch + 1, num_epochs, train_loss, train_acc, test_loss, test_acc))
if (epoch + 1) % 10 == 0:
lr /= 10
optimizer = optim.SGD(model.parameters(), lr=lr, momentum=0.9, weight_decay=5e-4)
```
raf-db download
raf-db 是一个用于检索和分析 RNA 剪接异构体和转录本的数据库。用户可以通过在线平台或下载数据集进行研究工作。raf-db download 用于提供用户下载数据库中的数据集和相关信息。用户可以通过在网站上注册账号并遵循相关指引,获得对数据库的访问权限。在获得访问权限后,用户可以通过 download 功能将感兴趣的数据集下载到本地计算机中,方便在离线状态下进行分析和研究。raf-db 提供了高质量的数据集和工具,以帮助用户深入了解和研究 RNA 剪接异构体和转录本的相关信息。通过下载数据库中的数据集,用户可以在自己的研究中使用这些数据来验证假设、进行数据分析、进行实验设计等工作,从而促进该领域的研究发展和进步。raf-db download 是一个方便、实用的工具,为科研工作者提供了便利,使他们能够更高效地获取和使用数据库中的数据,从而推动 RNA 剪接异构体和转录本的研究。同时,通过下载数据库中的数据集,用户还可以对数据进行定制化处理和分析,以满足其特定的研究需求。总之,raf-db download 是一个重要的数据获取工具,为用户提供了便利,并为 RNA 剪接异构体和转录本的研究和应用提供了有力支持。
阅读全文
相关推荐
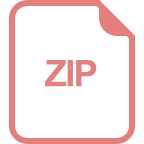
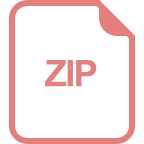
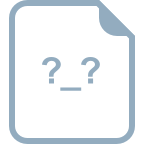
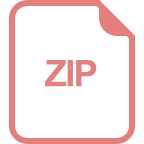
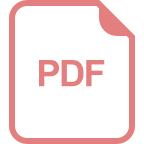
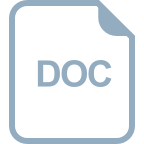
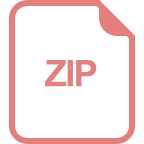
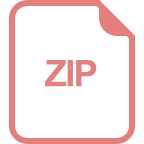
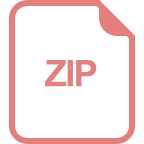
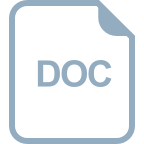
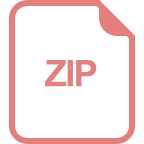
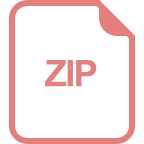
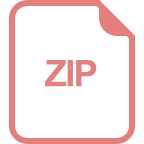