data preprocessing
时间: 2023-04-21 13:00:17 浏览: 87
数据预处理是指在进行数据分析和建模之前,对原始数据进行清洗、转换、集成和规范化等一系列操作的过程。其目的是为了提高数据的质量和可用性,减少数据分析和建模的误差和偏差,从而提高数据分析和建模的准确性和可靠性。数据预处理包括数据清洗、数据转换、数据集成和数据规范化等步骤。
相关问题
Data preprocessing is a critical procedure in many real world machine learning and AI problem. Using weather forecast as example, various data preprocessing such as data normalization, scaling and labeling are needed before the time-series weather information can be used for network training and testing. Use the time series weather data of Seattle (weather.csv) provided in this workshop as the time-series raw data for data preprocessing: Describe and explain the nature of data in each attribute of the time series records. Discuss what kind of data preprocessing methods are needed for each attribute. How about missing record and incorrect data, how can we fix such problems. Write Python program to implement the data processing method. Hint: The normal range and condition of each weather attribute are: Air Pressure 900 - 1200 Precipitation 0 - 300 Temperature -50 - 50 Max >= Min Temp Wind Speed (Grade) 0 - 10 Wind Direction 0 - 360
每个属性的时间序列记录中的数据性质如下:
1. 空气压力:连续数值数据,范围在900-120之间,需要进行数据标准化和缩放。
2. 降水量:连续数值数据,范围在-300之间,需要进行数据标准化和缩放。
3. 温度:连续数值数据,范围在-50到50之间,需要进行数据标准化和缩放。
4. 最高温度和最低温度:连续数值数据,范围在-50到50之间,需要进行数据标准化和缩放。
5. 风速(等级):离散数值数据,范围在-10之间,需要进行数据标准化和缩放。
6. 风向:连续数值数据,范围在-360之间,需要进行数据标准化和缩放。
对于缺失记录和不正确的数据,我们可以使用插值方法来填充缺失值或者删除不正确的数据。
以下是Python程序实现数据处理方法的示例代码:
```python
import pandas as pd
from sklearn.preprocessing import MinMaxScaler
# 读取数据
data = pd.read_csv('weather.csv')
# 处理空值
data = data.fillna(method='ffill')
# 标准化和缩放
scaler = MinMaxScaler()
data[['Air Pressure', 'Precipitation', 'Temperature', 'Max Temperature', 'Min Temperature', 'Wind Speed (mph)', 'Wind Direction (degrees)']] = scaler.fit_transform(data[['Air Pressure', 'Precipitation', 'Temperature', 'Max Temperature', 'Min Temperature', 'Wind Speed (mph)', 'Wind Direction (degrees)']])
# 输出处理后的数据
print(data)
```
import tkinter as tk from tkinter import filedialog import pandas as pd import numpy as np from sklearn.preprocessing import StandardScaler class DataImporter: def init(self, master): self.file_path = self.master = master self.master.title("数据导入") # 创建用于显示文件路径的标签 self.path_label = tk.Label(self.master, text="请先导入数据集!") self.path_label.pack(pady=10) # 创建“导入数据集”按钮 self.load_button = tk.Button(self.master, text="导入数据集", command=self.load_data) self.load_button.pack(pady=10) # 创建“显示数据集”按钮 self.show_button = tk.Button(self.master, text="显示数据集", command=self.show_data) self.show_button.pack(pady=10) # 创建“退出程序”按钮 self.quit_button = tk.Button(self.master, text="退出程序", command=self.master.quit) self.quit_button.pack(pady=10) # 创建一个空的 DataFrame 用于存放数据集 self.data = pd.DataFrame() def load_data(self): # 弹出文件选择对话框 file_path = filedialog.askopenfilename() # 如果用户选择了文件,则导入数据集 if file_path: self.data = pd.read_csv(file_path) self.path_label.config(text=f"已导入数据集:{file_path}") else: self.path_label.config(text="未选择任何文件,请选择正确的文件") def show_data(self): if not self.data.empty: # 创建一个新窗口来显示数据集 top = tk.Toplevel(self.master) top.title("数据集") # 创建用于显示数据集的表格 table = tk.Text(top) table.pack() # 将数据集转换为字符串并显示在表格中 table.insert(tk.END, str(self.data)) table.config(state=tk.DISABLED) # 创建“数据预处理”按钮 process_button = tk.Button(top, text="数据预处理", command=self.process_data) process_button.pack(pady=10) else: self.path_label.config(text="请先导入数据集") def process_data(self): try: self.data = pd.read_csv(self.file_path) missing_values = self.data.isnull().sum() for col in self.data.columns: mean = np.mean(self.data[col]) std = np.std(self.data[col]) outliers = [x for x in self.data[col] if (x > mean + 2 * std)] if len(outliers) > 0: print('Column {} has outliers: {}'.format(col, outliers)) scaler = StandardScaler() data_scaled = scaler.fit_transform(self.data) print('Data preprocessing completed.') except Exception as e: print('Error: ' + str(e)) if name == "main": root = tk.Tk() app = DataImporter(root) root.geometry("400x300+100+100") root.mainloop()上面的这段代码中,file_path么有定义属性,帮我按照代码的环境,补全属性
在 DataImporter 类中,需要将 self.file_path 定义为类的属性。可以在初始化方法中进行定义,如下所示:
```python
class DataImporter:
def __init__(self, master):
self.file_path = "" # 定义 file_path 属性
self.master = master
self.master.title("数据导入")
self.path_label = tk.Label(self.master, text="请先导入数据集!")
self.path_label.pack(pady=10)
self.load_button = tk.Button(self.master, text="导入数据集", command=self.load_data)
self.load_button.pack(pady=10)
self.show_button = tk.Button(self.master, text="显示数据集", command=self.show_data)
self.show_button.pack(pady=10)
self.quit_button = tk.Button(self.master, text="退出程序", command=self.master.quit)
self.quit_button.pack(pady=10)
self.data = pd.DataFrame()
def load_data(self):
self.file_path = filedialog.askopenfilename() # 更新 file_path 属性
if self.file_path:
self.data = pd.read_csv(self.file_path)
self.path_label.config(text=f"已导入数据集:{self.file_path}")
else:
self.path_label.config(text="未选择任何文件,请选择正确的文件")
...
```
这样,每次用户选择文件后,就会将文件路径保存到 self.file_path 属性中,方便后续对数据进行处理。
相关推荐
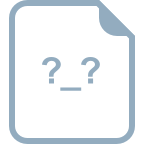
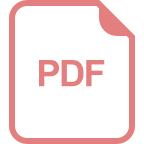












