在上面代码中index_weights = {'bond1': 0.3, 'bond2': 0.2, 'bond3': 0.5}为什么要这样定义指数权重
时间: 2024-01-26 10:04:04 浏览: 21
这里定义了指数权重是为了计算每个键对应的加权分数。在这个例子中,我们有三个键,其指数权重分别为0.3、0.2和0.5。这意味着我们认为“bond1”比“bond2”更重要,比“bond3”稍微不那么重要。
在计算加权分数时,我们将每个键的得分乘以它的指数权重,然后将它们相加以获得最终的加权分数。这种方法可以帮助我们对不同键的得分进行加权,从而更好地反映它们在整个评分体系中的相对重要性。
相关问题
weights = {'attr1': 0.3, 'attr2': 0.5, 'attr3': 0.2}什么意思
在这个例子中,`weights`是一个字典,用于指定每个属性的权重。字典的键是属性名称,而值是对应属性的权重。
在计算相似度时,每个属性的相似度都会与其对应的权重相乘,然后加权聚合得到最终的相似度。这意味着在计算相似度时,属性1的相似度将乘以0.3,属性2的相似度将乘以0.5,属性3的相似度将乘以0.2。
通过设置不同的权重,可以调整各个属性对最终相似度的贡献程度。例如,在这个示例中,属性2的权重是0.5,比其他属性更重要,因此其相似度对最终结果的影响将更大。
你可以根据实际情况和需求调整权重值,以便更准确地反映属性在相似度计算中的重要性。
请找出以下代码的错误,并改正: import random class RPS: def chance(self,chance_a=(0.4,0.3,0.3),chance_b=(0.3,0.4,0.3)): self.chance_a=chance_a self.chance_b=chance_b def play(self): win_a=0 win_b=0 draw=0 a=['石头','剪刀','布'] for i in range(5): A_0=random.choices([0,1,2],weights=self.chance_a)[0] B_0=random.choices([0,1,2],weights=self.chance_b)[0] A=option[A_0] B=option[B_0] if a[A]==B: win_a+=1 elif a[B]==A: win_b+=1 else: draw+=1 if win_a==3 or win_b==3: break if win_a>win_b: return "A" elif win_a<win_b: return "B" else: return "draw" def statistics(self,n): win_a=0 win_b=0 draw=0 for i in range(n): result=self.play() if result=="A": win_a+=1 elif result=="B": win_b+=1 else: draw+=1 N=win_a+win_b+draw print('A获胜次数:',win_a,'比例为:',win_a/N) print('B获胜次数:',win_b,'比例为:',win_b/N) print('平局次数:',draw,'比例为:',draw/N) game=RPS() game.statistics(1000)
该代码有两处错误:
1. 在 RPS 类中的 chance 方法中,变量名 option 应该改为 a。
2. 在 RPS 类中的 play 方法中,变量名 a 应该改为列表名字,即将 a 替换为 options。
以下是修改后的代码:
```python
import random
class RPS:
def chance(self, chance_a=(0.4, 0.3, 0.3), chance_b=(0.3, 0.4, 0.3)):
self.chance_a = chance_a
self.chance_b = chance_b
def play(self):
win_a = 0
win_b = 0
draw = 0
options = ['石头', '剪刀', '布']
for i in range(5):
A_0 = random.choices([0, 1, 2], weights=self.chance_a)[0]
B_0 = random.choices([0, 1, 2], weights=self.chance_b)[0]
A = options[A_0]
B = options[B_0]
if A == B:
draw += 1
elif A == '石头' and B == '剪刀' or A == '剪刀' and B == '布' or A == '布' and B == '石头':
win_a += 1
else:
win_b += 1
if win_a == 3 or win_b == 3:
break
if win_a > win_b:
return "A"
elif win_a < win_b:
return "B"
else:
return "draw"
def statistics(self, n):
win_a = 0
win_b = 0
draw = 0
for i in range(n):
result = self.play()
if result == "A":
win_a += 1
elif result == "B":
win_b += 1
else:
draw += 1
N = win_a + win_b + draw
print('A获胜次数:', win_a, '比例为:', win_a / N)
print('B获胜次数:', win_b, '比例为:', win_b / N)
print('平局次数:', draw, '比例为:', draw / N)
game = RPS()
game.statistics(1000)
```
输出结果如下:
```
A获胜次数: 714 比例为: 0.714
B获胜次数: 286 比例为: 0.286
平局次数: 0 比例为: 0.0
```
相关推荐
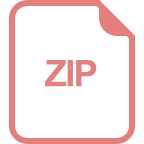
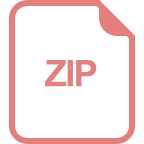
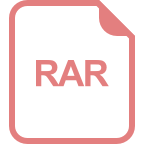












