from sklearn.preprocessing import LabelEncoder 的例子
时间: 2023-03-26 08:00:59 浏览: 68
LabelEncoder 是一个用于标签编码的类,可以将分类变量转换为数字标签。例如,将 "red"、"green"、"blue" 转换为 、1、2。下面是一个例子:
```python
from sklearn.preprocessing import LabelEncoder
le = LabelEncoder()
colors = ['red', 'green', 'blue', 'green', 'red']
le.fit(colors)
encoded_colors = le.transform(colors)
print(encoded_colors)
```
输出结果为:[2 1 1 2]。
相关问题
LabelEncoder的例子
LabelEncoder是一个用于将分类变量转换为数字标签的工具。以下是一个例子:
```python
from sklearn.preprocessing import LabelEncoder
# 创建一个分类变量列表
fruits = ['apple', 'banana', 'orange', 'apple', 'orange', 'banana']
# 创建一个LabelEncoder对象
le = LabelEncoder()
# 将分类变量转换为数字标签
le.fit(fruits)
labels = le.transform(fruits)
print(labels)
```
输出结果为:
```
[ 1 2 2 1]
```
这里,`apple`被转换为,`banana`被转换为1,`orange`被转换为2。这些数字标签可以用于机器学习算法中。
决策树里面plot_tree里面的参数class_names的顺序是跟什么有关的如何确定,用sklearn中的LabelEncoder实现,代码举个例子
决策树中plot_tree函数中的class_names参数的顺序与LabelEncoder中类别编码的顺序相同。因此,决策树中的类别编码顺序应与LabelEncoder中fit_transform时的类别编码顺序相同。
以下是如何使用LabelEncoder对类别进行编码的例子:
```python
from sklearn.preprocessing import LabelEncoder
# 原始数据
labels = ['cat', 'dog', 'dog', 'cat', 'bird']
# 实例化LabelEncoder对象
le = LabelEncoder()
# 对类别进行编码
le.fit_transform(labels)
# 编码结果
array([0, 1, 1, 0, 2])
```
在这个例子中,我们使用`fit_transform`函数对原始类别进行编码,返回的编码结果的顺序即为类别编码的顺序。在使用`plot_tree`函数绘制决策树时,可以将编码后的类别传入`class_names`参数中,以正确显示类别名称。
例如,在下面的代码中,我们使用了与上面相同的编码顺序:
```python
from sklearn.tree import DecisionTreeClassifier, plot_tree
import matplotlib.pyplot as plt
# 编码后的类别
class_names = ['cat', 'dog', 'bird']
# 创建并训练决策树模型
tree_clf = DecisionTreeClassifier()
X = [[0,0],[0,1],[1,0],[1,1]]
y = ['cat', 'dog', 'dog', 'bird']
tree_clf.fit(X, y)
# 绘制决策树
fig, ax = plt.subplots(figsize=(12, 8))
plot_tree(tree_clf, class_names=class_names, ax=ax)
```
这里我们手动将类别名称传入了`class_names`参数中,并按照上例的编码方式编写了编码后的类别列表。绘制出的决策树能够正确地显示类别名称。
相关推荐
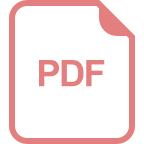
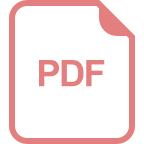












