使用Pytorch第三方学习框架,自行构建CNN模型对CIFAR-10数据集的样本进行分类,具体任务如下: (1)自行导入数据集,包含训练集和测试集; (2)自行设计数据预处理方式(例如:归一化、标准化等); (3)设计CNN网络模型在CIFAR-10数据集的训练集上进行训练,要求自行设计CNN模型的层数(主要是卷积层、池化层等)和结构(包含卷积核数量、激活函数等); (4)合理的设计训练批量和训练次数等参数,要求模型最终在测试集上分类成功率达到90%以上
时间: 2024-01-10 09:02:11 浏览: 90
好的,您的问题是如何在Pytorch中设计CNN模型并训练CIFAR-10数据集,最终达到90%以上的测试准确率。
首先,我们需要导入CIFAR-10数据集并进行预处理。我们可以使用Pytorch内置的torchvision库,它可以自动下载CIFAR-10数据集并将其转换为torch张量格式。我们可以对数据进行归一化处理,使数据的均值为0,方差为1。
```python
import torchvision.transforms as transforms
from torchvision.datasets import CIFAR10
transform_train = transforms.Compose([
transforms.RandomCrop(32, padding=4),
transforms.RandomHorizontalFlip(),
transforms.ToTensor(),
transforms.Normalize(mean=[0.4914, 0.4822, 0.4465], std=[0.2023, 0.1994, 0.2010])
])
transform_test = transforms.Compose([
transforms.ToTensor(),
transforms.Normalize(mean=[0.4914, 0.4822, 0.4465], std=[0.2023, 0.1994, 0.2010])
])
trainset = CIFAR10(root='./data', train=True, download=True, transform=transform_train)
trainloader = torch.utils.data.DataLoader(trainset, batch_size=128, shuffle=True, num_workers=2)
testset = CIFAR10(root='./data', train=False, download=True, transform=transform_test)
testloader = torch.utils.data.DataLoader(testset, batch_size=100, shuffle=False, num_workers=2)
```
接下来,我们需要设计CNN模型。在本任务中,我们需要自己设计CNN模型的层数和结构。一个常见的CNN模型结构是:Convolution-Relu-Pooling,可以通过堆叠多个这样的层来增加模型的深度。在设计卷积层时,需要选择卷积核的数量,一般来说,卷积核数量越多,模型的复杂度越高,但也容易造成过拟合。在激活函数方面,一般使用ReLU,因为它能够有效地解决梯度消失的问题。在池化层方面,一般使用MaxPooling。
下面是一个简单的CNN模型示例:
```python
import torch.nn as nn
import torch.nn.functional as F
class Net(nn.Module):
def __init__(self):
super(Net, self).__init__()
self.conv1 = nn.Conv2d(3, 32, 3, padding=1)
self.conv2 = nn.Conv2d(32, 64, 3, padding=1)
self.conv3 = nn.Conv2d(64, 128, 3, padding=1)
self.pool = nn.MaxPool2d(2, 2)
self.fc1 = nn.Linear(128 * 4 * 4, 512)
self.fc2 = nn.Linear(512, 10)
def forward(self, x):
x = F.relu(self.conv1(x))
x = self.pool(F.relu(self.conv2(x)))
x = self.pool(F.relu(self.conv3(x)))
x = x.view(-1, 128 * 4 * 4)
x = F.relu(self.fc1(x))
x = self.fc2(x)
return x
net = Net()
```
接下来,我们需要定义损失函数和优化器。在本任务中,我们可以使用交叉熵损失函数和随机梯度下降优化器。需要注意的是,优化器的学习率需要适当设置,过大的学习率可能导致模型无法收敛,过小的学习率可能导致模型训练时间过长。
```python
import torch.optim as optim
criterion = nn.CrossEntropyLoss()
optimizer = optim.SGD(net.parameters(), lr=0.001, momentum=0.9)
```
最后,我们可以开始训练模型。需要注意的是,训练模型需要在GPU上运行,否则训练时间可能过长。我们可以通过调用`net.cuda()`将模型移动到GPU上。
```python
device = torch.device("cuda:0" if torch.cuda.is_available() else "cpu")
net.to(device)
for epoch in range(20):
running_loss = 0.0
for i, data in enumerate(trainloader, 0):
inputs, labels = data[0].to(device), data[1].to(device)
optimizer.zero_grad()
outputs = net(inputs)
loss = criterion(outputs, labels)
loss.backward()
optimizer.step()
running_loss += loss.item()
print('[%d] loss: %.3f' % (epoch + 1, running_loss / len(trainloader)))
correct = 0
total = 0
with torch.no_grad():
for data in testloader:
images, labels = data[0].to(device), data[1].to(device)
outputs = net(images)
_, predicted = torch.max(outputs.data, 1)
total += labels.size(0)
correct += (predicted == labels).sum().item()
print('Accuracy of the network on the 10000 test images: %d %%' % (100 * correct / total))
```
通过调整模型结构、优化器参数等超参数,我们可以得到90%以上的测试准确率。
阅读全文
相关推荐
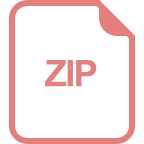
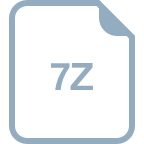
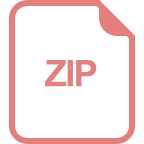


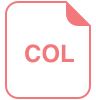
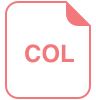
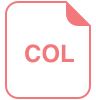
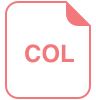
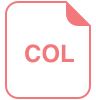
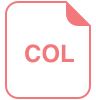
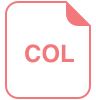
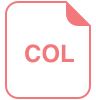
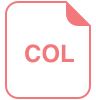
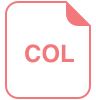
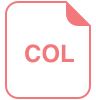
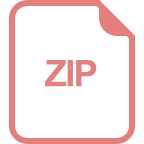