function [x,A] = PageRankScores(A_hat,epsilon) % [Note the extra output A here is for MATLAB Grader testing only.] % Use A_hat to form the probability matrix described on the question sheet n = size(A_hat, 1); D = sum(A_hat, 1); P = A_hat./D; TF = isnan(P);%判断是否为NaN P(TF) = ones(n,1)./n;%将NaN都替换为0 % ... then calculate the that into a "PageRank" matrix for this input value % of epsilon ... A = epsilon.*P+(1-epsilon)/n.*ones(n,n); % ... and use power_method to find the dominant eigenvalue & corresponding % eigenvector. x0 = ones(n, 1)/n; [x,lam] = power_method(A,x0); x=x/sum(x); % Ensure x is a probability vector, that is all 0<=x_j<=1 and sum(x)=1. if any(x < 0) || any(x > 1) error('all 0<=x_j<=1'); end if abs(sum(x) - 1) > 1e-6 error('sum(x) should be 1'); end end
时间: 2023-06-26 13:08:35 浏览: 45
这是一个 MATLAB 函数,它实现了 PageRank 算法,用于计算网页的权重得分。该函数的输入是一个邻接矩阵 A_hat 和一个参数 epsilon,输出是一个 PageRank 得分向量 x 和一个概率矩阵 A(仅用于 MATLAB Grader 测试)。函数首先使用邻接矩阵 A_hat 来构建概率矩阵 P,然后使用参数 epsilon 将其加权并与一个平均概率矩阵相加,得到一个新的概率矩阵 A。接着使用幂迭代法求解矩阵 A 的主特征值和对应的特征向量 x。最后,函数确保 x 是一个概率向量,即各个元素都在 0 到 1 之间,且所有元素之和为 1。如果 x 不满足这些条件,则会抛出错误。
相关问题
function [x,A] = PageRankScores(A_hat,epsilon) % [Note the extra output A here is for MATLAB Grader testing only.] % Use A_hat to form the probability matrix described on the question sheet n = size(A_hat, 1); D = sum(A_hat, 1); P = A_hat./D; TF = isnan(P);%判断是否为NaN P(TF) = ones(n,1)./n;%将NaN都替换为0 % ... then calculate the that into a "PageRank" matrix for this input value % of epsilon ... A = epsilon.*P+(1-epsilon)/n.*ones(n,n); % ... and use power_method to find the dominant eigenvalue & corresponding % eigenvector. x0 = ones(n, 1)/n; [x,lam] = power_method(A,x0); x=x/sum(x); % Ensure x is a probability vector, that is all 0<=x_j<=1 and sum(x)=1. if any(x < 0) || any(x > 1) error('all 0<=x_j<=1'); end if abs(sum(x) - 1) > 1e-6 error('sum(x) should be 1'); end end它报这个错误Double check that the output of your function x is a column vector of size
The error message is telling you to check if the output of your function `x` is a column vector of the expected size. You can use the `size` function to check the size of `x`.
In this case, the expected size is not specified in the error message. However, based on the function signature, we can assume that `x` should be a column vector with the same number of rows as the input matrix `A_hat`. So, you can check if `x` is a column vector and has the same number of rows as `A_hat` by adding the following code at the end of the function:
```
if ~iscolumn(x) || size(x,1) ~= size(A_hat,1)
error('The output x is not a column vector of the expected size.');
end
```
This will throw an error if `x` is not a column vector or has the wrong number of rows.
class DQNAgent: def __init__(self, input_dim, output_dim, learning_rate=0.001, pretrained=True): self.network = DQN(input_dim, output_dim, pretrained=pretrained) self.target_network = DQN(input_dim, output_dim, pretrained=pretrained) self.buffer = ReplayBuffer(1000) self.optimizer = optim.Adam(self.network.parameters(), lr=learning_rate) self.criteria = nn.MSELoss() self.gamma = 0.9 self.epsilon = 0 self.epsilon_decay = 0.999 self.epsilon_min = 0.05 self.output_dim = output_dim
这是一个基于DQN算法的智能体(Agent)类。它的作用是实现一个DQN智能体,用于解决强化学习中的决策问题。主要有以下几个成员:
1. `__init__(self, input_dim, output_dim, learning_rate=0.001, pretrained=True)`:初始化方法,传入输入维度(input_dim)、输出维度(output_dim)、学习率(learning_rate)和是否使用预训练(pretrained)模型。在初始化过程中,它创建了两个DQN网络实例:self.network和self.target_network,以及一个经验回放缓冲区实例self.buffer。同时,它还定义了优化器(self.optimizer)和损失函数(self.criteria)。
2. `self.network = DQN(input_dim, output_dim, pretrained=pretrained)`:创建一个DQN网络实例,用于近似值函数的估计。该网络将输入维度(input_dim)和输出维度(output_dim)作为参数传入,并根据预训练(pretrained)标志来初始化模型参数。
3. `self.target_network = DQN(input_dim, output_dim, pretrained=pretrained)`:创建一个目标网络实例,用于计算目标Q值。与self.network类似,它也接受输入维度(input_dim)和输出维度(output_dim)作为参数,并根据预训练(pretrained)标志来初始化模型参数。
4. `self.buffer = ReplayBuffer(1000)`:创建一个经验回放缓冲区实例,用于存储智能体与环境之间的交互数据。它的容量为1000,可以根据需要进行调整。
5. `self.optimizer = optim.Adam(self.network.parameters(), lr=learning_rate)`:创建一个Adam优化器实例,用于更新网络参数。它的参数是self.network的可学习参数,学习率为learning_rate。
6. `self.criteria = nn.MSELoss()`:创建一个均方误差损失函数实例,用于计算值函数的误差。它将用于计算网络输出与目标Q值之间的差距。
7. `self.gamma = 0.9`:折扣因子,用于计算未来奖励的折现值。
8. `self.epsilon = 0`:ε-greedy策略中的ε值,用于探索与利用的权衡。
9. `self.epsilon_decay = 0.999`:ε值的衰减率,用于逐渐减小探索的概率。
10. `self.epsilon_min = 0.05`:ε值的最小值,探索的概率不会低于这个值。
11. `self.output_dim = output_dim`:输出维度。
该类将DQN算法的各个组件进行了封装,并提供了一些方法来实现智能体的训练和决策过程。
相关推荐
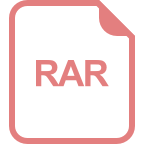
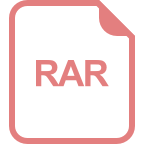
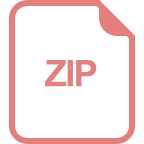













