def block1(x, filters, kernel_size=3, stride=1, conv_shortcut=True, name=None): """A residual block. Arguments: x: input tensor. filters: integer, filters of the bottleneck layer. kernel_size: default 3, kernel size of the bottleneck layer. stride: default 1, stride of the first layer. conv_shortcut: default True, use convolution shortcut if True, otherwise identity shortcut. name: string, block label. Returns: Output tensor for the residual block. """ bn_axis = 3 if backend.image_data_format() == 'channels_last' else 1 if conv_shortcut: shortcut = layers.Conv2D( 4 * filters, 1, strides=stride, name=name + '_0_conv')(x) shortcut = layers.BatchNormalization( axis=bn_axis, epsilon=1.001e-5, name=name + '_0_bn')(shortcut) else: shortcut = x #第一个卷积结构 x = layers.Conv2D(filters, 1, strides=stride, name=name + '_1_conv')(x) x = layers.BatchNormalization( axis=bn_axis, epsilon=1.001e-5, name=name + '_1_bn')(x) x = layers.Activation('relu', name=name + '_1_relu')(x) #第二个卷积结构 x = layers.Conv2D( filters, kernel_size, padding='SAME', name=name + '_2_conv')(x) x = layers.BatchNormalization( axis=bn_axis, epsilon=1.001e-5, name=name + '_2_bn')(x) x = layers.Activation('relu', name=name + '_2_relu')(x) #第三个卷积结构 x = layers.Conv2D(4 * filters, 1, name=name + '_3_conv')(x) x = layers.BatchNormalization( axis=bn_axis, epsilon=1.001e-5, name=name + '_3_bn')(x) x = layers.Add(name=name + '_add')([shortcut, x]) x = layers.Activation('relu', name=name + '_out')(x) return x def stack1(x, filters, blocks, stride1=2, name=None): """A set of stacked residual blocks. Arguments: x: input tensor. filters: integer, filters of the bottleneck layer in a block. blocks: integer, blocks in the stacked blocks. stride1: default 2, stride of the first layer in the first block. name: string, stack label. Returns: Output tensor for the stacked blocks. """ x = block1(x, filters, stride=stride1, name=name + '_block1') for i in range(2, blocks + 1): x = block1(x, filters, conv_shortcut=False, name=name + '_block' + str(i)) return x
时间: 2023-11-18 21:06:20 浏览: 256
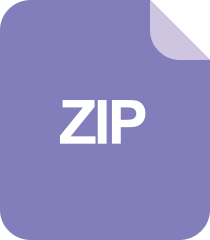
Highway_Conv1d_Keras_Tensorflow2:pytorch,keras和tensorflow2.x中的conv1d公路网络
这是一个用于构建深度残差网络(ResNet)的函数,包含了两个子函数:block1和stack1。其中block1是一个残差块,stack1是一组堆叠的残差块。在ResNet中,每个残差块由三个卷积层组成,其中第一个卷积层可以使用1x1卷积进行下采样,第三个卷积层的输出通道数是第二个卷积层的四倍。每个残差块的输出是输入和最后一个卷积层的输出的和,并经过ReLU激活函数。stack1函数调用block1函数构建一个堆叠的残差块,可以使用stride1参数指定第一个残差块的下采样步长。
阅读全文
相关推荐
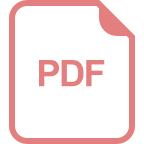
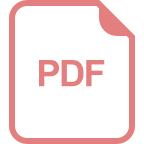
















