建立模型预测这组数据2018-2030的PCI、RQI、RDI、SRI的值 # 创建DataFrame data = { '年份': [2009, 2010, 2011, 2012, 2013, 2014, 2015, 2016, 2107], 'PCI': [91.67, 91.67, 91.39, 91.25, 90.99, 90.15, 88.02, 85.97, 82.74], 'RQI': [92.27, 92.05, 91.77, 91.25, 90.87, 89.72, 87.67, 82.92, 80.07], 'RDI': [96.02, 91.39, 88.10, 87.76, 85.51, 84.94, 82.42, 85.25, 71.60], 'SRI': [92.27, 92.27, 92.77, 91.25, 90.87, 89.72, 91.67, 82.92, 86.07]
时间: 2023-09-23 18:04:21 浏览: 60
为了预测未来几年的PCI、RQI、RDI和SRI的值,你可以使用时间序列分析或回归模型。
对于时间序列分析,你可以考虑使用ARIMA(自回归移动平均)模型或其变体。ARIMA模型可以捕捉时间序列的趋势、季节性和随机性,并进行预测。你可以使用Python中的statsmodels库来拟合ARIMA模型并进行预测。
对于回归模型,你可以使用线性回归或其他回归算法,将年份作为自变量,PCI、RQI、RDI和SRI作为因变量进行拟合。然后,使用训练好的模型进行未来几年的预测。你可以使用Python中的scikit-learn库或其他机器学习库来构建回归模型。
下面是一个示例,演示如何使用线性回归模型进行预测:
```python
import pandas as pd
from sklearn.linear_model import LinearRegression
# 创建DataFrame
data = {
'年份': [2009, 2010, 2011, 2012, 2013, 2014, 2015, 2016, 2107],
'PCI': [91.67, 91.67, 91.39, 91.25, 90.99, 90.15, 88.02, 85.97, 82.74],
'RQI': [92.27, 92.05, 91.77, 91.25, 90.87, 89.72, 87.67, 82.92, 80.07],
'RDI': [96.02, 91.39, 88.10, 87.76, 85.51, 84.94, 82.42, 85.25, 71.60],
'SRI': [92.27, 92.27, 92.77, 91.25, 90.87, 89.72, 91.67, 82.92, 86.07]
}
df = pd.DataFrame(data)
# 构建线性回归模型
model = LinearRegression()
# 拟合模型
model.fit(df[['年份']], df[['PCI', 'RQI', 'RDI', 'SRI']])
# 预测未来几年的值
future_years = [2018, 2019, 2020, 2021, 2022, 2023, 2024, 2025, 2026]
future_data = pd.DataFrame({'年份': future_years})
predictions = model.predict(future_data[['年份']])
# 打印预测结果
print("预测结果:")
for i, year in enumerate(future_years):
print(f"年份: {year}")
print(f"PCI: {predictions[i][0]}")
print(f"RQI: {predictions[i][1]}")
print(f"RDI: {predictions[i][2]}")
print(f"SRI: {predictions[i][3]}")
```
在这个示例中,我们首先创建了一个DataFrame,包含了已知的年份和相应的PCI、RQI、RDI和SRI值。然后,我们使用线性回归模型进行拟合,并使用拟合好的模型进行未来几年的预测。最后,我们打印出预测结果。
请注意,这只是一个简单的示例,实际情况中可能需要考虑更复杂的时间序列模型或改进的回归模型,以及进行数据预处理和模型评估等步骤。
相关推荐
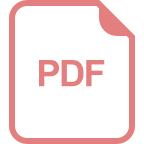
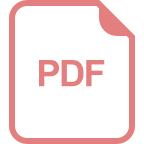


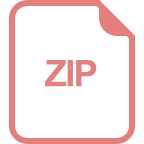
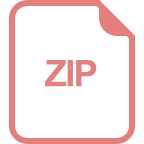
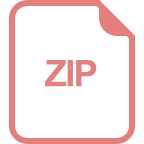
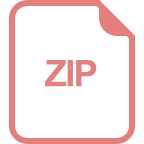
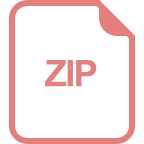
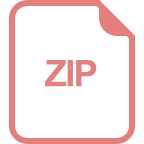
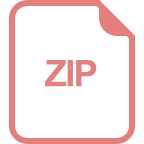
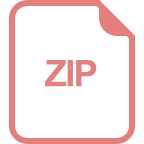