在Python的scikit-learn库中,如何利用过滤法中的VarianceThreshold进行特征选择,并给出实例代码?
时间: 2024-11-16 12:17:19 浏览: 6
在数据预处理和特征工程中,使用VarianceThreshold进行特征选择可以帮助我们去除数据集中方差较低的特征,这些特征往往不携带有效信息。通过Python的scikit-learn库中的VarianceThreshold类,我们可以轻松实现这一过程。
参考资源链接:[监督学习中的特征选择方法:过滤法、嵌入法、包装法](https://wenku.csdn.net/doc/1m6n9u036c?spm=1055.2569.3001.10343)
首先,需要安装scikit-learn库,如果尚未安装,可以使用pip安装命令:
```python
pip install scikit-learn
```
接下来,我们可以使用VarianceThreshold来选择特征。假设我们有一个数据集,我们希望保留那些方差大于某个阈值的特征。以下是一个简单的示例代码:
```python
from sklearn.feature_selection import VarianceThreshold
from sklearn.datasets import make_classification
import numpy as np
# 创建一个模拟数据集
X, y = make_classification(n_samples=1000, n_features=50, n_informative=10, n_redundant=10, random_state=0)
# 初始化VarianceThreshold实例,设置方差阈值为0.1
selector = VarianceThreshold(threshold=0.1)
# 使用fit方法计算各个特征的方差
selector.fit(X)
# 转换数据,仅保留方差大于0.1的特征
X_filtered = selector.transform(X)
# 输出保留的特征数量
print(
参考资源链接:[监督学习中的特征选择方法:过滤法、嵌入法、包装法](https://wenku.csdn.net/doc/1m6n9u036c?spm=1055.2569.3001.10343)
阅读全文
相关推荐
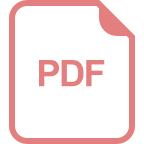
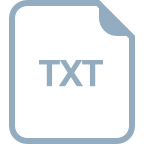
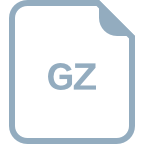

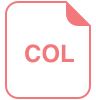
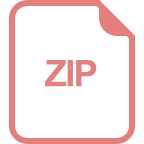
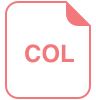
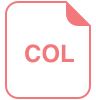
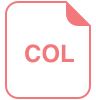
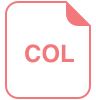
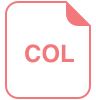
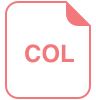
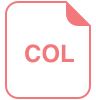
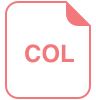
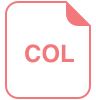
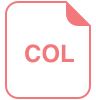
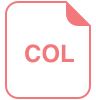
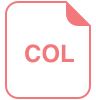