matlab实现混淆矩阵
时间: 2023-10-16 18:28:48 浏览: 55
在 MATLAB 中,可以使用 confusionmat 函数来生成混淆矩阵。这个函数需要两个参数:真实标签和预测标签。
下面是一个示例代码:
```matlab
% 定义真实标签和预测标签
groundTruth = [1 1 2 2 3 3];
predictedLabels = [1 1 2 3 3 3];
% 生成混淆矩阵
confMat = confusionmat(groundTruth, predictedLabels);
% 显示混淆矩阵
disp(confMat);
```
输出结果为:
```
2 0 0
0 1 1
0 0 2
```
其中,混淆矩阵的行表示真实标签,列表示预测标签。例如,第一行第一列的值为 2,表示真实标签为 1,预测标签也为 1 的样本有2个。
如果需要更好的可视化效果,可以使用 plotconfusion 函数。例如:
```matlab
% 显示混淆矩阵
figure;
plotconfusion(groundTruth, predictedLabels);
```
这个函数会生成一个混淆矩阵的可视化图像。
相关问题
matlab代码实现混淆矩阵
您可以使用MATLAB中的confusionmat函数来实现混淆矩阵。该函数接受两个输入参数,一个是真实标签向量,另一个是预测标签向量。下面是一个示例代码:
```matlab
% 真实标签向量和预测标签向量
trueLabels = [1 1 0 0 1 0 1];
predictedLabels = [1 0 0 1 1 0 0];
% 计算混淆矩阵
C = confusionmat(trueLabels, predictedLabels);
% 显示混淆矩阵
disp('混淆矩阵:');
disp(C);
```
运行上述代码后,将输出混淆矩阵C的值:
```
混淆矩阵:
2 1
2 2
```
在这个例子中,真实标签向量中有3个1和4个0,预测标签向量中有3个1和4个0。混淆矩阵C的第一行第一列的值2表示真实标签为1且被正确预测为1的样本数;第一行第二列的值1表示真实标签为1但被错误预测为0的样本数;第二行第一列的值2表示真实标签为0但被错误预测为1的样本数;第二行第二列的值2表示真实标签为0且被正确预测为0的样本数。
您可以根据实际需要修改真实标签和预测标签的向量,以及添加更多的类别。
matlab中混淆矩阵可视化
在Matlab中,可以通过绘制混淆矩阵来对模型的性能进行可视化。混淆矩阵是一个二维矩阵,用于表示分类模型的预测结果与真实标签之间的关系。根据引用中的代码示例,我们可以看到绘制混淆矩阵的具体步骤如下:
1. 首先,需要定义一个混淆矩阵ConfMat,其中包含了真阳性(True Positive, TP)、真阴性(True Negative, TN)、假阳性(False Positive, FP)和假阴性(False Negative, FN)的数量。这些值可以根据具体的分类结果进行统计。
2. 接下来,可以通过使用imagesc函数将混淆矩阵绘制成一个热力图,以直观地显示不同类别之间的分类情况。
3. 为了更好地理解热力图中的内容,可以设置坐标轴的刻度和标签,分别表示类别的真实标签值。
4. 最后,可以使用text函数添加信息,如真阳性、假阳性、真阴性和假阴性的数量,并根据特定的阈值T对其进行颜色标记。
需要注意的是,引用中提到的targets和outputs两个矩阵不是实际预测模型的1xN矩阵,而是MxN矩阵,其中N表示样本数目,M表示样本属性个数或类别数。通过对单样本属性进行多属性扩展,可以将1xN矩阵转换为MxN矩阵,从而进行混淆矩阵的绘制。
综上所述,通过以上步骤,我们可以在Matlab中实现混淆矩阵的可视化。<span class="em">1</span><span class="em">2</span><span class="em">3</span>
#### 引用[.reference_title]
- *1* [理解Matlab中的数组和矩阵:区别与用途](https://download.csdn.net/download/weixin_41784475/88226805)[target="_blank" data-report-click={"spm":"1018.2226.3001.9630","extra":{"utm_source":"vip_chatgpt_common_search_pc_result","utm_medium":"distribute.pc_search_result.none-task-cask-2~all~insert_cask~default-1-null.142^v93^chatsearchT3_2"}}] [.reference_item style="max-width: 33.333333333333336%"]
- *2* [【有问不答】混淆矩阵可视化(MATLAB)](https://blog.csdn.net/xsz591541060/article/details/118998758)[target="_blank" data-report-click={"spm":"1018.2226.3001.9630","extra":{"utm_source":"vip_chatgpt_common_search_pc_result","utm_medium":"distribute.pc_search_result.none-task-cask-2~all~insert_cask~default-1-null.142^v93^chatsearchT3_2"}}] [.reference_item style="max-width: 33.333333333333336%"]
- *3* [MATLAB实现多分类预测结果混淆矩阵(Confusion matrix)可视化](https://blog.csdn.net/qq_35667901/article/details/109016537)[target="_blank" data-report-click={"spm":"1018.2226.3001.9630","extra":{"utm_source":"vip_chatgpt_common_search_pc_result","utm_medium":"distribute.pc_search_result.none-task-cask-2~all~insert_cask~default-1-null.142^v93^chatsearchT3_2"}}] [.reference_item style="max-width: 33.333333333333336%"]
[ .reference_list ]
相关推荐
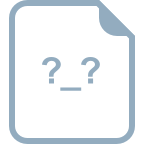
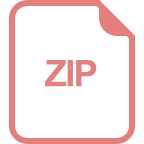












